Rare, Protein-Altering Variants in AS3MT and Arsenic Metabolism Efficiency: A Multi-Population Association Study
Environmental Health Perspectives(2021)
摘要
Vol. 129, No. 4 ResearchOpen AccessRare, Protein-Altering Variants in AS3MT and Arsenic Metabolism Efficiency: A Multi-Population Association Studyis corrected byErratum: “Rare, protein-altering variants in AS3MT and arsenic metabolism efficiency: a multi-population association study” Dayana A. Delgado, Meytal Chernoff, Lei Huang, Lin Tong, Lin Chen, Farzana Jasmine, Justin Shinkle, Shelley A. Cole, Karin Haack, Jack Kent, Jason Umans, Lyle G. Best, Heather Nelson, Donald Vander Griend, Joseph Graziano, Muhammad G. Kibriya, Ana Navas-Acien, Margaret R. Karagas, Habibul Ahsan, and Brandon L. Pierce Dayana A. Delgado Department of Public Health Sciences, University of Chicago (UChicago), Chicago, Illinois, USA Search for more papers by this author , Meytal Chernoff Department of Public Health Sciences, University of Chicago (UChicago), Chicago, Illinois, USA Search for more papers by this author , Lei Huang Center for Research Informatics, UChicago, Chicago, Illinois, USA Search for more papers by this author , Lin Tong Department of Public Health Sciences, University of Chicago (UChicago), Chicago, Illinois, USA Search for more papers by this author , Lin Chen Department of Public Health Sciences, University of Chicago (UChicago), Chicago, Illinois, USA Search for more papers by this author , Farzana Jasmine Department of Public Health Sciences, University of Chicago (UChicago), Chicago, Illinois, USA Search for more papers by this author , Justin Shinkle Department of Public Health Sciences, University of Chicago (UChicago), Chicago, Illinois, USA Search for more papers by this author , Shelley A. Cole Texas Biomedical Research Institute, San Antonio, Texas, USA Search for more papers by this author , Karin Haack Texas Biomedical Research Institute, San Antonio, Texas, USA Search for more papers by this author , Jack Kent Texas Biomedical Research Institute, San Antonio, Texas, USA Search for more papers by this author , Jason Umans Georgetown-Howard Universities Center for Clinical and Translational Science, Washington, DC, USA Search for more papers by this author , Lyle G. Best Missouri Breaks Industries Research, Inc., Timber Lake, South Dakota, USA Search for more papers by this author , Heather Nelson School of Public Health, University of Minnesota, Minneapolis, Minnesota, USA Search for more papers by this author , Donald Vander Griend Department of Pathology, University of Illinois at Chicago, Chicago, Illinois, USA Search for more papers by this author , Joseph Graziano Mailman School of Public Health, Columbia University, New York City, New York, USA Search for more papers by this author , Muhammad G. Kibriya Department of Public Health Sciences, University of Chicago (UChicago), Chicago, Illinois, USA Search for more papers by this author , Ana Navas-Acien Mailman School of Public Health, Columbia University, New York City, New York, USA Search for more papers by this author , Margaret R. Karagas Department of Epidemiology, Geisel School of Medicine, Dartmouth College, Hanover, New Hampshire, USA Search for more papers by this author , Habibul Ahsan Department of Public Health Sciences, University of Chicago (UChicago), Chicago, Illinois, USA Department of Human Genetics, UChicago, Chicago, Illinois, USA Comprehensive Cancer Center, UChicago, Chicago, Illinois, USA Department of Medicine, UChicago, Chicago, Illinois, USA Search for more papers by this author , and Brandon L. Pierce Address correspondence to Brandon L. Pierce, The University of Chicago, 5841 S. Maryland Ave., MC2000, Chicago, IL 60637 USA. Telephone: (773) 702-1917. Email: E-mail Address: [email protected] Department of Public Health Sciences, University of Chicago (UChicago), Chicago, Illinois, USA Department of Human Genetics, UChicago, Chicago, Illinois, USA Comprehensive Cancer Center, UChicago, Chicago, Illinois, USA Search for more papers by this author Published:7 April 2021CID: 047007https://doi.org/10.1289/EHP8152AboutSectionsPDF Supplemental Materials ToolsDownload CitationsTrack Citations ShareShare onFacebookTwitterLinked InRedditEmail AbstractBackground:Common genetic variation in the arsenic methyltransferase (AS3MT) gene region is known to be associated with arsenic metabolism efficiency (AME), measured as the percentage of dimethylarsinic acid (DMA%) in the urine. Rare, protein-altering variants in AS3MT could have even larger