MeDM: Mediating Image Diffusion Models for Video-to-Video Translation with Temporal Correspondence Guidance
arXiv (Cornell University)(2023)
摘要
This study introduces an efficient and effective method, MeDM, that utilizes pre-trained image Diffusion Models for video-to-video translation with consistent temporal flow. The proposed framework can render videos from scene position information, such as a normal G-buffer, or perform text-guided editing on videos captured in real-world scenarios. We employ explicit optical flows to construct a practical coding that enforces physical constraints on generated frames and mediates independent frame-wise scores. By leveraging this coding, maintaining temporal consistency in the generated videos can be framed as an optimization problem with a closed-form solution. To ensure compatibility with Stable Diffusion, we also suggest a workaround for modifying observed-space scores in latent-space Diffusion Models. Notably, MeDM does not require fine-tuning or test-time optimization of the Diffusion Models. Through extensive qualitative, quantitative, and subjective experiments on various benchmarks, the study demonstrates the effectiveness and superiority of the proposed approach. Project page can be found at https://medm2023.github.io
更多查看译文
关键词
image diffusion models,temporal correspondence guidance,video-to-video
AI 理解论文
溯源树
样例
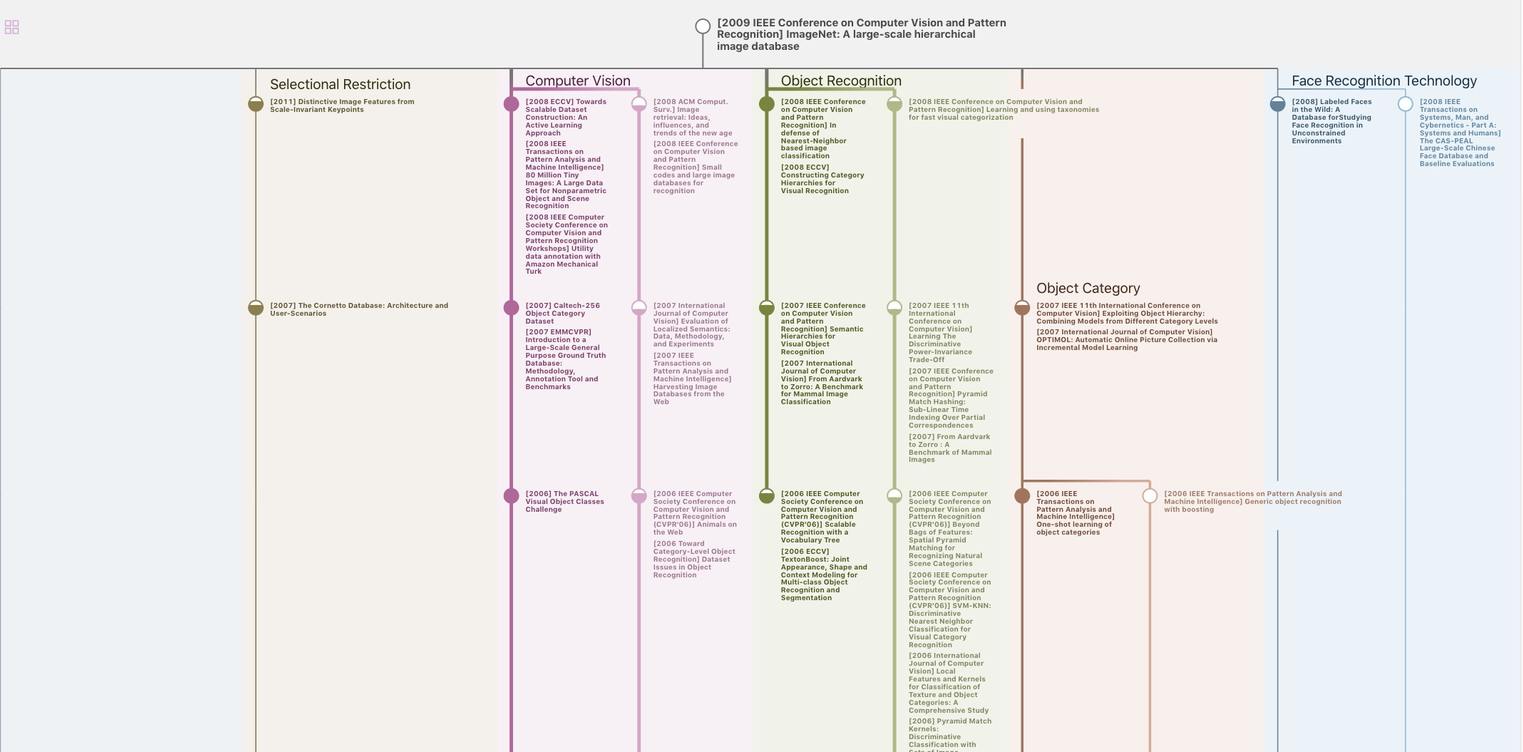
生成溯源树,研究论文发展脉络
Chat Paper
正在生成论文摘要