Extracting Top-k Frequent and Diversified Patterns in Knowledge Graphs
IEEE TRANSACTIONS ON KNOWLEDGE AND DATA ENGINEERING(2024)
摘要
A knowledge graph contains many real-world facts that can be used to support various analytical tasks, e.g., exceptional fact discovery and the check of claims. In this work, we attempt to extract top -k frequent and diversified patterns from knowledge graph by well capturing user interest. Specifically, we first formalize the core-based top -k frequent pattern discovery problem, which finds the top -k frequent patterns that are extended from a core pattern specified by user query and have the highest frequency. In addition, to diversify the top -k frequent patterns, we define a distance function to measure the dissimilarity between two patterns, and return top -k patterns in which the pairwise diversity of any two resultant patterns exceeds a given threshold. As the search space of candidate patterns is exponential w.r.t. the number of nodes and edges in the knowledge graph, discovering frequent and diversified patterns is computationally challenging. To achieve high efficiency, we propose a suite of techniques, including (1) We devise a meta-index to avoid generating invalid candidate patterns; (2) We propose an upper bound of the frequency score (i.e., MNI) of the candidate pattern, which is used to prune unqualified candidates earlier and prioritize the enumeration order of patterns; (3) We design an advanced join-based approach to compute the MNI of candidate patterns efficiently; and (4) We develop a lower bound for distance function and incrementally compute the pair wise diversity among the patterns. Using real-world knowledge graphs, we experimentally verify the efficiency and effectiveness of our proposed techniques. We also demonstrate the utility of the extracted patterns by case studies.
更多查看译文
关键词
Knowledge discovery,graph pattern mining,data exploration
AI 理解论文
溯源树
样例
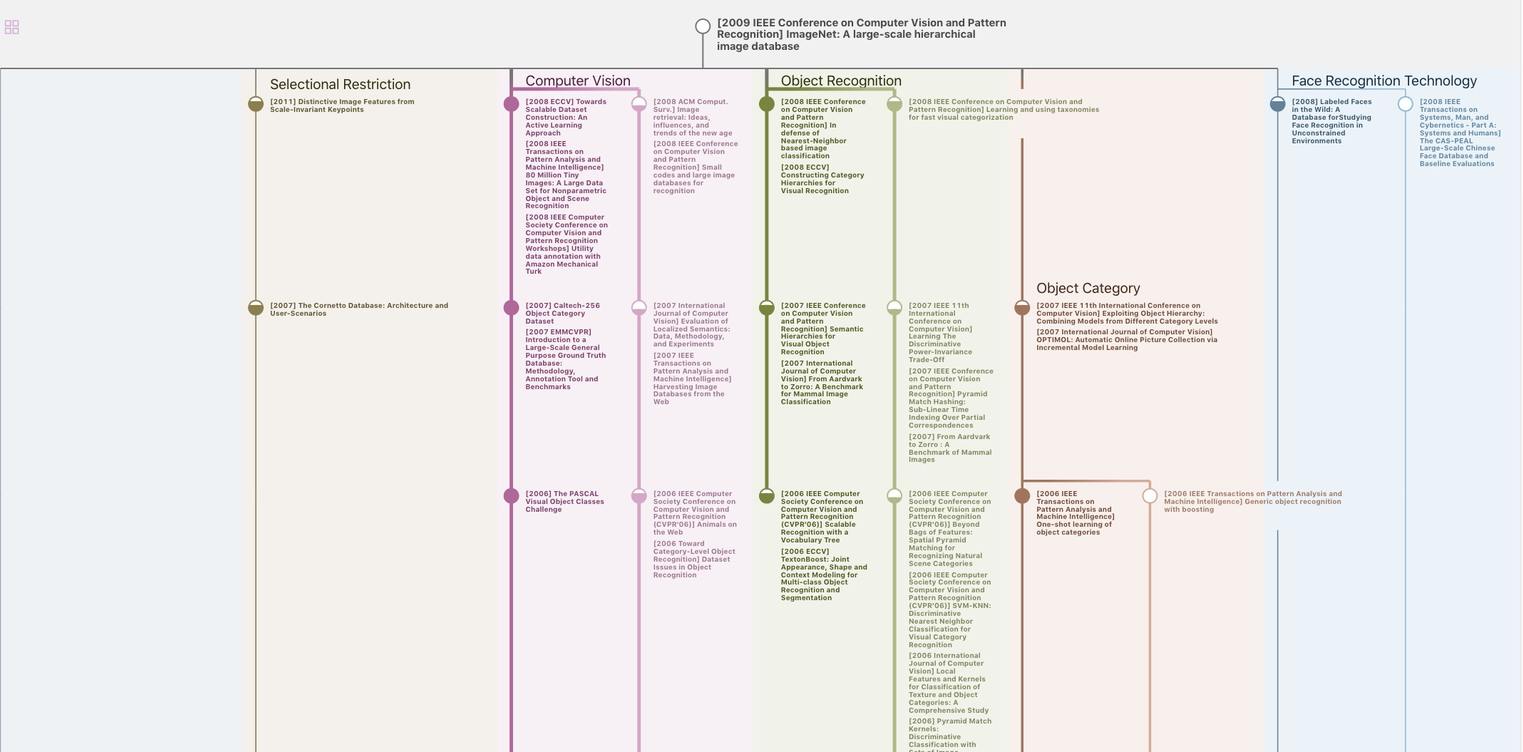
生成溯源树,研究论文发展脉络
Chat Paper
正在生成论文摘要