effects on AME, but their contribution to AME has not been investigated.Objectives:We estimated the impact of rare, protein-coding variation in AS3MT on AME using a multi-population approach to facilitate the discovery of population-specific and shared causal rare variants.Methods:We generated targeted DNA sequencing data for the coding regions of AS3MT for three arsenic-exposed cohorts with existing data on arsenic species measured in urine: Health Effects of Arsenic Longitudinal Study (HEALS, n=2,434), Strong Heart Study (SHS, n=868), and New Hampshire Skin Cancer Study (NHSCS, n=666). We assessed the collective effects of rare (allele frequency <1%), protein-altering AS3MT variants on DMA%, using multiple approaches, including a test of the association between rare allele carrier status (yes/no) and DMA% using linear regression (adjusted for common variants in 10q24.32 region, age, sex, and population structure).Results:We identified 23 carriers of rare-protein-altering AS3MT variant across all cohorts (13 in HEALS and 5 in both SHS and NHSCS), including 6 carriers of predicted loss-of-function variants. DMA% was 6–10% lower in carriers compared with noncarriers in HEALS [β=−9.4 (95% CI: −13.9, −4.8)], SHS [β=−6.9 (95% CI: −13.6, −0.2)], and NHSCS [β=−8.7 (95% CI: −15.6, −2.2)]. In meta-analyses across cohorts, DMA% was 8.7% lower in carriers [β=−8.7 (95% CI: −11.9, −5.4)].Discussion:Rare, protein-altering variants in AS3MT were associated with lower mean DMA%, an indicator of reduced AME. Although a small percentage of the population (0.5–0.7%) carry these variants, they are associated with a 6–10% decrease in DMA% that is consistent across multiple ancestral and environmental backgrounds. https://doi.org/10.1289/EHP8152IntroductionMore than 200 million people are exposed to inorganic arsenic (iAs) through drinking water worldwide (Naujokas et al. 2013). Dietary exposure to iAs (primarily through rice and grain products) is an emerging concern that has received less regulatory focus (Nachman et al. 2017). Chronic exposure to levels of iAs above the World Health Organization (WHO) safety standard for drinking water (>10μg/L) has been recognized to pose a significant risk of adverse outcomes across multiple organ systems (Mohammed Abdul et al. 2015). Epidemiological studies in arsenic-affected areas of South America, Asia, and North America have demonstrated that chronic exposure to iAs is associated with adverse health effects, including increased risk for cardiovascular disease (Moon et al. 2017), diabetes (Sung et al. 2015), cognitive dysfunction (Karim et al. 2019; Tyler and Allan 2014), adverse birth outcomes (Milton et al. 2017), and overall mortality (Argos et al. 2010). In addition, iAs exposure increases the risk for cancers of the skin (Karagas et al. 2015), lung (Kuo et al. 2017; Lamm et al. 2015), bladder (Gamboa-Loira et al. 2017; Kuo et al. 2017), and kidney (Ferreccio et al. 2013).The metabolism of iAs in humans involves a series of reduction and methylation reactions. iAs enters the body as iAsIII (trivalent arsenite) or iAsV (pentavalent arsenate). Sequential reduction and methylation reactions are catalyzed by glutathione and arsenic (+3 oxidation state) methyltransferase (AS3MT), respectively, producing monomethylated (MMAIII and MMAV) and dimethylated (DMAIII and DMAV) forms of arsenic. Consumed arsenic is eliminated in the urine, primarily as a DMA, although smaller percentages are eliminated as MMA and iAs. Arsenic methylation facilitates excretion of arsenic in urine given that DMA is more rapidly expelled from the body compared with MMA or iAs (Gamble et al. 2006, 2007; Peters et al. 2015). There are a number of factors believed to impact individuals’ ability to metabolize arsenic, including genetic differences, age, sex, body mass index (BMI), smoking status, nutritional status, and arsenic exposure level (Jansen et al. 2016; Kordas et al. 2016; Shen et al. 2016). Arsenic metabolism efficiency (AME) is often represented by the percentage of arsenic species in the urine that are DMA (DMA%) (Hopenhayn-Rich et al. 1996; Vahter 1999).Common inherited genetic variation in the 10q24.32 region (containing AS3MT) is known to impact AME and the risk for arsenic-induced skin lesions. Two independent association signals for DMA% have been reported in the 10q24.32 region in a Bangladeshi cohort (Pierce et al. 2012, 2013). These DMA%-associated variants, best represented by single nucleotide polymorphisms (SNPs) rs9527 and rs11191527, were also associated with