DiaPer: End-to-End Neural Diarization with Perceiver-Based Attractors
CoRR(2023)
摘要
Until recently, the field of speaker diarization was dominated by cascaded
systems. Due to their limitations, mainly regarding overlapped speech and
cumbersome pipelines, end-to-end models have gained great popularity lately.
One of the most successful models is end-to-end neural diarization with
encoder-decoder based attractors (EEND-EDA). In this work, we replace the EDA
module with a Perceiver-based one and show its advantages over EEND-EDA; namely
obtaining better performance on the largely studied Callhome dataset, finding
the quantity of speakers in a conversation more accurately, and running
inference on almost half of the time on long recordings. Furthermore, when
exhaustively compared with other methods, our model, DiaPer, reaches remarkable
performance with a very lightweight design. Besides, we perform comparisons
with other works and a cascaded baseline across more than ten public wide-band
datasets. Together with this publication, we release the code of DiaPer as well
as models trained on public and free data.
更多查看译文
AI 理解论文
溯源树
样例
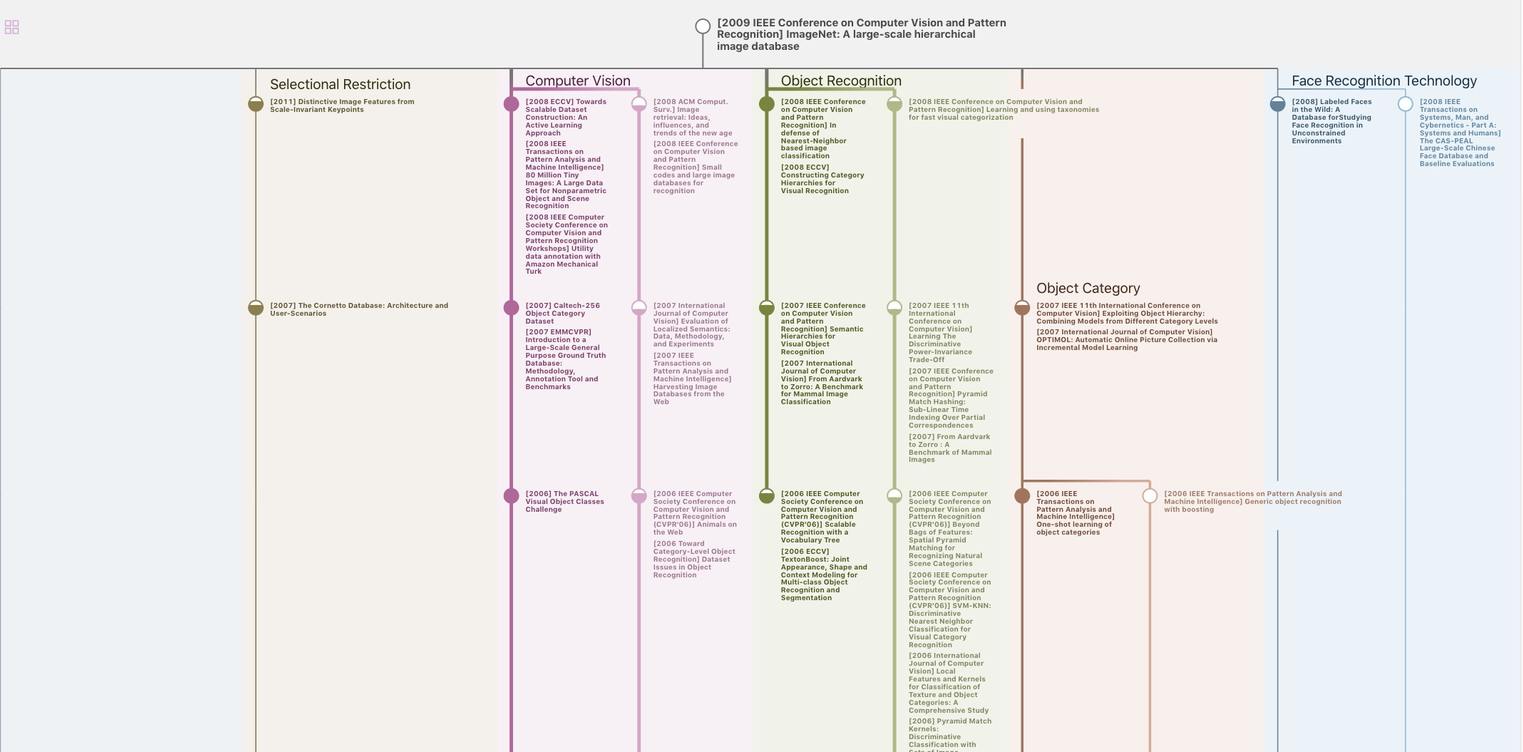
生成溯源树,研究论文发展脉络
Chat Paper
正在生成论文摘要