High Precision Traffic Flow Reconstruction via Hybrid Method
IEEE TRANSACTIONS ON INTELLIGENT TRANSPORTATION SYSTEMS(2023)
摘要
Traffic management and sustainable mobility are the central topics for intelligent transportation systems (ITS). By means of modern technologies, it is possible to collect real-time traffic flow data to extract useful information to monitor and control vehicular traffic. On the other hand, costs to obtain this piece of information are high. It requires either direct measures in the network road by installing large number of sensors (more precise data) or acquiring data from international providers supplying data coming from onboard units, mobile app, navigators, etc. In current paper, this problem has been addressed providing a solution granting traffic flow data in each road segment of the whole network by reconstructing the computation by means of data from few scattered traffic sensors in fixed positions of the road network. The proposed approach combines the solution of nonlinear Partial Differential Equations (PDEs) with machine learning for improving the state-of-the-art solutions of PDE. The result has been a higher precision with respect to PDE-based solutions, and a strongly reduced execution time. Several different machine learning models have been compared for such a purpose, demonstrating the general viability of the hybrid architecture proposed. The research result has been obtained in the framework of both the Sii-Mobility national project on transport systems, and MOST, the National Center on Sustainable mobility (both funded by the Italian Ministry of Research), by exploiting the Snap4City platform.
更多查看译文
关键词
Roads,Sensors,Data models,Computational modeling,Predictive models,Junctions,Pollution measurement,Traffic flow reconstruction,traffic flows,machine learning,hybrid architectures,machine learning PDE solution
AI 理解论文
溯源树
样例
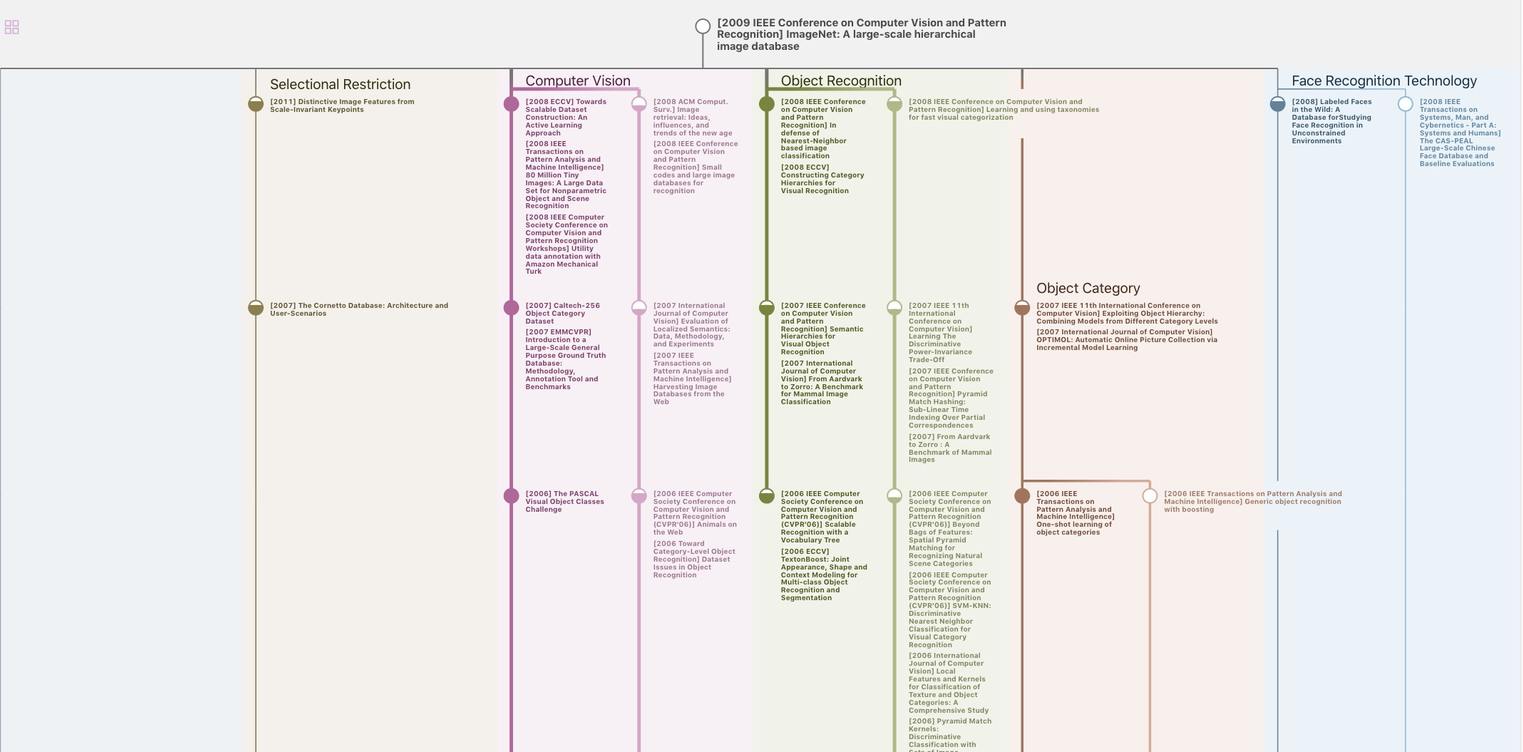
生成溯源树,研究论文发展脉络
Chat Paper
正在生成论文摘要