Exploiting Group-Level Behavior Pattern for Session-Based Recommendation
IEEE TRANSACTIONS ON KNOWLEDGE AND DATA ENGINEERING(2024)
摘要
Session-based recommendation (SBR) is a challenging task, which aims to predict users' future interests based on anonymous behavior sequences. Existing methods leverage powerful representation learning approaches to encode sessions into a low-dimensional space. However, despite such achievements, the existing studies focus on the instance-level session learning, while neglecting the group-level users' preferences (e.g., the common preferences of group users in repeat consumption). To this end, we propose a novel Repeat-aware Neural Mechanism for Session-based Recommendation (RNMSR). In RNMSR, we propose to learn the user preference from two levels: (i) instance-level, which employs GNNs on a similarity-based item-pairwise session graph to capture the users' preference in instance-level. (ii) group-level, which converts sessions into group-level behavior patterns to model the group-level users' preferences. In RNMSR, we combine instance-level and group-level user preference to model the repeat consumption of users, i.e., whether users take repeated consumption and which items are preferred by users. Extensive experiments are conducted on three real-world datasets, i.e., Diginetica, Yoochoose, and Nowplaying, demonstrating that the proposed method consistently achieves state-of-the-art performance in all the tests.
更多查看译文
关键词
Graph neural network,representation learning,session-based recommendation
AI 理解论文
溯源树
样例
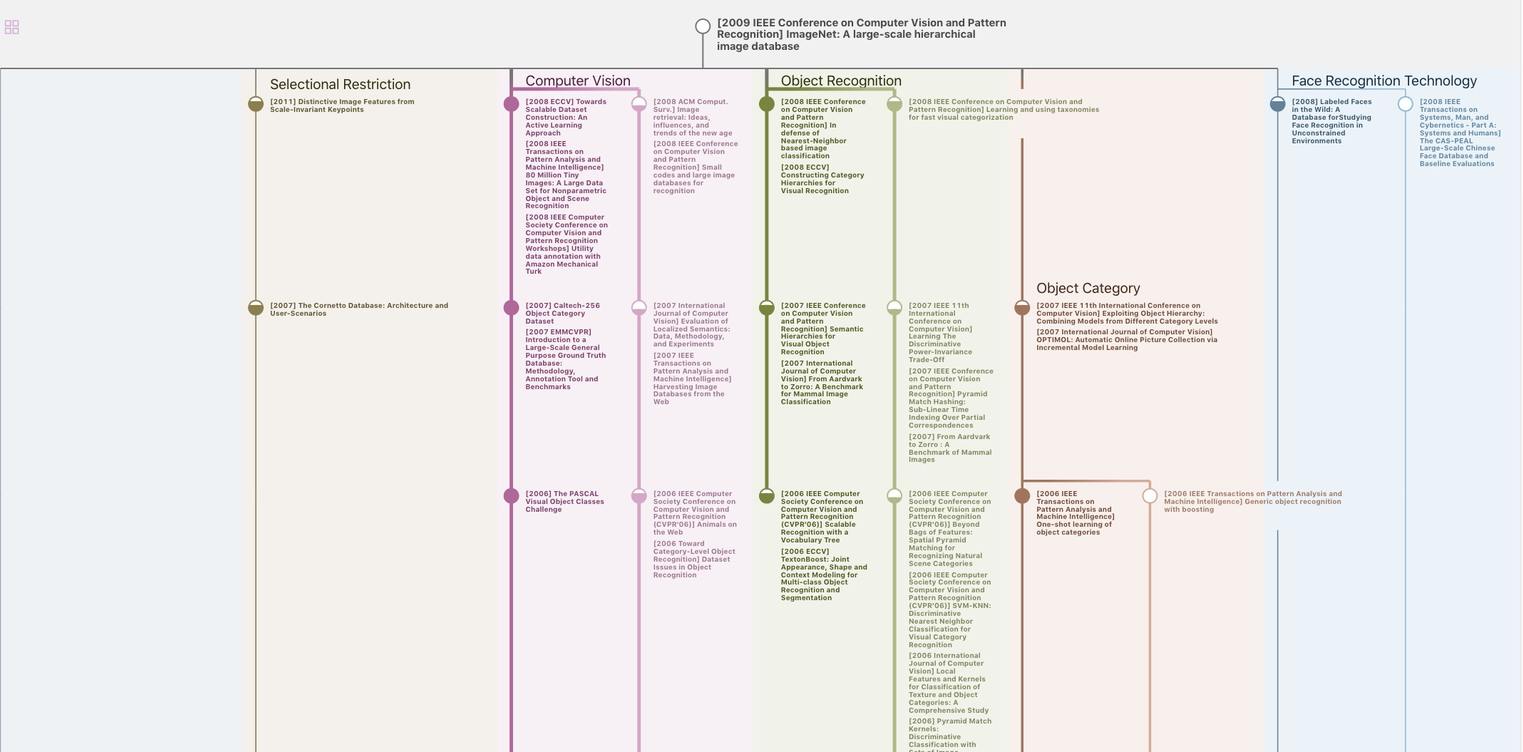
生成溯源树,研究论文发展脉络
Chat Paper
正在生成论文摘要