Development and Validation of a Machine Learning System to Identify Reflux Events in Esophageal 24-hour pH/Impedance Studies
Clinical and translational gastroenterology(2023)
摘要
INTRODUCTION: Esophageal 24-hour pH/impedance testing is routinely performed to diagnose gastroesophageal reflux disease. Interpretation of these studies is time-intensive for expert physicians and has high inter-reader variability. There are no commercially available machine learning tools to assist with automated identification of reflux events in these studies. METHODS: A machine learning system to identify reflux events in 24-hour pH/impedance studies was developed, which included an initial signal processing step and a machine learning model. Gold-standard reflux events were defined by a group of expert physicians. Performance metrics were computed to compare the machine learning system, current automated detection software (Reflux Reader v6.1), and an expert physician reader. RESULTS: The study cohort included 45 patients (20/5/20 patients in the training/validation/test sets, respectively). The mean age was 51 (standard deviation 14.5) years, 47% of patients were male, and 78% of studies were performed off proton-pump inhibitor. Comparing the machine learning system vs current automated software vs expert physician reader, area under the curve was 0.87 (95% confidence interval [CI] 0.85–0.89) vs 0.40 (95% CI 0.37–0.42) vs 0.83 (95% CI 0.81–0.86), respectively; sensitivity was 68.7% vs 61.1% vs 79.4%, respectively; and specificity was 80.8% vs 18.6% vs 87.3%, respectively. DISCUSSION: We trained and validated a novel machine learning system to successfully identify reflux events in 24-hour pH/impedance studies. Our model performance was superior to that of existing software and comparable to that of a human reader. Machine learning tools could significantly improve automated interpretation of pH/impedance studies.
更多查看译文
关键词
reflux events,esophageal,esophageal
AI 理解论文
溯源树
样例
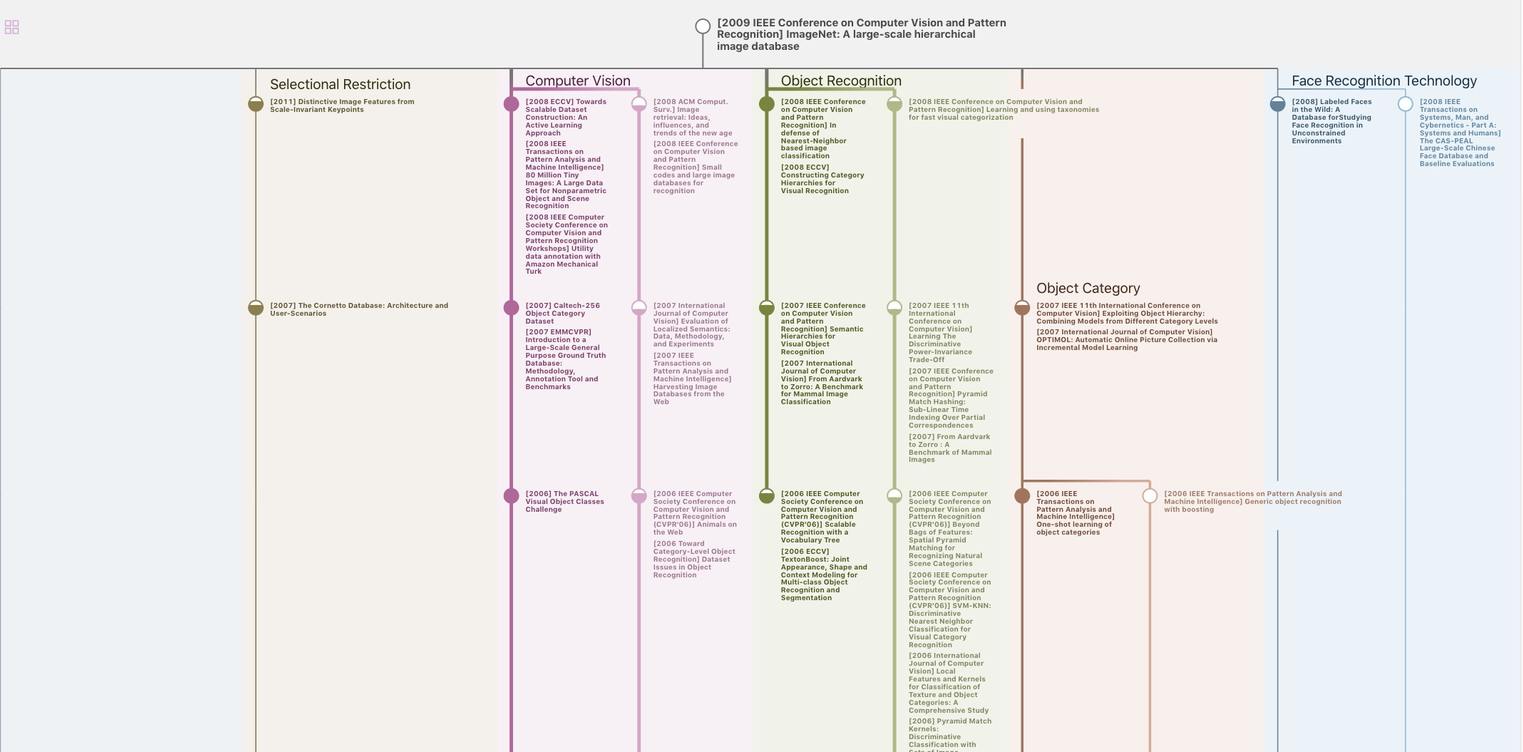
生成溯源树,研究论文发展脉络
Chat Paper
正在生成论文摘要