Noisy Evolutionary Optimization With Application to Grid-Based Persistent Monitoring
IEEE Transactions on Evolutionary Computation(2023)
摘要
This work concerns evolutionary approaches to black-box noisy optimization where the problem is accessed via noisy function evaluations. An evolution strategy (ES) algorithm is proposed, which uses a Gaussian distribution to guide the search and requires only the comparisons among solutions. The new method achieves the similar convergence rate as finite-difference based gradient methods on non-convex landscapes, while being adaptive in the sense that the convergence is ensured with any initial step-size. We further improve the method with a variance adaptation mechanisms that alleviates the need for hyper-parameter tunning and an asynchronous parallelization implementation that enables linear speedup. The persistent monitoring task is chosen as an application to investigate the effectiveness of the proposed method. The task is to schedule a team of agents to minimize the uncertainties of some targets in a changing environment defined on a grid map. We show in the single-agent case that the task can be cast into a sequence of pathfinding subproblems of which the sequential order can be modeled as a Markov decision procedure and solved by the proposed ES method. In the multi-agent case, we show that solving the single-agent subproblems using the ES method in a round-robin way can provide collision-free solutions. Numerical studies demonstrate the reduction in convergence time and the robustness against complicated environments relative to several existing evolutionary algorithms and zeroth-order gradient methods.
更多查看译文
关键词
Evolutionary algorithms,noisy optimization,persistent monitoring,derivative-free optimization
AI 理解论文
溯源树
样例
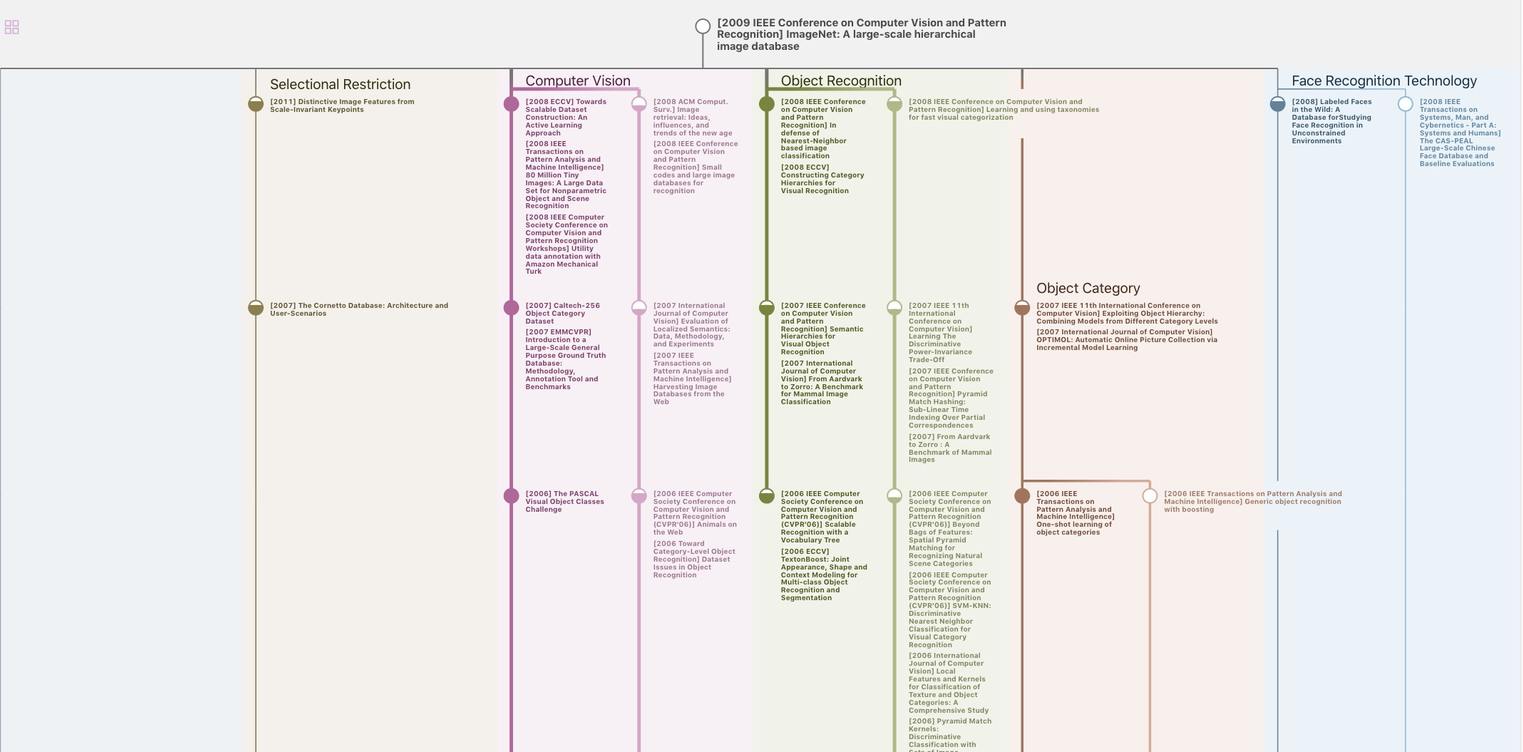
生成溯源树,研究论文发展脉络
Chat Paper
正在生成论文摘要