Linear Subspace Surrogate Modeling for Large-Scale Expensive Single/Multi-Objective Optimization
IEEE Transactions on Evolutionary Computation(2023)
摘要
Despite that the surrogate-assisted evolutionary algorithms have achieved great success in addressing expensive optimization problems, they still suffer from stiff challenges when the number of dimensions of problems becomes large. The primary reason lies in that it is very hard to build an acceptable surrogate model in the high-dimensional search space with small amounts of evaluated historical data in evolution. To tackle this issue, we suggest an effective surrogate modeling method for large-scale expensive optimization in this paper, where the models are built on a number of linear subspaces instead of the original search space. Specifically, a linear subspace is constructed by a pair of points/solutions which are generated based on the set of elite solutions. For each linear subspace, several historical solutions are first associated according to their distance to the linear subspace, and then a surrogate model is trained by the associated solutions and used to evaluate the offspring. To ensure the exploration and exploitation capacity of the proposed method, these linear subspaces and the surrogate models are updated after a few iterations. Experimental results on CEC’2010 and CEC’2013 single-objective optimization problems with up to 1500 decision variables show that the proposed algorithm is superior over six comparison algorithms. Moreover, we also extend the proposed algorithm to multi-objective optimization problems and verified its competitiveness on problems with up to 1500 decision variables.
更多查看译文
关键词
Large-scale problem,expensive optimization,multi-objective optimization,surrogate model
AI 理解论文
溯源树
样例
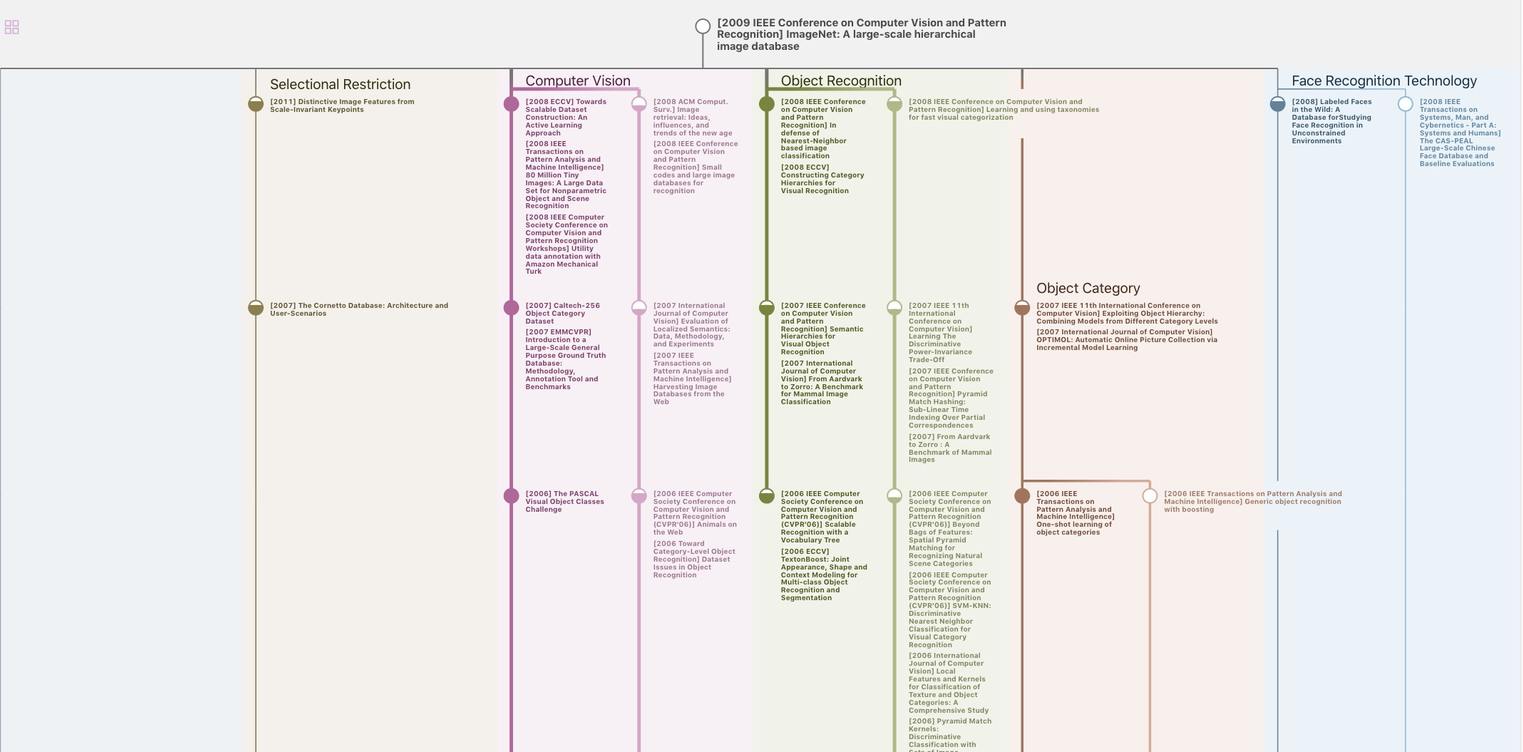
生成溯源树,研究论文发展脉络
Chat Paper
正在生成论文摘要