O-187 The combination of machine learning and pharmacogenetics for the prediction of sub-optimal ovarian response
Human Reproduction(2023)
摘要
Abstract Study question Can an artificial intelligence (AI)-based model predict the risk of suboptimal ovarian response using patient genetic data? Summary answer AI-based predictive models can identify relevant predicting factors for suboptimal ovarian response, including several genetic variants. What is known already A substantial proportion of women classified as “normo-responders” may not reach the optimal range of oocytes retrieved after COS. Polyzos and Sunkara (2015) defined a new category of women (15-36%) with a worse prognosis and a “suboptimal” level of ovarian response in which the number of oocytes retrieved ranges between 4 and 9 oocytes. The explanation for this behavior has been suggested to be genetic and indeed variants in FSH receptor and LH subunit-B have been identified that predispose to lower ovarian sensitivity to stimulation. Therefore, these women might require higher doses of gonadotropins or longer stimulations. Study design, size, duration This observational study included a retrospective analysis of 1370 ovarian stimulations from egg donors performed between March 2018 and April 2022. The oocyte donor candidates were selected according to our clinic donation program requirements and ASRM and ESHRE guidelines for oocyte donation. They were stimulated following a progesterone-primed ovarian stimulation protocol. All donors started stimulation with 150-300 IU/day of FSH according to AFC and BMI. A GnRH agonist was used for final oocyte maturation. Participants/materials, setting, methods Oocyte donors were healthy women between 18 and 35 years old (n = 504). In order to establish the predictive machine learning models, patient characteristics and controlled ovarian stimulation data were recorded in a data frame. In addition, donors were genotyped for 31 variants corresponding to 16 genes associated with ovarian response. The association between the different variables and risk of suboptimal ovarian response was analysed using SPSS (v23.0) and R (v. 4.2.0) statistical software. Main results and the role of chance The oocyte donors had a mean age of 