MMA%, iAs%, and skin lesion risk, highlighting the 10q24.32 region as a key source of individual variability in AME and susceptibility to arsenic toxicity. Studies in other arsenic-exposed populations have also reported associations between AS3MT SNPs and AME phenotypes (Agusa et al. 2009; Engström et al. 2011, 2015; García-Alvarado et al. 2018), including a study of American Indian populations in the United States with low-to-moderate levels of iAs exposure (Balakrishnan et al. 2017).Rare genetic variation [i.e., minor allele frequency (MAF) <1%] may also impact AME. Gao et al. (2015) estimated the heritability of DMA% due to common SNPs to be ∼16% [95% confidence interval (CI) (−7.5%, 39.5%) based on a standard error (SE) of 12%] in a sample of unrelated Bangladeshi individuals; however, when restricting to only close relatives, the heritability estimate increased to 63% [95% CI: (31.6%, 94.4%), based on a SE of 16%], potentially reflecting the contributions of rare variants. Family studies in American Indian communities also reported DMA% heritability estimates >50% (Tellez-Plaza et al. 2013), also suggesting that rare variants may contribute to the heritability of AME. However, the contribution of rare variants to AME has not been assessed for any specific genes, including AS3MT.In this study, our primary goal was to characterize the impact of rare, protein-altering variants in AS3MT on AME in three different arsenic-exposed populations. This multicohort approach allows us to assess the generalizability of our findings across cohorts of different ancestral and environmental backgrounds. Understanding the role of rare variation is critical for identifying individuals at high risk for arsenic toxicities and understanding the biological mechanisms underlying interindividual differences in susceptibility to toxicity.MethodsStudy ParticipantsThis study uses data from three different studies of arsenic-exposed populations: the Health Effects of Arsenic Longitudinal Study (HEALS), the Strong Heart Study (SHS), and the New Hampshire Skin Cancer Study (NHSCS). We selected these three cohorts because of their known arsenic exposure, existing data on arsenic species, and available DNA for sequencing.HEALS is a prospective cohort study designed to investigate health outcomes associated with chronic arsenic exposure through drinking water in Araihazar, Bangladesh (Ahsan et al. 2006). Arsenic concentrations have been measured in >5,000 wells in the study area. A total of 11,746 participants (5,042 males and 6,704 females, 18–75 years of age) were enrolled between 1999 and 2001 after providing informed consent. Participants completed a written questionnaire and participated in clinical exams at baseline. Blood and urine samples were also collected from participants at baseline (Ahsan et al. 2006). Participants were simultaneously assessed for arsenical skin lesions at baseline and every 2 y thereafter by trained physicians using a structured protocol (Argos et al. 2011). Arsenic species in baseline urine samples were measured previously for 4,794 HEALS participants (Jansen et al. 2016). For the present study, we selected 2,719 of these participants who had available DNA for sequencing, of which 273 were skin lesion cases at baseline. Although many of these participants were randomly selected for arsenic species measurement in baseline urine, a sizable fraction (∼50%) were selected at subsequent follow-up visits based on outcomes they experienced (i.e., skin lesions, respiratory symptoms, and cardiovascular conditions) in a case–cohort fashion. So although this group was relatively healthy at baseline, the participants were not randomly selected.The SHS is the largest population-based cohort study of cardiovascular disease in American Indian men and women. The SHS includes 12 American Indian tribes and communities and was designed to estimate cardiometabolic disease morbidity and mortality and the prevalence of its risk factors (Moon et al. 2013). Between 1989 and 1991, the SHS recruited 4,549 American Indian men and women between the ages of 45 to 74. These participants have been exposed to low-to-moderate levels of iAs primarily through drinking water (Navas-Acien et al. 2009) and to a lower extent, diet (i.e., rice) (Nigra et al. 2019). Arsenic species were measured in 3,973 participants (Moon et al. 2013). These individuals are members of large families, so we restricted participation to a group of 997 unrelated individuals with existing measures of arsenic species and available