Abstract 5380: Systematic evaluation and comparison of drug response prediction models: a case study of prediction generalization across cell lines datasets
Cancer Research(2023)
摘要
Abstract Predictive modeling holds great promise for improving personalized cancer treatment and efficiency of drug development. In recent years, deep learning (DL) has been extensively explored for drug response prediction (DRP), outperforming classical machine learning in prediction generalization to new data. Despite the considerable interest in DRP, no agreed-upon methodology for evaluating and comparing the diverse DL models yet exists. Existing papers generally demonstrate the performance of proposed models using cross-validation within a single cell line dataset and compare with baseline models of their choice, substantially limiting the scope and validity of model evaluation and comparison. In this work, we investigate the ability of DRP models for generalizing predictions across datasets of multiple drug screening studies, a more challenging scenario mimicking practical applications of DRP models. Five cell line datasets and six community DRP models with advanced DL architectures have been explored. Public cell line drug screening datasets have been curated and processed for this analysis, including CCLE, CTRP, GDSC1, GDSC2, and GCSI. For each dataset, the same preprocessing pipeline was used to generate cell line gene expressions, drug representations, and drug response values. The six DRP models include advanced architectures and feature engineering methods such as transformer, graph neural network, and image representation of tabular data. Systematic model curation and training have been applied, including consistent training and testing data splits across models and hyperparameter optimization (HPO). To cope with the large-scale model training and HPO, automatic workflows have been implemented and executed on high-performance computing systems. A 5-by-5 matrix of prediction scores, corresponding to the five datasets in both row and column dimensions, has been generated for each model, with off-diagonal values representing the cross-dataset generalization. Despite the advanced DL techniques, all models exhibit substantially inferior performance in cross-dataset analysis as compared with cross-validation within a single dataset. This result demonstrates the challenge of cross-dataset generalization for DRP and motivates the need for rigorous and systematic evaluation of DRP models, which simulates real-world applications. Citation Format: Alexander Partin, Thomas S. Brettin, Yitan Zhu, Jamie Overbeek, Oleksandr Narykov, Priyanka Vasanthakumari, Austin Clyde, Sara E. Jones, Satishkumar Ranganathan Ganakammal, Justin M. Wozniak, Andreas Wilke, Jamaludin Mohd-Yusof, Michael R. Weil, Alexander T. Pearson, Rick L. Stevens. Systematic evaluation and comparison of drug response prediction models: a case study of prediction generalization across cell lines datasets. [abstract]. In: Proceedings of the American Association for Cancer Research Annual Meeting 2023; Part 1 (Regular and Invited Abstracts); 2023 Apr 14-19; Orlando, FL. Philadelphia (PA): AACR; Cancer Res 2023;83(7_Suppl):Abstract nr 5380.
更多查看译文
关键词
drug response prediction models,cell lines,prediction generalization
AI 理解论文
溯源树
样例
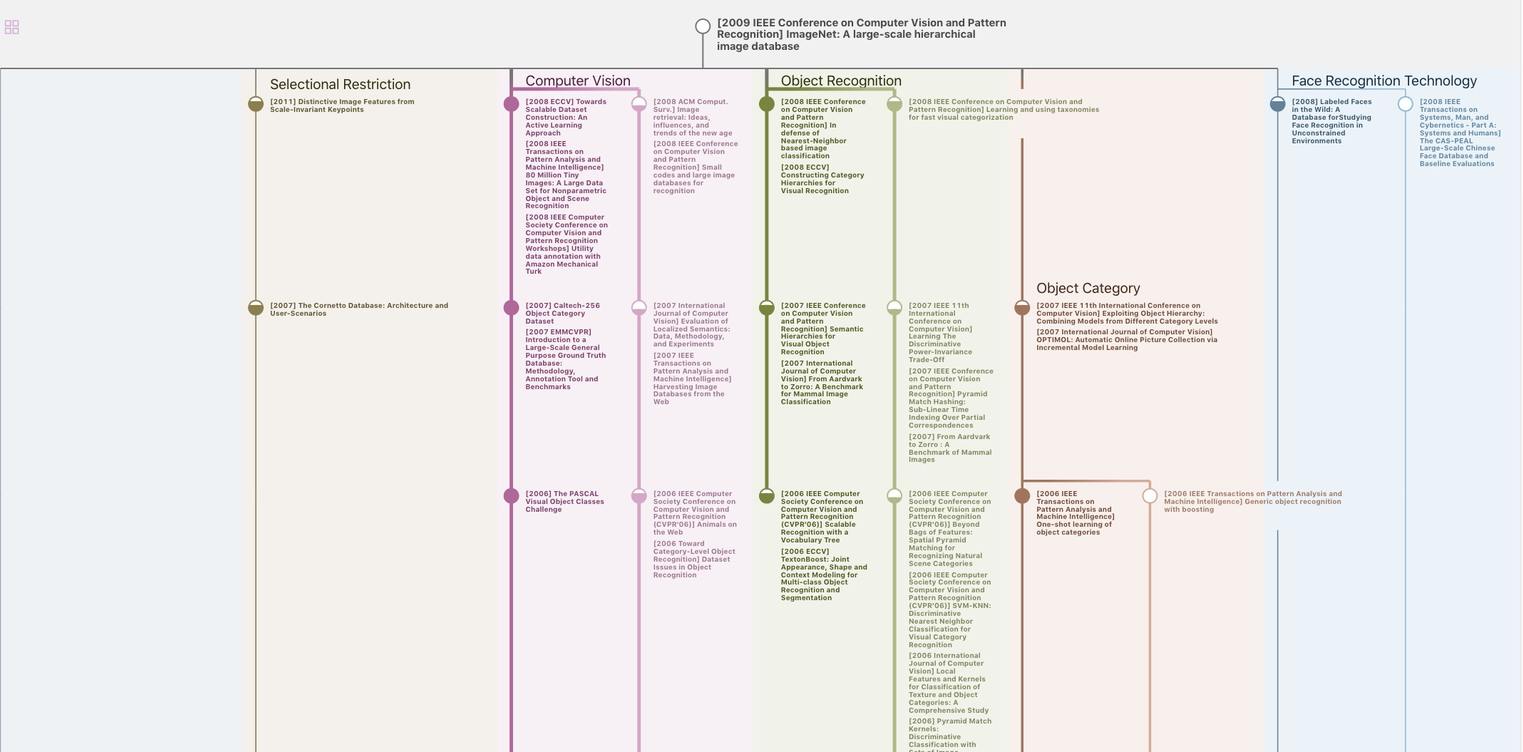
生成溯源树,研究论文发展脉络
Chat Paper
正在生成论文摘要