Learned spatiotemporal correlation priors for CEST image denoising using incorporated global‐spectral convolution neural network
Magnetic Resonance in Medicine(2023)
摘要
Purpose To develop a deep learning‐based method, dubbed Denoising CEST Network (DECENT), to fully exploit the spatiotemporal correlation prior to CEST image denoising. Methods DECENT is composed of two parallel pathways with different convolution kernel sizes aiming to extract the global and spectral features embedded in CEST images. Each pathway consists of a modified U‐Net with residual Encoder‐Decoder network and 3D convolution. Fusion pathway with 1 × 1 × 1 convolution kernel is utilized to concatenate two parallel pathways, and the output of DECENT is noise‐reduced CEST images. The performance of DECENT was validated in numerical simulations, egg white phantom experiments, and ischemic mouse brain and human skeletal muscle experiments in comparison with existing state‐of‐the‐art denoising methods. Results Rician noise was added to CEST images to mimic a low SNR situation for numerical simulation, egg white phantom experiment, and mouse brain experiments, while human skeletal muscle experiments were of inherently low SNR. From the denoising results evaluated by peak SNR (PSNR) and structural similarity index (SSIM), the proposed deep learning‐based denoising method (DECENT) can achieve better performance compared to existing CEST denoising methods such as NLmCED, MLSVD, and BM4D, avoiding complicated parameter tuning or time‐consuming iterative processes. Conclusions DECENT can well exploit the prior spatiotemporal correlation knowledge of CEST images and restore the noise‐free images from their noisy observations, outperforming state‐of‐the‐art denoising methods.
更多查看译文
关键词
spatiotemporal correlation priors
AI 理解论文
溯源树
样例
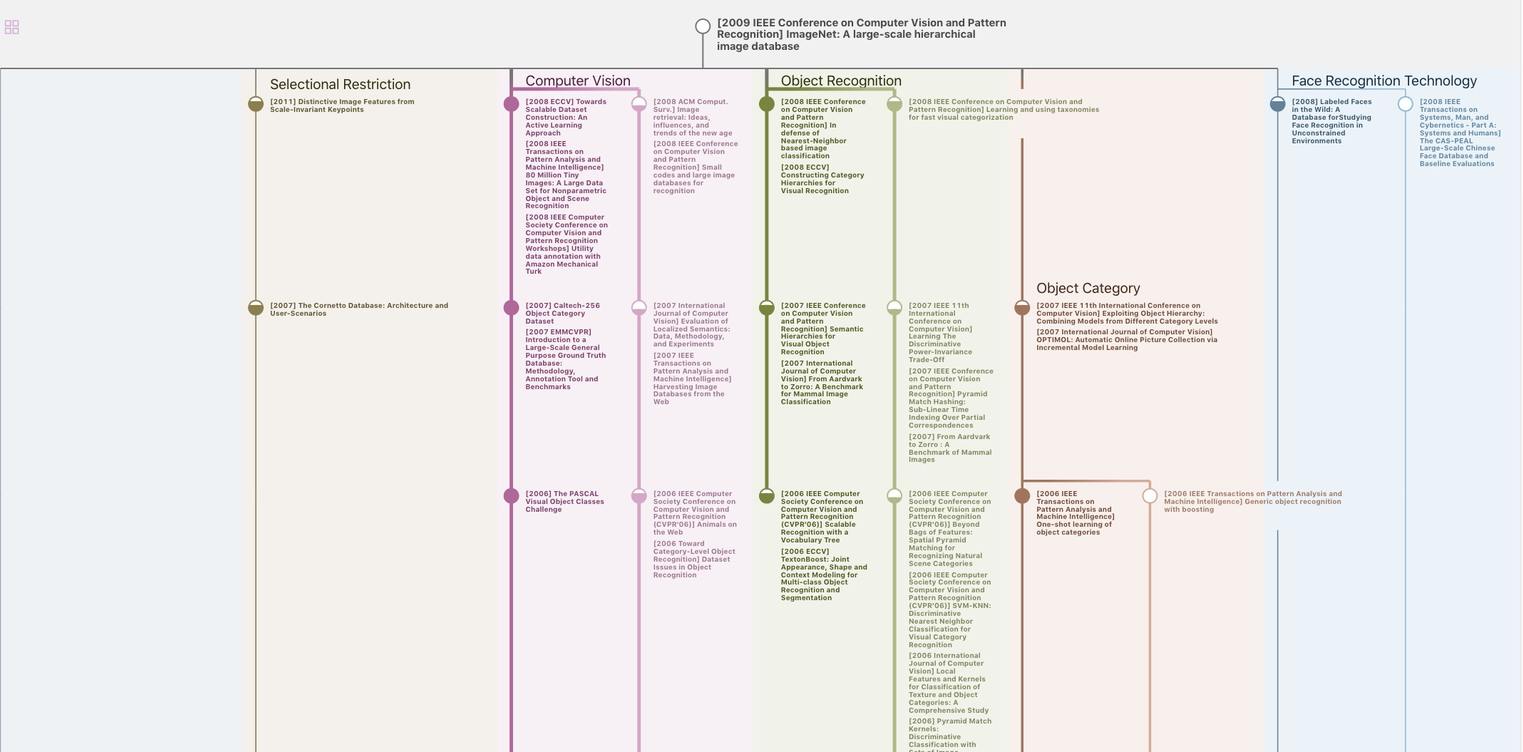
生成溯源树,研究论文发展脉络
Chat Paper
正在生成论文摘要