Jointly spatial-temporal representation learning for individual trajectories
CoRR(2023)
摘要
Individual trajectories, containing substantial information on
human-environment interactions across space and time, is a crucial input for
geospatial foundation models (GeoFMs). However, existing attempts, leveraging
trajectory data for various applications have overlooked the implicit
spatial-temporal dependency within trajectories and failed to encode and
represent it in a format friendly to deep learning, posing a challenge in
obtaining general-purpose trajectory representations. Therefore, this paper
proposes a spatial-temporal joint representation learning method (ST-GraphRL)
to formalize learnable spatial-temporal dependencies into trajectory
representations. The proposed ST-GraphRL consists of three compositions: (i) a
weighted directed spatial-temporal graph to explicitly construct mobility
interactions over both space and time dimensions; (ii) a two-stage jointly
encoder (i.e., decoupling and fusion) to learn entangled spatial-temporal
dependencies by independently decomposing and jointly aggregating space and
time information; (iii) a decoder guides ST-GraphRL to learn explicit mobility
regularities by simulating the spatial-temporal distributions of trajectories.
Tested on three real-world human mobility datasets, the proposed ST-GraphRL
outperformed all the baseline models in predicting movement spatial-temporal
distributions and preserving trajectory similarity with high spatial-temporal
correlations. We also explore how spatial-temporal features presented in latent
space, validating that ST-GraphRL understands spatial-temporal patterns. This
method is also transferable for general-purpose geospatial data representations
for broad downstream tasks, as well advancing GeoFMs developing.
更多查看译文
AI 理解论文
溯源树
样例
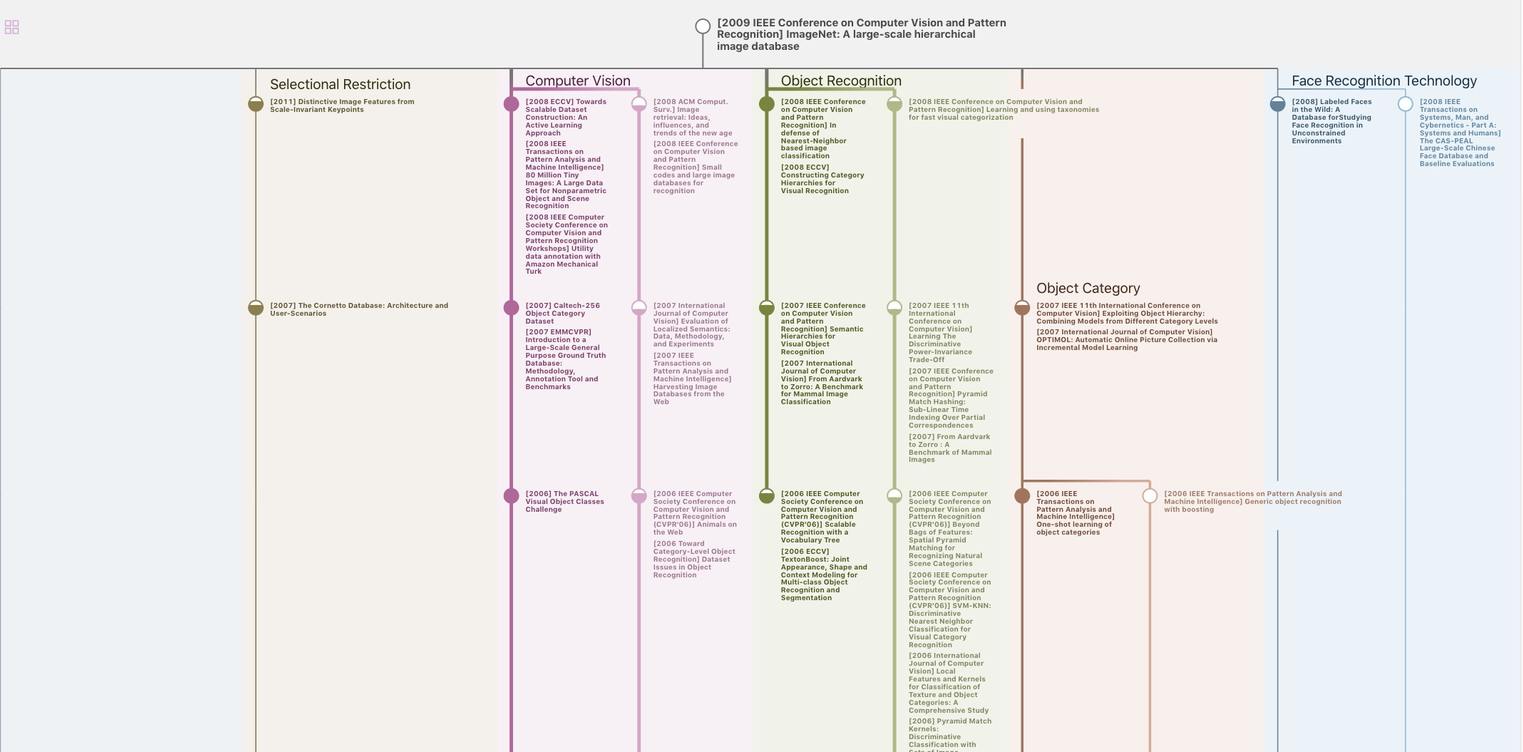
生成溯源树,研究论文发展脉络
Chat Paper
正在生成论文摘要