A deep learning approach for accurate and robust T1 estimation from cardiac magnetic resonance MOLLI sequences
European Heart Journal - Cardiovascular Imaging(2023)
摘要
Abstract Funding Acknowledgements Type of funding sources: None. Background T1 parametric maps of the myocardium are a recent development in cardiac Magnetic Resonance Imaging (MRI). They can be used to characterize the composition of the heart and identify alterations that can be indicative of a pathology. A common method of T1 estimation involves pixel-wise non-linear curve fitting from Modified Look-Locker Inversion Recovery (MOLLI) series [1]. Whilst relatively accurate, this method presents several drawbacks. Firstly, it assumes magnetization recovery to be a purely exponential process dependent on T1, thus neglecting other parameters such as T2 and B1 that can interfere in the process. Secondly, it does not consider that each acquisition during an inversion recovery experiment inverts the magnetization vector by a given angle and thus interferes with the recovery leading to the measurement of a shorter T1* rather than the actual T1 [2]. Additionally, since it considers each pixel individually, neglecting spatial information, it is sensitive to image quality [3]. Finally, since non-linear curve fitting is an iterative algorithm, it is computationally expensive and time-consuming. Methods To address these issues a convolutional neural network, DeepBLESS [4], and two spatially aware variants of it, were trained to predict T1, T2, and B1 with a dataset of MOLLI series simulated from experimental data. The simulation was performed using Bloch simulations and parameters extracted from a dataset composed of 27 single-slice MOLLI series acquired with the 5(3s)3 protocol using a b-SSFP readout at a main magnetic field strength of 3 Tesla. Thus, a dataset could be generated without relying on exponential fitting for ground truth generation. Results The exponential fitting of T1 was shown to consistently underestimate true T1 values by 138 +- 90 ms. In comparison, the spatially naïve DeepBLESS approach showed a mean absolute error of 14 +- 9 ms and the spatially aware DeepBLESS approach (Median-DeepBLESS) showed a mean absolute error of 13 +- 9 ms. As can be seen in Figure 1, Median-DeepBLESS also showed considerably more resilience to noise than exponential fitting and the spatially naïve version of DeepBLESS. Additionally, the deep learning approaches showcased promising results with regards to the estimation of T2 and B1 from MOLLI sequences (See Figure 2). Conclusions It was shown that the Deep Learning approaches eliminated the underestimation bias of exponential fitting with the spatially aware network Median-DeepBLESS being more robust to noise than both exponential fitting and the standard DeepBLESS network for myocardial T1 estimation. Relatively accurate estimation of T2 and B1 from MOLLI sequences was also accomplished through the Deep Learning approach, which may lead to simultaneous T1, T2, and B1 estimation in common clinical practice in the future.
更多查看译文
关键词
robust t1 estimation,deep learning,deep learning approach
AI 理解论文
溯源树
样例
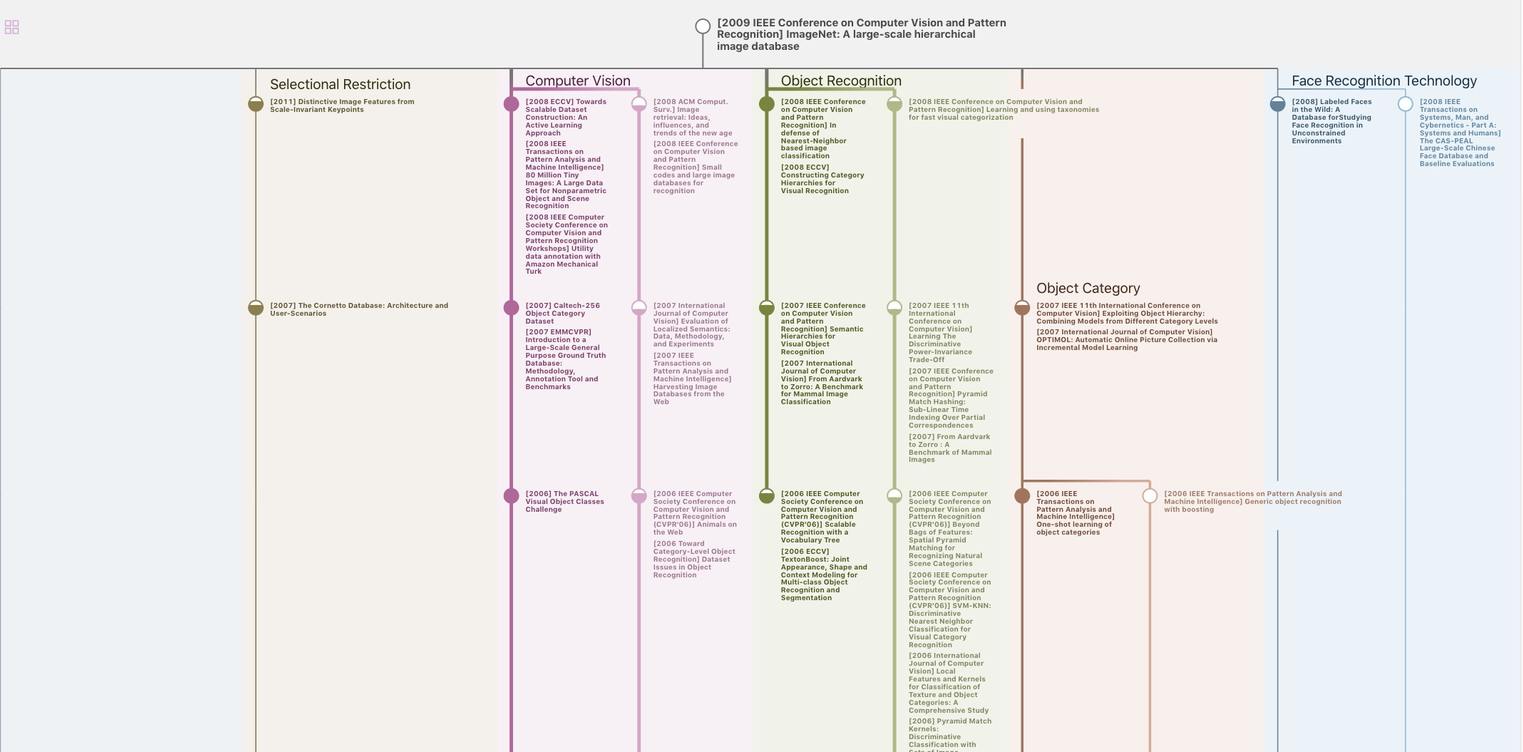
生成溯源树,研究论文发展脉络
Chat Paper
正在生成论文摘要