Spatial-Temporal Feature Network for Speech-Based Depression Recognition
IEEE TRANSACTIONS ON COGNITIVE AND DEVELOPMENTAL SYSTEMS(2024)
摘要
Depression is a serious mental disorder that has received increased attention from society. Due to the advantage of easy acquisition of speech, researchers have tried to propose various automatic depression recognition algorithms based on speech. Feature selection and algorithm design are the main difficulties in speech-based depression recognition. In our work, we propose the spatial-temporal feature network (STFN) for depression recognition, which can capture the long-term temporal dependence of audio sequences. First, to obtain a better feature representation for depression analysis, we develop a self-supervised learning framework, called vector quantized wav2vec transformer net (VQWTNet) to map speech features and phonemes with transfer learning. Second, the stacked gated residual blocks in the spatial feature extraction network are used in the model to integrate causal and dilated convolutions to capture multiscale contextual information by continuously expanding the receptive field. In addition, instead of LSTM, our method employs the hierarchical contrastive predictive coding (HCPC) loss in HCPCNet to capture the long-term temporal dependencies of speech, reducing the number of parameters while making the network easier to train. Finally, experimental results on DAIC-WOZ (Audio/Visual Emotion Challenge (AVEC) 2017) and E-DAIC (AVEC 2019) show that the proposed model significantly improves the accuracy of depression recognition. On both data sets, the performance of our method far exceeds the baseline and achieves competitive results compared to state-of-the-art methods.
更多查看译文
关键词
Convolutional neural network (CNN),deep learning,depression recognition,long short-term memory network,speech recognition
AI 理解论文
溯源树
样例
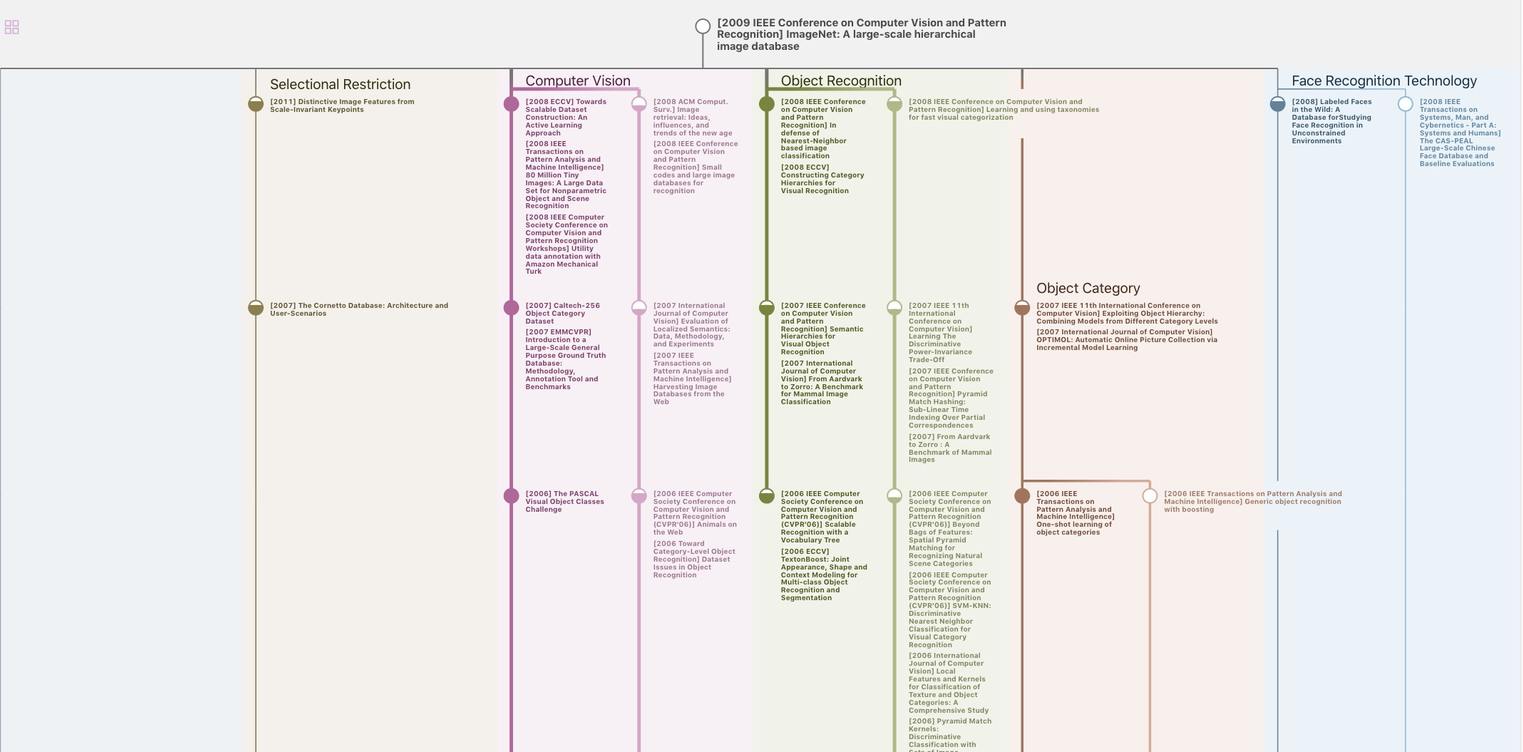
生成溯源树,研究论文发展脉络
Chat Paper
正在生成论文摘要