A Multi-Modal Vertical Federated Learning Framework Based on Homomorphic Encryption
IEEE TRANSACTIONS ON INFORMATION FORENSICS AND SECURITY(2024)
摘要
Federated learning has gained prominence as an effective solution for addressing data silos, enabling collaboration among multiple parties without sharing their data. However, existing federated learning algorithms often neglect the challenge posed by multi-modal data distribution. Moreover, previous pioneering work face limitations in encrypting the exponential and logarithmic operations of the objective function with multiple independent variables, and they rely on a third-party cooperator for encryption. To address these limitations, this paper introduces a universal multi-modal vertical federated learning framework. To tackle the data distribution challenge, we propose a two-step multi-modal transformer model that captures cross-domain semantic features effectively. For encryption, where traditional additively homomorphic encryption algorithms fall short by supporting only addition and multiplication, we employ bivariate Taylor series expansion to transform the objective function. Integrating these components, we present a comprehensive training and transmission protocol that eliminates the need for a third-party cooperator during the encryption process. Extensive experiments conducted on diverse video-text and image-text datasets validate the superior performance of our framework compared to state-of-the-art approaches, affirming its effectiveness in multi-modal vertical federated learning settings.
更多查看译文
关键词
Vertical federated learning,universal frame-work,homomorphic encryption,bivariate Taylor series expansion,multi-modal learning,cross-domain semantic feature extraction
AI 理解论文
溯源树
样例
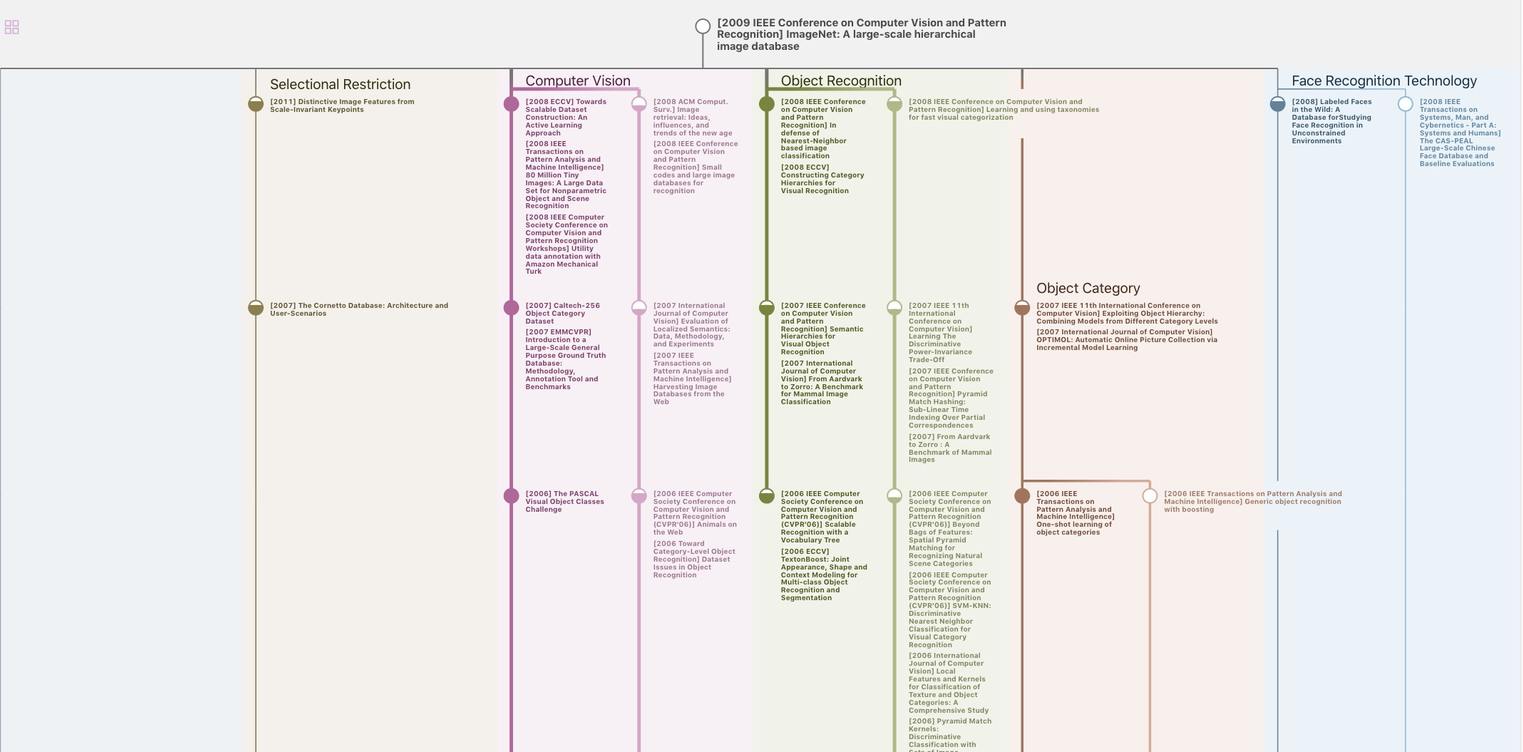
生成溯源树,研究论文发展脉络
Chat Paper
正在生成论文摘要