DiffCap: Exploring Continuous Diffusion on Image Captioning
arXiv (Cornell University)(2023)
摘要
Current image captioning works usually focus on generating descriptions in an autoregressive manner. However, there are limited works that focus on generating descriptions non-autoregressively, which brings more decoding diversity. Inspired by the success of diffusion models on generating natural-looking images, we propose a novel method DiffCap to apply continuous diffusions on image captioning. Unlike image generation where the output is fixed-size and continuous, image description length varies with discrete tokens. Our method transforms discrete tokens in a natural way and applies continuous diffusion on them to successfully fuse extracted image features for diffusion caption generation. Our experiments on COCO dataset demonstrate that our method uses a much simpler structure to achieve comparable results to the previous non-autoregressive works. Apart from quality, an intriguing property of DiffCap is its high diversity during generation, which is missing from many autoregressive models. We believe our method on fusing multimodal features in diffusion language generation will inspire more researches on multimodal language generation tasks for its simplicity and decoding flexibility.
更多查看译文
关键词
image captioning,diffcap,continuous diffusion
AI 理解论文
溯源树
样例
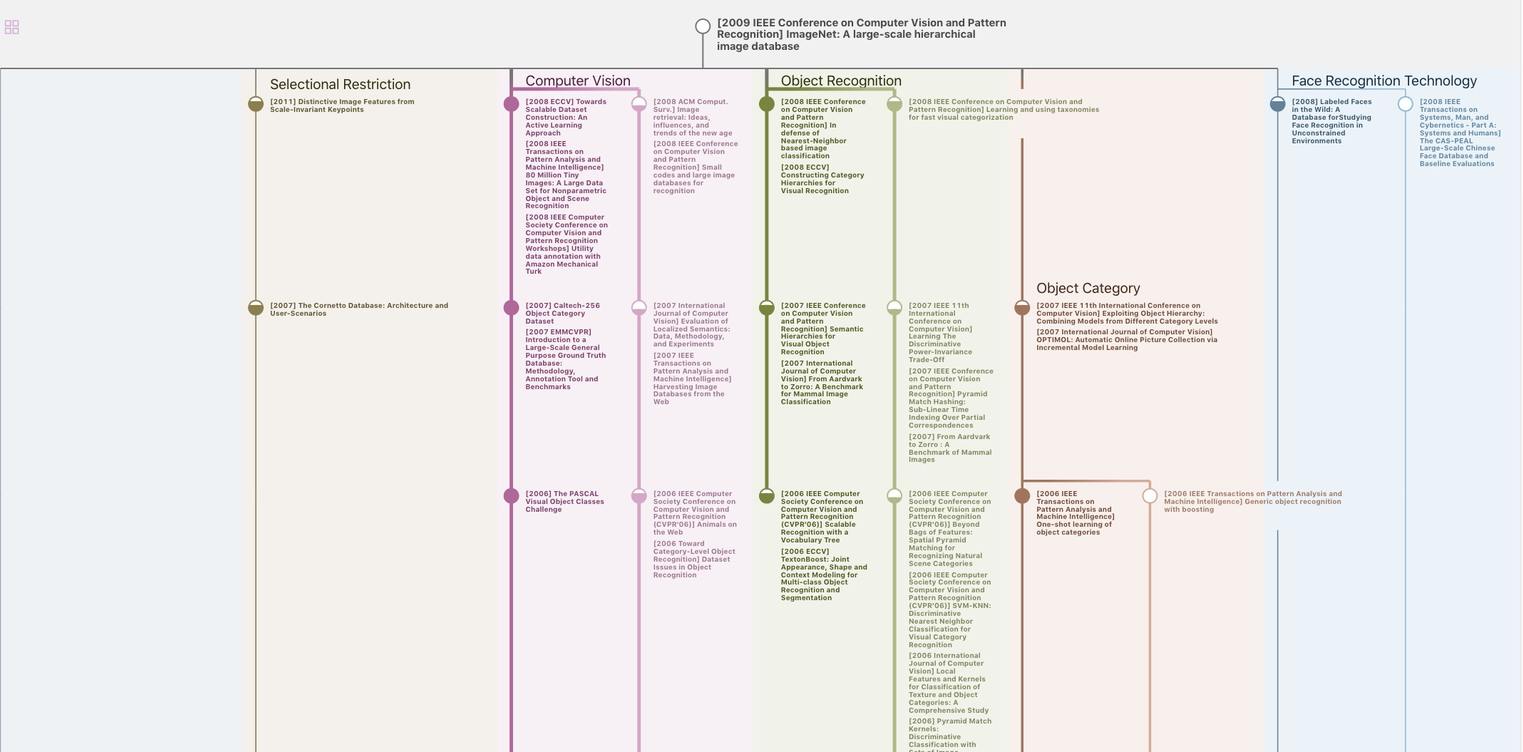
生成溯源树,研究论文发展脉络
Chat Paper
正在生成论文摘要