DecAge: Decentralized Flow Scheduling for Industrial 5G and TSN Integrated Networks
IEEE TRANSACTIONS ON NETWORK SCIENCE AND ENGINEERING(2024)
摘要
The integration of fifth-generation (5G) and time-sensitive networking (TSN) is a key technology to promote the wireless upgrade of manufacturing industry, and some preliminary enhancements on 5G with TSN-integration have been introduced to empower industrial internet ubiquitous connectivity and real-time forwarding. However, at present, the 5G system is only served as a logical bridge with 5G-specific procedures hidden from TSN networks, and thus the deep integration of 5G and TSN is still an open issue. This article focuses on the co-design problem of flow scheduling in 5G and TSN, where 5G grant-free configured-grants (CGs) and TSN cyclic queuing and forwarding mechanisms are adopted. Firstly, we innovatively propose to achieve seamless 5G+TSN transmission by intelligently selecting CGs in 5G, where the starting time offsets of different CGs will influence the flow injection time in the downstream TSN network to mitigate the queue overflow issue. Then, an age-of-information (age) aware decentralized and deterministic scheduling (DecAge) scheme based on actor-critic reinforcement learning is proposed. DecAge guides time-sensitive flow hosts to autonomously make packet sampling-transmission decisions so that timeliness-based age constraints can be respected via collision-free 5G+TSN transmission. DecAge contains multiple local actors learning hierarchically-structured scheduling policies for flow hosts and a central graph attention network-based critic to estimate these policies. Through simulation, DecAge shows the robustness to environmental non-stationarity by elaborately coordinating distributed learning and yields improved performance over non-hierarchical agents on sample-efficient learning.
更多查看译文
关键词
5G mobile communication,Job shop scheduling,Scheduling,Switches,NOMA,Reinforcement learning,Planning,5G+TSN flow scheduling,grant-free CGs,cyclic queuing and forwarding,multi-agent reinforcement learning
AI 理解论文
溯源树
样例
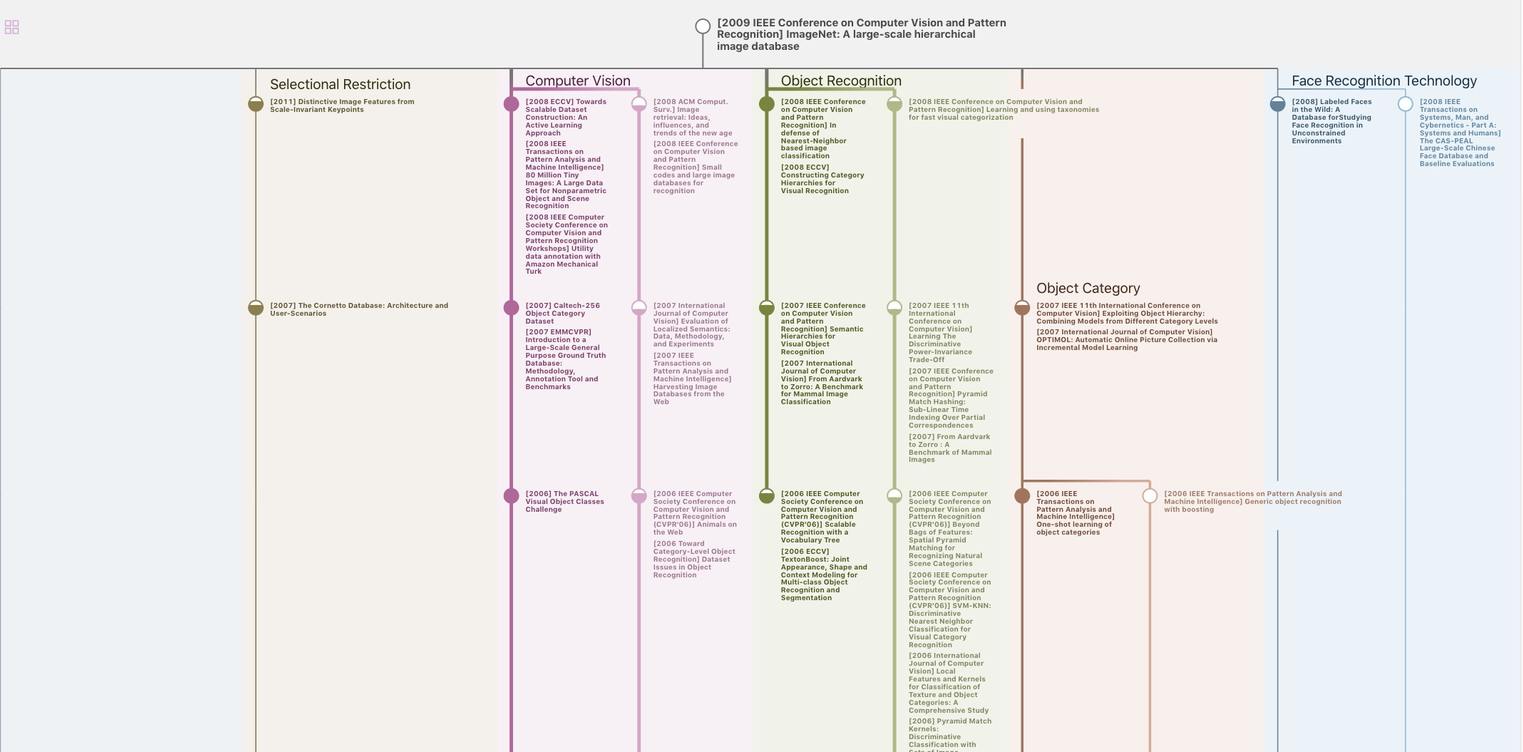
生成溯源树,研究论文发展脉络
Chat Paper
正在生成论文摘要