AdaDSR: Adaptive Configuration Optimization for Neural Enhanced Video Analytics Streaming
IEEE INTERNET OF THINGS JOURNAL(2024)
摘要
Neural-based super-resolution (SR) has achieved great success in enhancing image or video quality, creating new opportunities for building bandwidth-efficient and high-accuracy video analytics (VAs) systems. Intuitively, with the help of SR techniques, cameras only need to send downsampled low-quality frames to the server in a canonical edge-assisted VAs framework. The server-side SR model then upscales the quality of received frames for the subsequent VAs tasks, incurring thus substantially reduced bandwidth consumption. Nonetheless, as revealed by our measurement results on real-world video clips, higher delivery quality does not necessarily lead to higher analysis accuracy. This motivates us to study the content-adaptive downsampling and upscaling ratio selection problem for VAs streaming. We propose an SR-based VAs framework, named AdaDSR that can dynamically select the optimal downsampling and upscaling ratios so that the system utility can be maximized. AdaSDR is configured to balance the tradeoffs among accuracy, network cost, and computational cost. It further leverages the temporal consistency of videos to skip trivial decisions so that the camera's processing overhead can be reduced. Experiments on real-world video data sets demonstrate that AdaDSR can improve the average utility by 7.2%-18.4% when compared with state-of-the-art approaches under diverse video scenes.
更多查看译文
关键词
Configuration optimization,edge computing,super-resolution (SR),video analytics (VAs)
AI 理解论文
溯源树
样例
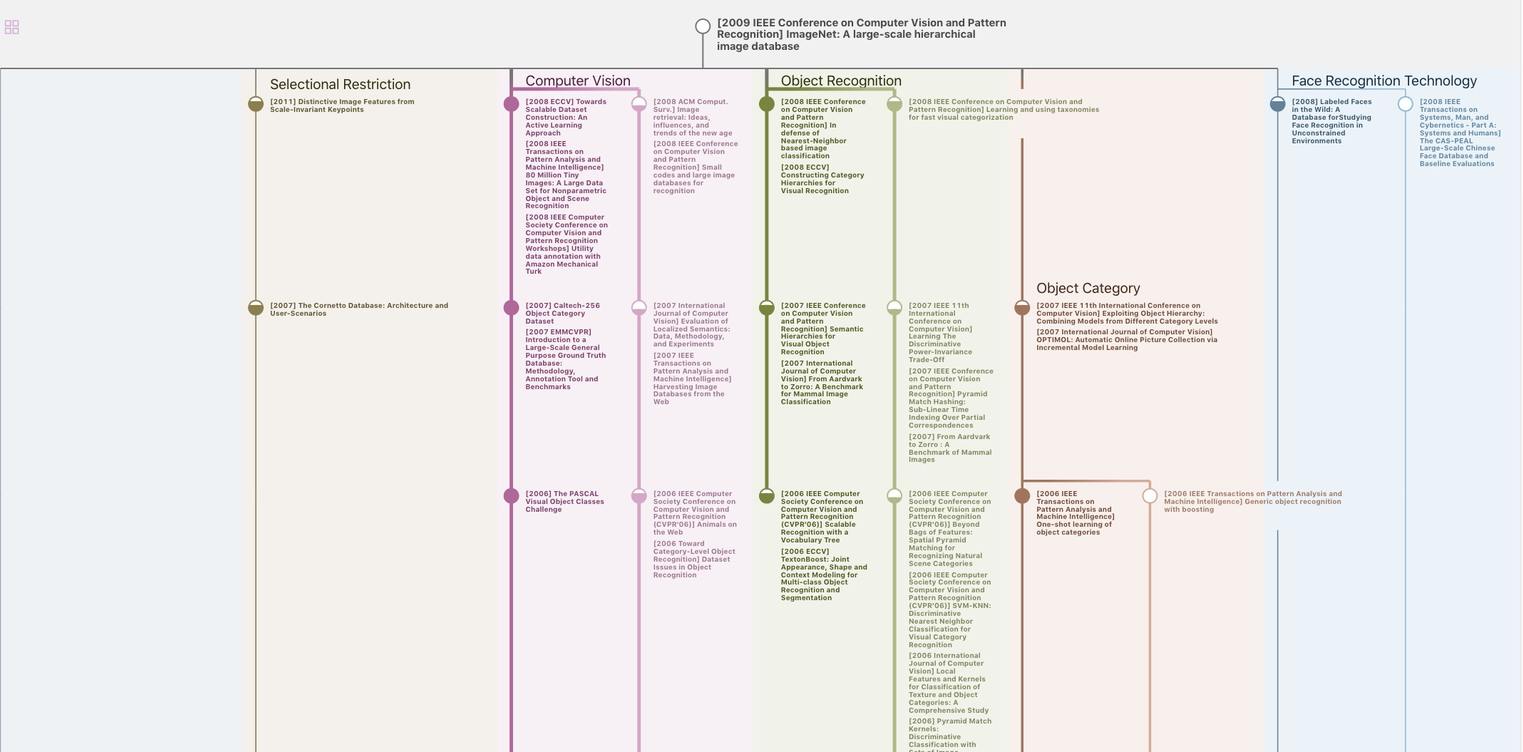
生成溯源树,研究论文发展脉络
Chat Paper
正在生成论文摘要