Local Spectral Similarity-Guided Sparse Unmixing of Hyperspectral Images With Spatial Graph Regularization
IEEE TRANSACTIONS ON GEOSCIENCE AND REMOTE SENSING(2023)
摘要
As the spectral library continues to expand, sparse hyperspectral unmixing methods have been developed to solve the mixing problem without the need for end-member extraction or generation. These methods leverage the intrinsic spectral and spatial information to enhance the accuracy of fractional abundance estimation. However, their effectiveness is limited by rigid spatial regularization and insufficient utilization of spectral-spatial information that hampers the improvement of unmixing performance. To overcome this limitation, we present a novel algorithm named local spectral similarity-guided sparse hyperspectral unmixing with spatial graph regularization (SGSU). In SGSU, we introduce a spatial graph regularization to enforce the interpixel correlation within spatial clusters and assign them to corresponding abundance vectors. To reduce the computational cost, we employ an adaptive superpixel-based spatial grouping strategy to segment the hyperspectral image, which translates the intrinsic geometry into constraints on abundance. Furthermore, we introduce a weighting factor with two components into the sparse unmixing framework. One component is based on the row sparsity of the estimated abundances, indicating the presence of active end-members; the other component is based on the similarity between neighboring pixels, which promotes piecewise smoothness of the estimated abundances. To obtain a more robust solution, we adopt a double-loop scheme based on the alternating direction method of multipliers (ADMM) algorithm to solve the SGSU model. Experimental results on both simulated and real hyperspectral datasets demonstrate that the proposed SGSU algorithm outperforms state-of-the-art sparse unmixing methods in terms of both accuracy of abundance estimation and end-member identification from spectral libraries. Our algorithm achieves superior unmixing results, which indicates its potential for practical applications in hyperspectral imaging.
更多查看译文
关键词
Hyperspectral imaging,Libraries,Sparse matrices,Estimation,Correlation,Collaboration,TV,Graph regularization,sparse hyperspectral unmixing,spatial information,spatially weighted unmixing,superpixel segmentation
AI 理解论文
溯源树
样例
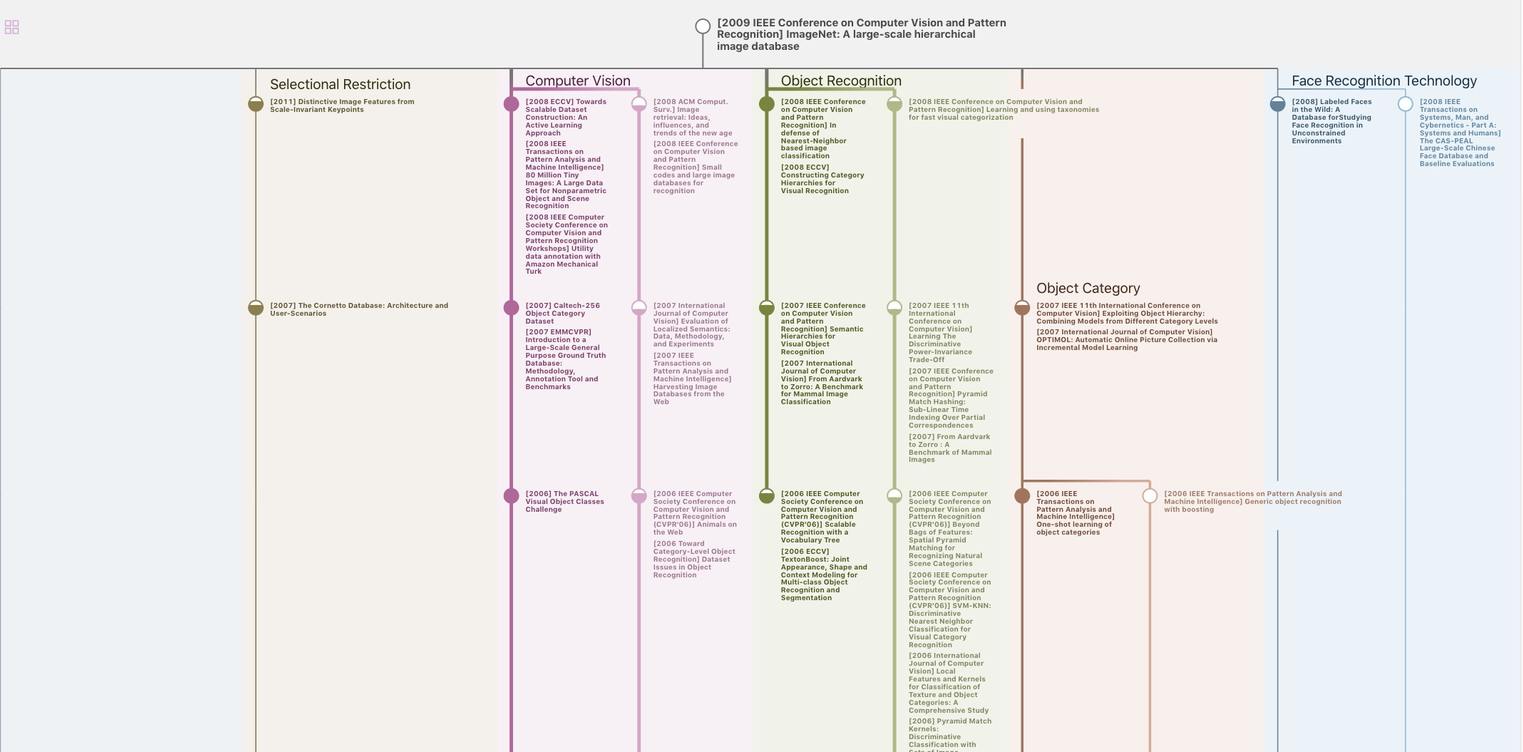
生成溯源树,研究论文发展脉络
Chat Paper
正在生成论文摘要