Joint Device Scheduling and Bandwidth Allocation for Federated Learning over Wireless Networks
IEEE Transactions on Wireless Communications(2023)
摘要
Federated Learning (FL) has been widely used to train shared machine learning models while addressing the privacy concerns. When deployed in wireless networks, bandwidth resources limitation is a key issue, thereby necessitating device scheduling and bandwidth allocation. It is challenging to carry out device scheduling due to the large combinatorial search space. Besides, the heterogeneous computing capabilities and uncertain channel states of wireless devices complicate the design of a bandwidth allocation method. In this paper, we propose a joint device scheduling and bandwidth allocation framework for implementing FL in wireless networks. Specifically, deep reinforcement learning (DRL) is employed to conduct device scheduling. To this end, the state space, action space, and reward function of DRL are carefully defined for a typical FL system. Long short-term memory (LSTM) is adopted as the DRL agent to analyze the sequential input data. Given the scheduled devices of each global iteration, the proposed bandwidth allocation method aims to minimize the weighted sum of the time delay and energy consumption. Numerical experiments on both independent and identically distributed (IID) and non-IID datasets demonstrate that the proposed framework enables FL to reach the desired accuracy with low time delay and energy consumption.
更多查看译文
关键词
Bandwidth Allocation,Device Scheduling,Federated Learning,Privacy,Reinforcement Learning
AI 理解论文
溯源树
样例
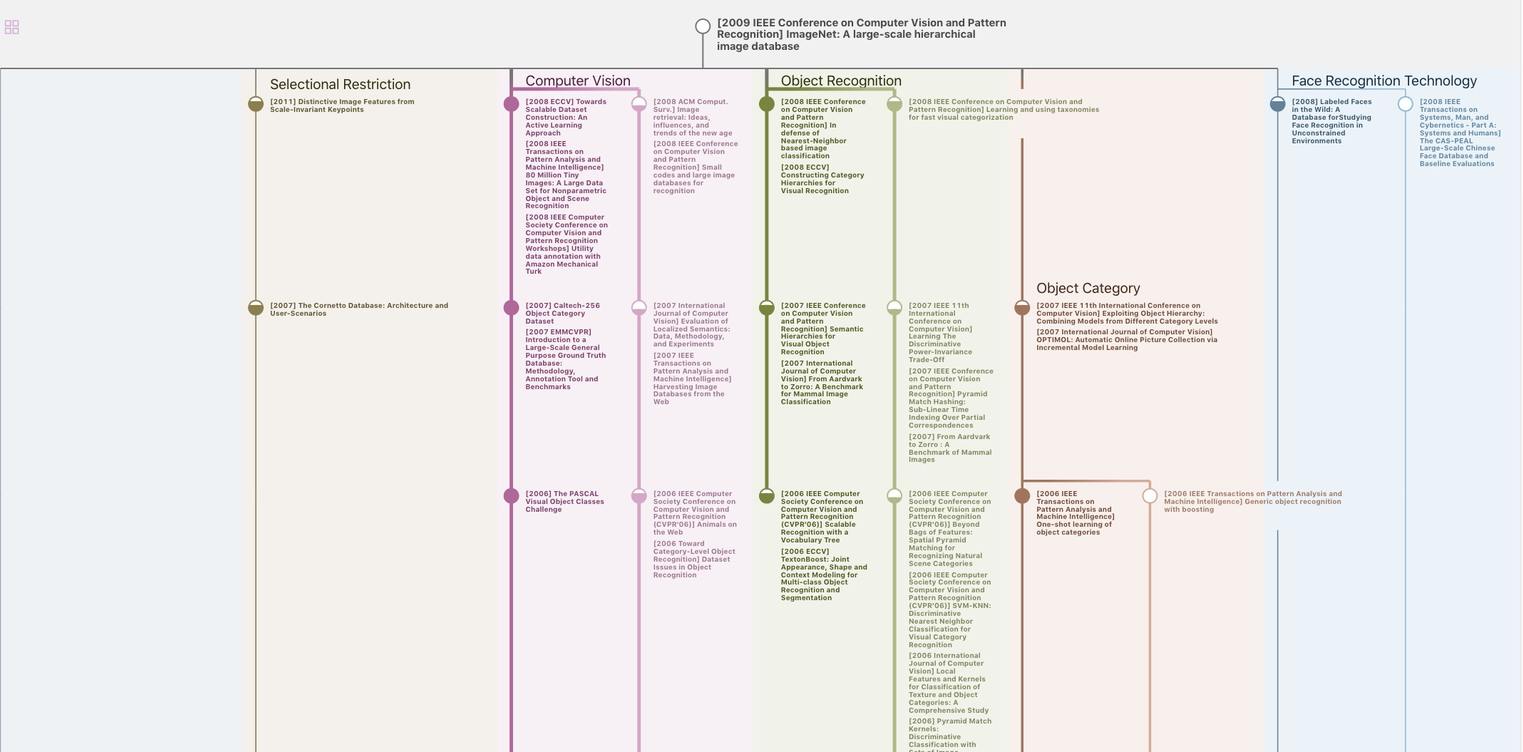
生成溯源树,研究论文发展脉络
Chat Paper
正在生成论文摘要