Deep Supervised Multi-View Learning With Graph Priors
IEEE TRANSACTIONS ON IMAGE PROCESSING(2024)
摘要
This paper presents a novel method for supervised multi-view representation learning, which projects multiple views into a latent common space while preserving the discrimination and intrinsic structure of each view. Specifically, an apriori discriminant similarity graph is first constructed based on labels and pairwise relationships of multi-view inputs. Then, view-specific networks progressively map inputs to common representations whose affinity approximates the constructed graph. To achieve graph consistency, discrimination, and cross-view invariance, the similarity graph is enforced to meet the following constraints: 1) pairwise relationship should be consistent between the input space and common space for each view; 2) within-class similarity is larger than any between-class similarity for each view; 3) the inter-view samples from the same (or different) classes are mutually similar (or dissimilar). Consequently, the intrinsic structure and discrimination are preserved in the latent common space using an apriori approximation schema. Moreover, we present a sampling strategy to approach a sub-graph sampled from the whole similarity structure instead of approximating the graph of the whole dataset explicitly, thus benefiting lower space complexity and the capability of handling large-scale multi-view datasets. Extensive experiments show the promising performance of our method on five datasets by comparing it with 18 state-of-the-art methods.
更多查看译文
关键词
Structure preservation,discriminant structure,common space,cross-view recognition,cross-modal retrieval
AI 理解论文
溯源树
样例
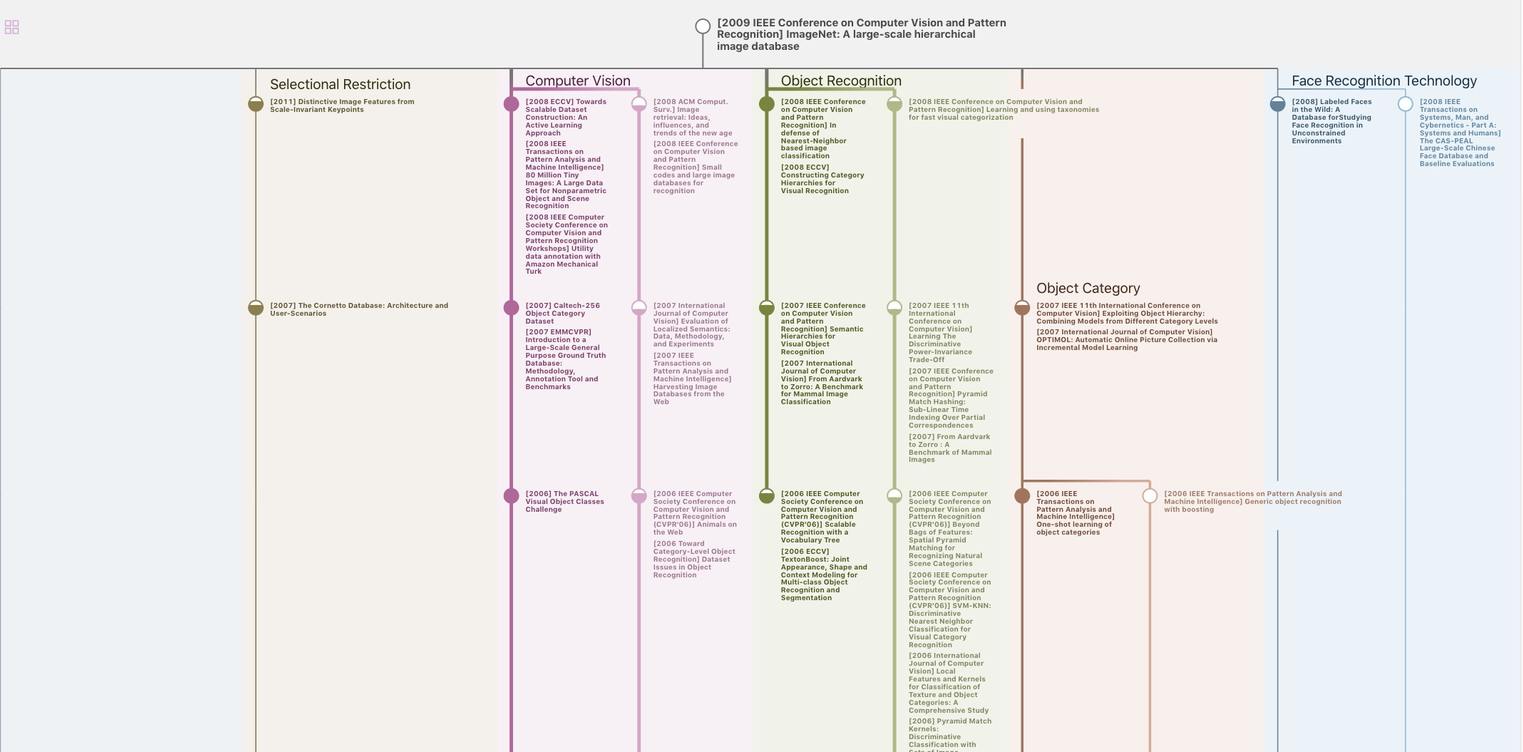
生成溯源树,研究论文发展脉络
Chat Paper
正在生成论文摘要