DNA for sequencing.The NHSCS is a population-based case–control study of basal cell carcinoma (BCC) and squamous cell carcinoma (SCC) of the skin (Gilbert-Diamond et al. 2013). Invasive SCC incident cases (n=510), 25–74 years of age, were recruited from >90% of practicing dermatologist and pathologist clinics in New Hampshire and bordering areas. Controls (n=483) frequency-matched on sex and age were selected from the New Hampshire Center for Medicare and Medicaid Services and driver’s license records. The enrollment period for cases and controls was between 2003 and 2009. Participants interviewers masked to the study hypothesis ascertained sociodemographic, lifestyle, medical, and sun-exposure information. In addition, home water and spot urine samples were collected and used to measure total urinary arsenic concentration and arsenic species (post-diagnosis measurements for SCC cases) (Gilbert-Diamond et al. 2013). Of the 993 cases and controls, 288 individuals did not have sufficient DNA for sequencing and were excluded from the present study. This resulted in 706 individuals with existing data on arsenic species selected for the present sequencing study (349 SCC cases and 357 controls).Measurement of Arsenic Metabolites in UrineAll participants selected for this study had existing data on arsenic species in urine. In all three cohorts, speciation analysis of arsenic metabolites was performed using high-performance liquid chromatography (HPLC) (Scheer et al. 2012) followed by detection using ICPMS (Ahsan et al. 2006; Gilbert-Diamond et al. 2011; Moon et al. 2013). Details regarding the limit of detection (LOD) for each metabolite and percentage of samples below the LOD have been described previously (Ahsan et al. 2006; Gilbert-Diamond et al. 2011; Navas-Acien et al. 2009). Briefly, in HEALS the LOD was 1μg/L for iAsIII, iAsV, MMA, and DMA. In SHS, iAsIII was first oxidized to iAsV in the urine, following the methods described by Scheer et al. (2012), this method minimizes undetectable iAsIII or iAsV in urine samples from populations with low levels of exposure. The LOD was 0.1μg/L for iAsV and 0.5μg/L for MMA and DMA in SHS. In NHSCS, the LOD was 0.15μg/L for iAsIII, 0.1μg/L for iAsV, 0.14μg/L for MMA, and 0.11μg/L for DMA.For this analysis, iAsIII and iAsV were summed to obtain total iAs, and each arsenic species (iAs, MMA, and DMA) is expressed as a percentage of the sum of each of these three species (iAs+MMA+DMA). Arsenocholine (AsC) and arsenobetaine (AsB) are nontoxic forms of (organic) arsenic and were excluded from our analyses. We used DMA% as our primary measure of AME; MMA% and iAs% were used as secondary measures of AME. In the SHS and NHSCS, measures of AME were not computed if ≥2 species were undetectable for an individual. If only one metabolite was undetectable, this missing metabolite was estimated as the LOD divided by the square root of 2 and was used in downstream analyses. For the present study, of the 2,719 HEALS participants, 523, 155, and 4 participants had values below the LOD for iAsIII, iAsV, and MMA, respectively. We kept these participants in our analyses and set arsenic species values that were 60), RMSMappingQuality (MQ<40), StrandOddsRatio (SOR>3), MappingQualityRankSumTest (MQRankSum<−12.5), or ReadPosRankSum<−8. We removed indels that met at least one of the following conditions: QD<2.0, FS>200, ReadPosRankSum>−20, InbreedingCoeff<−0.8, or SOR>10. The first filter excluded 141 bi-allelic variants and 17 indels, resulting in 256 variants passing all the quality metrics. In addition, we excluded all variants with call rates of <90%, resulting 135 for HEALS, 92 for SHS, and 120 for NHSCS. Overall, we were able to sequence 9 of the 11 exons in AS3MT (Illumina was unable to design flanking probes for Exons 5 and 10) at a depth of coverage ranging between 173 and 729 per exon, across all cohorts (Figure 1).Figure 1. Average aligned read depth (depth of coverage) of AS3MT exons. Nine of 11 exons (excluding Exons 5 and 10) in AS3MT were sequenced with an average depth of coverage across all cohorts ranging from 173×to 729× (30× is deemed high quality). Rare, protein-altering variants were identified across all cohorts: five variants in HEALS in Exons 4 and 6, four variants in SHS in Exons 4, 8, 9, and 11, and five in NHSCS in Exons 6, 7, 9, and 11. Note: CCDS, Consensus Coding Sequence project; chr, chromosome; Human Feb. 2009, February 2009 Human reference sequence; HEALS, Health Effects of Arsenic Longitudinal Study; NHSCS, New Hampshire Skin