SecKNN: FSS-Based Secure Multi-Party KNN Classification Under General Distance Functions
IEEE TRANSACTIONS ON INFORMATION FORENSICS AND SECURITY(2024)
摘要
As a practical machine learning method, the K-nearest neighbors (KNN) classification has received widespread attention. The achievement of the KNN classification relies heavily on a large amount of labeled data. However, in the real world, data is often held by different data owners. How to realize efficient joint computing among multiple data owners under the premise of protecting data security and privacy is an urgent problem to be solved. In this paper, we construct a secure multi-party KNN classification scheme (SecKNN) based on function secret sharing (FSS) technology, which is a novel cryptographic primitive and can achieve cheap communication and computation costs for secure computation. Compared with the existing works, our scheme dramatically reduces computational overhead and runs roughly 50.8 times faster than the state-of-the-art approach. Furthermore, our scheme supports the secure KNN classification under general distance functions such as Euclidean distance, Manhattan distance, and Hamming distance. To implement our SecKNN scheme, we design two efficient FSS schemes for Hamming distance function, which implements secure two-party and multi-party Hamming distance computation in a single round. They can be considered as independent research results. Finally, we give formal security proofs for the proposed protocols and validate the effectiveness and efficiency of our protocols through experiments.
更多查看译文
关键词
Secure multi-party computation,function secret sharing,privacy-preserving k-nearest neighbors,Hamming distance,Manhattan distance
AI 理解论文
溯源树
样例
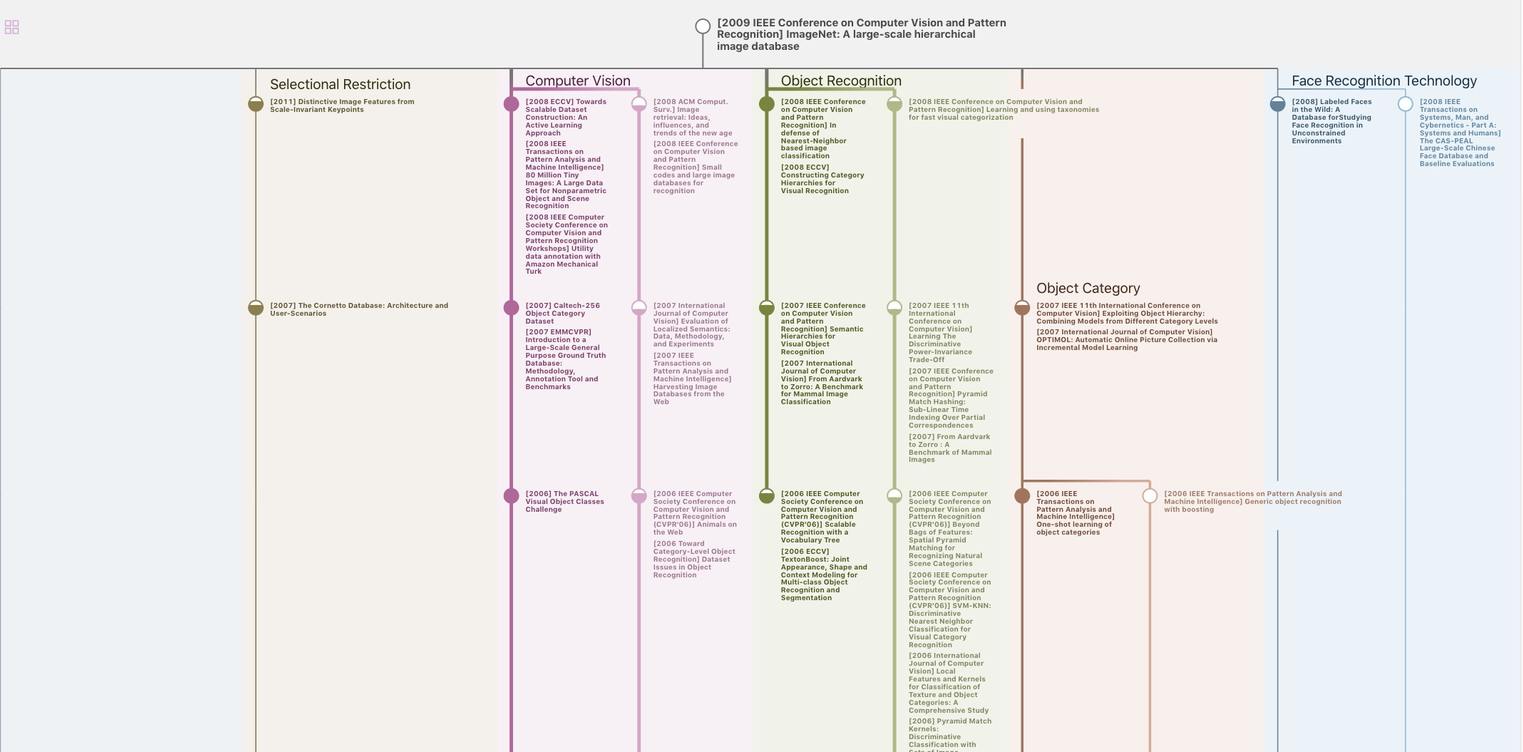
生成溯源树,研究论文发展脉络
Chat Paper
正在生成论文摘要