Edge TMS: Optimized Real-Time Temperature Monitoring Systems Deployed on Edge AI Devices
IEEE INTERNET OF THINGS JOURNAL(2024)
摘要
The temperature monitoring system (TMS) aims to reduce the infection spread and outbreak of COVID-19 through early detection. Conventional and currently deployed TMS have high implementation cost and require a substantial amount of space. Also, the performance often depends on the accuracy of the thermal camera. To address this, we propose Edge TMS wherein a multitask cascaded convolutional neural networks (MTCNNs)based TMS is deployed on an edge AI device. To overcome the resource constraints of edge AI devices, an optimization method is applied to compress MTCNN up to 100x. The compressed MTCNN is deployed on the local PC, Jetson Xavier, Jetson TX2, and Jetson Nano which yields pruning-per-reduction ratio (PPRR) values of 1.21, 1.63, 1.99, and 2.10, respectively. We proposed the PPRR metric to measure the performance of the compressed model. Low PPRR values indicate an improvement in the hardware performance and computational efficiency of the optimized model. The optimized model deployed in all the Jetson series achieved an average percent power reduced (%R) of 53.18% with a percent difference of 35.9% from the results of the local PC.
更多查看译文
关键词
Deep learning (DL),edge AI devices,image processing and computer vision,network architecture optimization,temperature monitoring system (TMS)
AI 理解论文
溯源树
样例
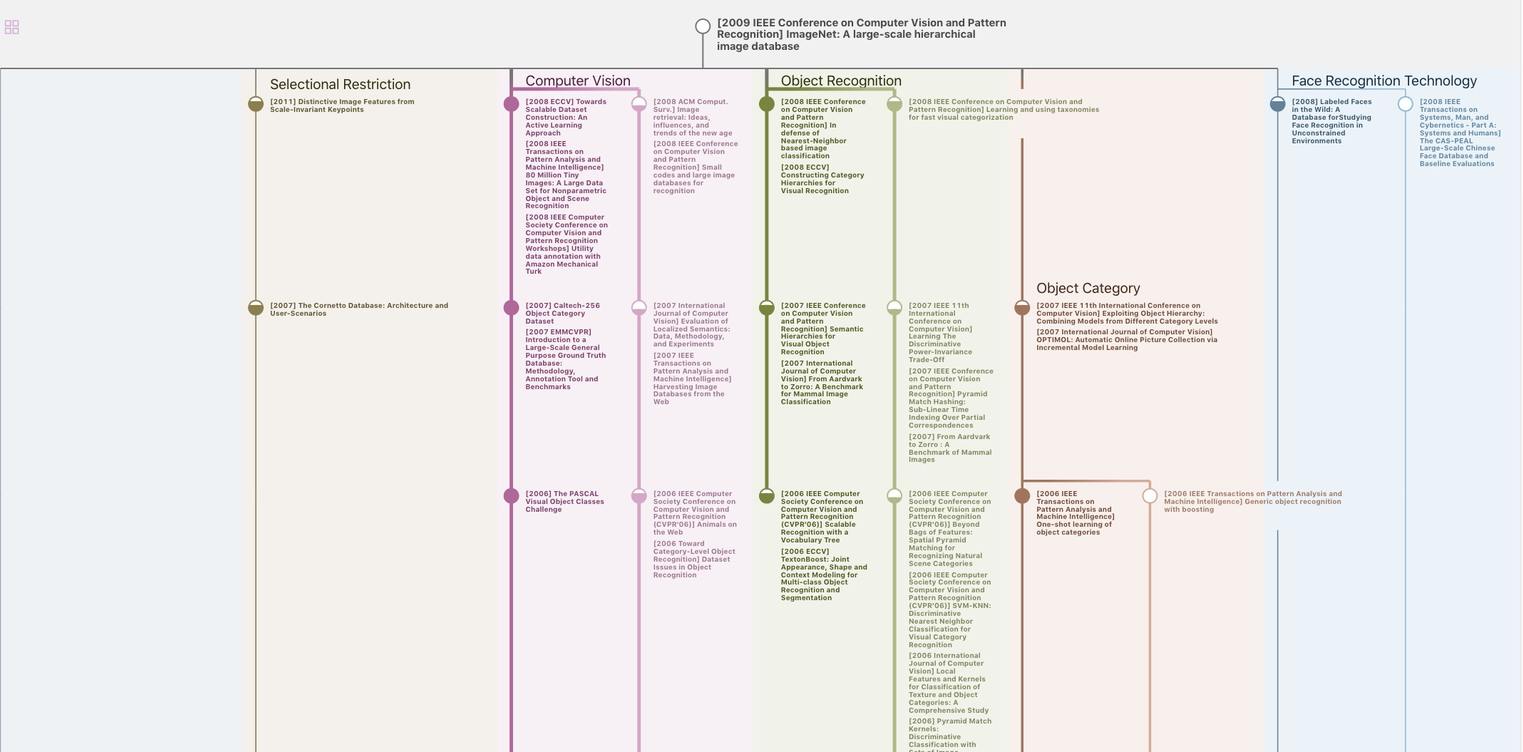
生成溯源树,研究论文发展脉络
Chat Paper
正在生成论文摘要