Cancer Study; Rfam, RNA families; SHS, Strong Heart Study; UCSC, University of California, Santa Cruz.Among the 2,719 HEALS participants selected for this study, we excluded 260 participants because of a low depth of coverage (<30×) and 25 participants who did not have genome-wide SNP data available for the generation of a kinship matrix, resulting in 2,434 remaining HEALS participants. Among the 997 SHS participants, we excluded 123 participants whose samples had extremely low coverage due to a very low number of reads and 6 participants with missing metabolite data, leaving 868 SHS participants. Among the 705 NHSCS participants selected for this study, we removed 39 samples owing to missing metabolite data and one sample due to a low number of reads, resulting in 666 NHSCS participants.In an effort to validate our sequenced variants, we used existing HEALS SNP array and exome array data (Pierce et al. 2019) for 2,363 of the HEALS participants sequenced for this study. We identified 721 overlapping variants in both data sets (sequencing data and imputed SNP array data). We compared genotypes for each variant and found that 645 of 721 variants had >90% consistent genotypes across 2,363 individuals. Only 3 variants had <60% consistency. In addition, we used the Genome Aggregation Database (gnomAD) version 2.1.1 (Karczewski et al. 2020) to validate observed variants and to obtain an estimate of the rare variants missed in our study (specifically in Exons 5 and 10). GnomAD provides exome sequencing data for >125,000 unrelated individuals, most of which are classified into six ancestral populations (African American, Latino, East Asian, Finnish, non-Finish European, and South Asian).Variant AnnotationAll variants were annotated using the Annotate Variation (ANNOVAR) software (Wang et al. 2010), which provided information on the impact of each exonic variant on the amino acid sequence (e.g., nonsynonymous, synonymous, frameshift indel). A variant was annotated as a predicted loss of function variant (pLoF) if they were categorized by ANNOVAR as frameshift, premature stop codon, loss of start or stop codon, or splice acceptor or donor. For this analysis, we focused on variants with a MAF of<0.01 that altered the protein sequence. Thus, we excluded all synonymous, intronic, and intergenic variants detected. These exclusions resulted in five, four, and five AS3MT rare, protein-altering variants in the HEALS, SHS, and NHSCS cohorts, respectively.We used the sorting intolerant from tolerant (SIFT) (Ng and Henikoff 2003) and PolyPhen (Adzhubei et al. 2010) tools to predict how variants coding for amino acid changes impact protein function. SIFT uses information on sequence homology and physical properties of amino acids to predict the impact on protein function, whereas PolyPhen uses sequence, phylogenetic, and structural information to empirically predict the effect of the substitution. We also report Combined Annotation Dependent Depletion (CADD) scores (Rentzsch et al. 2019) for the variants we analyzed, which are based on evolutionary information, conversion metrics, functional genomic data, and transcription information. CADD scores are Phred-like C-scores that rank variants relative to all possible substitutions of the human genome (8.6×109). The CADD score represents the potential level of deleteriousness. For instance, variants with CADD scores of 0–10 are in the top 10% most deleterious, CADD scores 10–20 are in the top 1%, CADD scores 20–30 are in the top 0.1%, and so on.Identification of Common Variants in 10q24.32 Region Associated with DMA%In light of the well-established associations between common variants in the 10q24.32 region and AME, we used our sequencing data to genotype common variants in the 10q24.32 region and identify variants showing independent associations with DMA% in each population. We performed linear conditional, forward stepwise regression tests, stratified by cohort. We restricted analyses to 4,990 variants in the 10q24.32 target region and excluded variants with a Hardy-Weinberg p<1×10−10 (n=122) and variants with a MAF<0.005 (n=4,485). This quality control resulted in 383 variants across all cohorts (HEALS n=2,436, SHS n=874, and NHSCC n=752).Linear conditional, forward stepwise regression tests consisted of a series of association analyses. To identify the primary association signal, we individually tested each of the 383 common variants for an association with DMA%, controlling for age, sex, and population structure in HEALS and SHS. We used