Federated Split Learning With Joint Personalization-Generalization for Inference-Stage Optimization in Wireless Edge Networks.
IEEE Trans. Mob. Comput.(2024)
摘要
The demand for intelligent services at the network edge has introduced several research challenges. One is the need for a machine learning architecture that achieves personalization (to individual clients) and generalization (to unseen data) properties concurrently across different applications. Another is the need for an inference strategy that can satisfy network resource and latency constraints during testing-time. Existing techniques in federated learning have encountered a steep trade-off between personalization and generalization, and have not explicitly considered the resource requirements during the inference-stage. In this paper, we propose SplitGP, a joint edge-AI training and inference strategy that simultaneously captures generalization/personalization for efficient inference across resource-constrained clients. The training process of SplitGP is based on federated split learning, with the key idea of optimizing the client-side model to have personalization capability tailored to its main task, while training the server-side model to have generalization capability for handling out-of-distribution tasks. During testing-time, each client selectively offloads inference tasks to the server based on the uncertainty threshold tunable based on network resource availability. Through formal convergence analysis and inference time analysis, we provide guidelines on the selection of key meta-parameters in SplitGP. Experimental results confirm the advantage of SplitGP over existing baselines.
更多查看译文
关键词
Federated learning,split learning,edge-AI,inference,personalization,wireless edge network
AI 理解论文
溯源树
样例
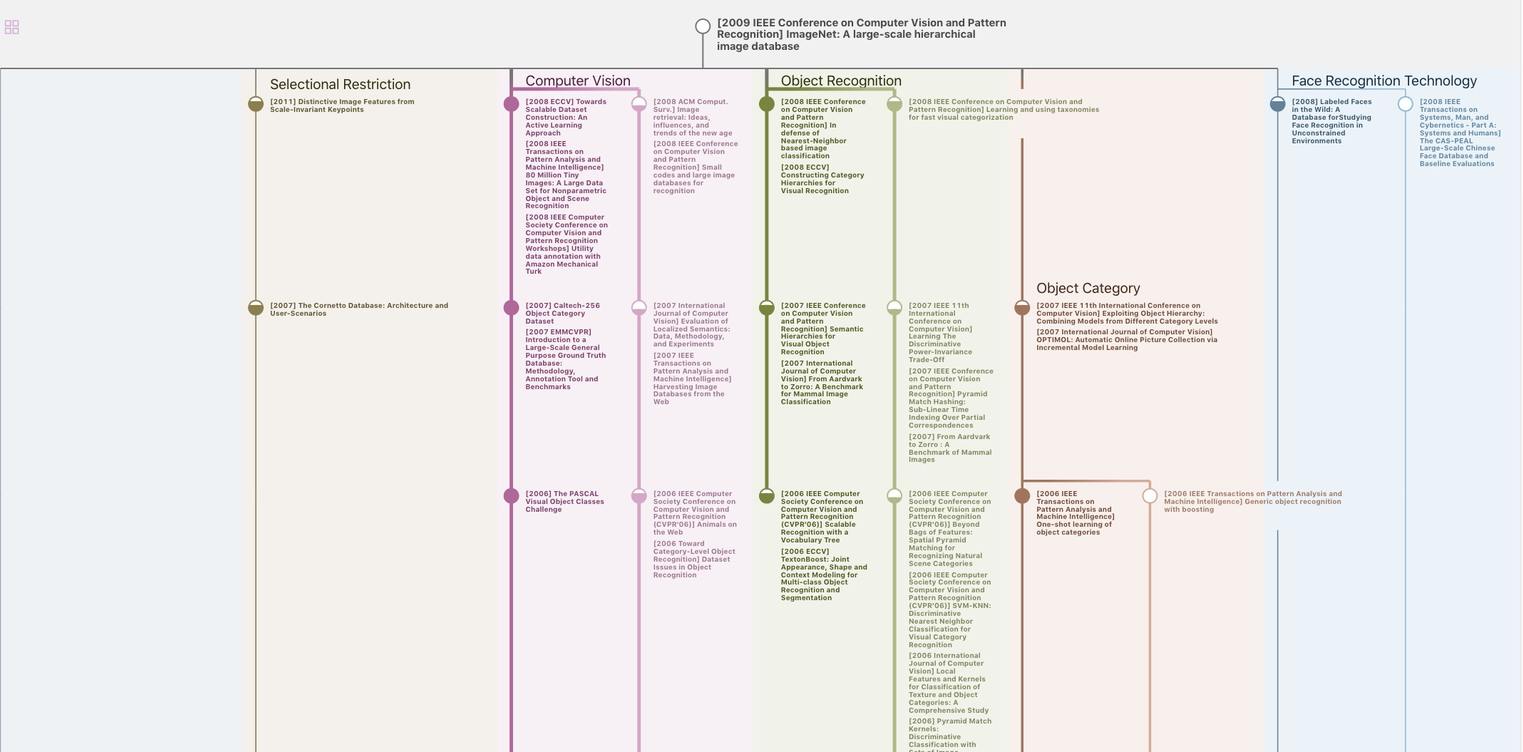
生成溯源树,研究论文发展脉络
Chat Paper
正在生成论文摘要