Big data analytics for relative humidity time series forecasting based on the LSTM network and ELM
IJAIN (International Journal of Advances in Intelligent Informatics)(2023)
摘要
Accurate and reliable relative humidity forecasting is important when evaluating the impacts of climate change on humans and ecosystems. However, the complex interactions among geophysical parameters are challenging and may result in inaccurate weather forecasting. This study combines long short-term memory (LSTM) and extreme learning machines (ELM) to create a hybrid model-based forecasting technique to predict relative humidity to improve the accuracy of forecasts. Detailed experiments with univariate and multivariate problems were conducted, and the results show that LSTM-ELM and ELM-LSTM have the lowest MAE and RMSE results compared to stand-alone LSTM and ELM for the univariate problem. In addition, LSTM-ELM and ELM-LSTM result in lower computation time than stand-alone LSTM. The experiment results demonstrate that the proposed hybrid models outperform the comparative methods in relative humidity forecasting. We employed the recursive feature elimination (RFE) method and showed that dewpoint temperature, temperature, and wind speed are the factors that most affect relative humidity. A higher dewpoint temperature indicates more air moisture, equating to high relative humidity. Humidity levels also rise as the temperature rises.
更多查看译文
关键词
big data analytics,relative humidity,time series forecasting,lstm,elm
AI 理解论文
溯源树
样例
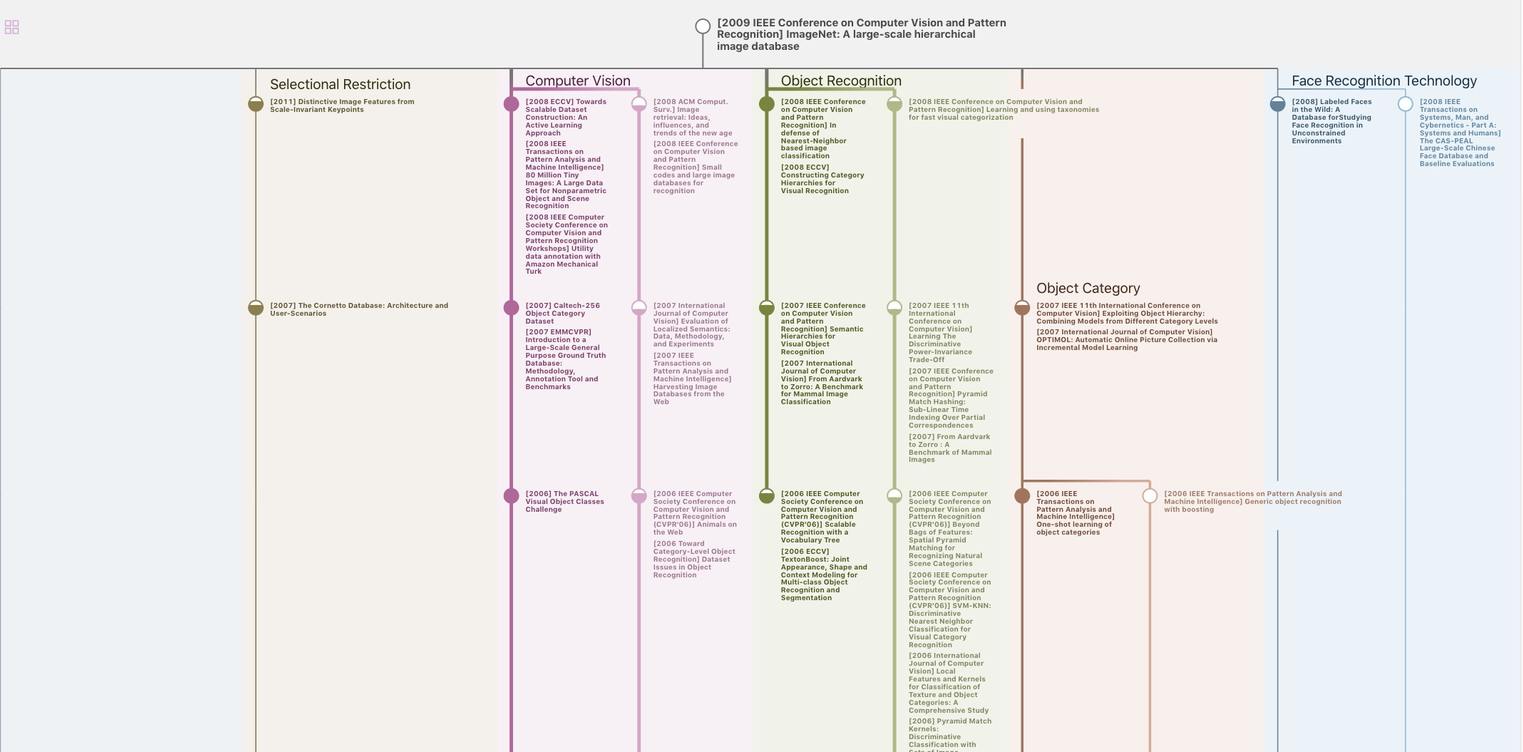
生成溯源树,研究论文发展脉络
Chat Paper
正在生成论文摘要