25.4±4.0 and a high ovarian reserve (AFC: 18.4±7). The mean number of oocytes retrieved after ovarian stimulation was 16.3±7.5, of which 13.1±6.4 were mature. Despite being young women with good ovarian reserve, 16.4% of the stimulations were suboptimal (4-9 oocytes retrieved). Classical statistical method (binary logistic regression) and 5 different supervised classification machine learning algorithms (multi-layer perceptron, support vector-machines, k-nearst neighbors, random forest and eXtreme Gradient Boosting (XGBoost)) were used to establish a prediction model for suboptimal ovarian response. The model with the highest AUC value was the XGBoost (0.876). The accuracy, sensitivity and specificity were 0.798, 0.809 and 0.787 respectively. The variables that had the greatest predictive power in the best machine learning algorithm (XGBoost) were: age, BMI, AFC and total gonadotrophin dose. In addition, four genetic variants were identified that modified the risk of a suboptimal ovarian response: -ESR2 (oestrogen receptor; c.*39G>A). -LHB (LH beta subunit; c.82T>C/p.Trp28Arg). -SOD2 (superoxide dismutase; c.47T>C/p.Val16Ala). A mitochondrial enzyme that catalyses the detoxification and protection against redox damage that can occur in COS. -and TP53 (tumour suppressor protein; c.215C>T/p.Pro72Leu) that exerts a protective effect on DNA damage in folliculogenesis. Limitations, reasons for caution Our investigation was performed with retrospectively collected data, and hence it will be of importance to collect data prospectively to confirm that the new identified variants are associated with suboptimal ovarian response also in the IVF population. Wider implications of the findings The combination of AI and pharmacogenetics has led to the identification of genetic variants that might predispose to suboptimal ovarian response. Women who carry these variants (erroneously considered as normo-responders) could be candidates for personalization of their ovarian stimulation treatment with higher doses of gonadotrophins and/or longer stimulations. Trial registration number Not applicable
更多查看译文
关键词
pharmacogenetics,machine learning,sub-optimal
AI 理解论文
溯源树
样例
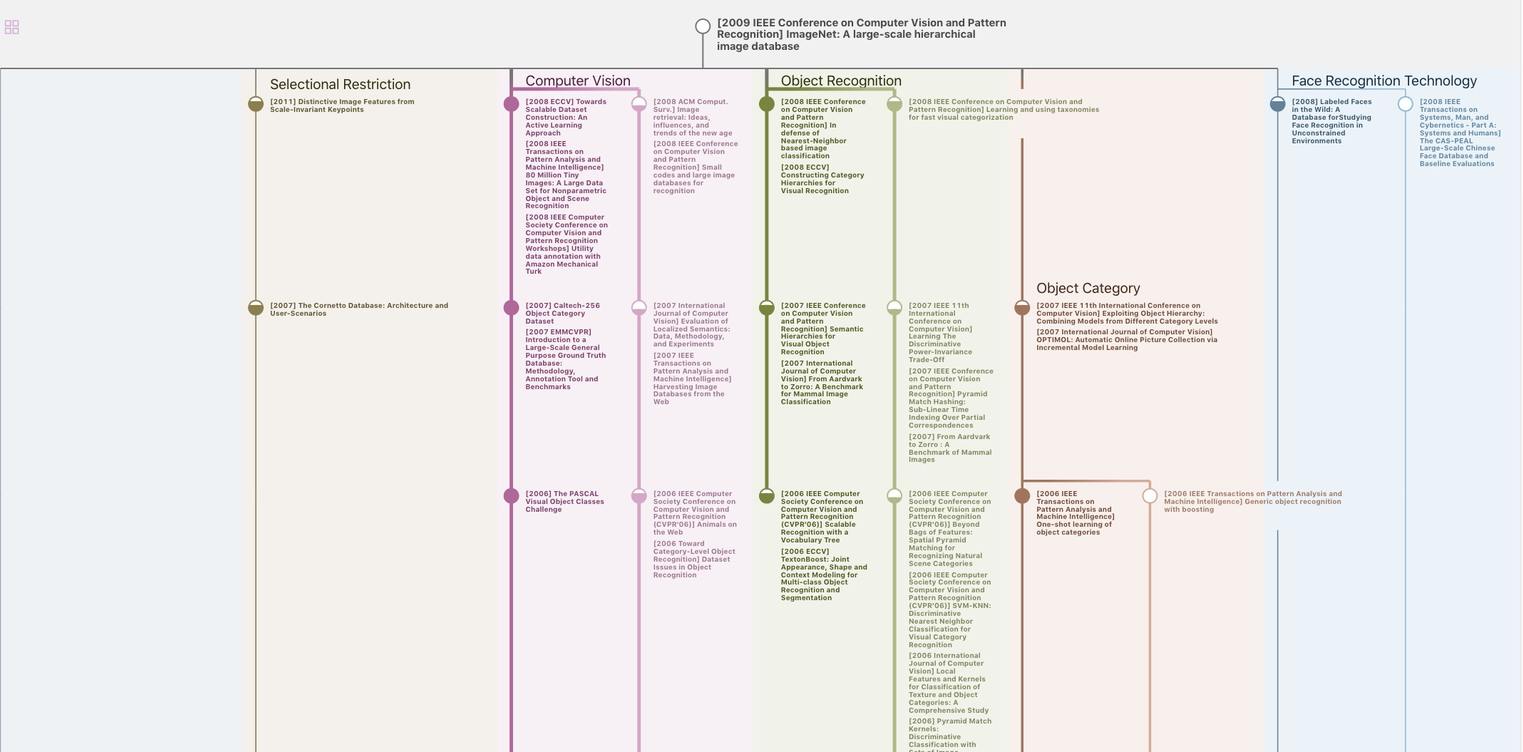
生成溯源树,研究论文发展脉络
Chat Paper
正在生成论文摘要