PLINK (Purcell et al. 2007) for SHS and NHSCS and genome-wide complex trait analysis (GCTA) (Yang et al. 2011) for HEALS (for adjustment of kinship matrix). To identify secondary independent association signals, we included the primary signal (identified in the previous analyses) as a covariate and repeated our association analyses. We repeated these analyses, adjusting for both the primary and secondary signals, to identify any remaining independent signals in each population. These analyses resulted in the identification of two independent lead variants in HEALS, rs12573221 (p=6.4×10−12) and rs14553735 (p=7×10−11), three independent lead variants in SHS, rs10786722 (p=1×10−20), rs4919687 (p=7.9×10−9), and rs10883846 (p=4.7×10−16), and a single lead variant in NHSCS, rs76255497 (p=7×10−5). We conducted analyses of MMA% and iAs% and found that the DMA% associated lead variants were consistently among the top five lead independent variants in analyses of MMA% and iAs%, across all cohorts. Given this agreement in results across metabolites, we adjusted for the DMA% associated individual SNP alleles (0, 1, or 2) in our linear regressions. This adjustment ensured association estimates were not biased due to linkage disequilibrium (LD) between common variants known to impact AME and the rare variants studied in this work.Statistical AnalysisThe classical single-variant–based association test is not typically extended to analyses of rare variants because of a lack of statistical power (Asimit and Zeggini 2010). Instead, investigators have developed methods that aggregate variants in a biologically relevant region (i.e., a gene) and evaluate their cumulative effects. This approach is now the standard for rare variant studies and can provide reasonable power to detect association between a gene-based set of rare variants and a human trait (Lee et al. 2014). In the present study, the primary hypothesis was that carrying a rare, protein-altering variant in AS3MT reduces AME (represented by DMA%). To test this hypothesis, we conducted a burden test, where we assigned each individual a binary carrier status and tested whether the mean of DMA% differs between carriers and noncarriers (using linear regression). Individuals carrying at least one rare, protein-altering variant in AS3MT were assigned a carrier status of 1, and 0 otherwise. We did not observe any individuals carrying more than one rare, protein-altering variant in AS3MT. The burden test makes the strong assumption that all variants analyzed in the gene are causal and affect the trait in the same direction and with the same magnitude. Violation of these assumptions may result in loss of power (Neale et al. 2011).We fit linear regression models for SHS and NHSCS, adjusted for age and sex, with carrier status as the predictor and arsenic metabolites (DMA%, MMA%, and iAs%) as outcomes. For HEALS, we conducted the burden test using a mixed-linear model based association test (–mlma) and incorporated a kinship matrix as implemented in the GCTA software (Yang et al. 2011) to control for cryptic relatedness among participants. For SHS, we accounted for population structure by adjusting for the first five principle components (PCs) derived from existing genome-wide SNP data described previously (PCs provided by SHS) (Matise et al. 2011). We were not able to control for population structure in NHSCS because of the lack of existing genome-wide SNP data; however, 99.5% of NHSCS participants included in this study self-reported as non-Hispanic white (only three individuals self-reported as Hispanic or Latino). To ensure minimal bias due to population structure, we conducted the same burden test excluding the three individuals who self-reported as Hispanic or Latino. In addition, we performed the burden test with adjustment and without adjustment for common SNPs in the 10q24.32 region.To address the possibility of bias in the association between rare variant carrier status and AME due to prevalent skin lesion and SCC case status, we repeated the burden tests excluding 293 prevalent skin lesion cases in HEALS (1 skin lesion case was a carrier of the rs3523887 rare variant) and 349 SCC cases in NHSCS. We analyzed 2,076 HEALS individuals without prevalent skin lesions and 357 NHSCS controls and adjusted for age, sex, relatedness (in HEALS only), and common SNPs in the 10q24.32 region. To estimate the association between carrier status and DMA% across all
更多查看译文
关键词
arsenic metabolism efficiency,protein-altering,multi-population
AI 理解论文
溯源树
样例
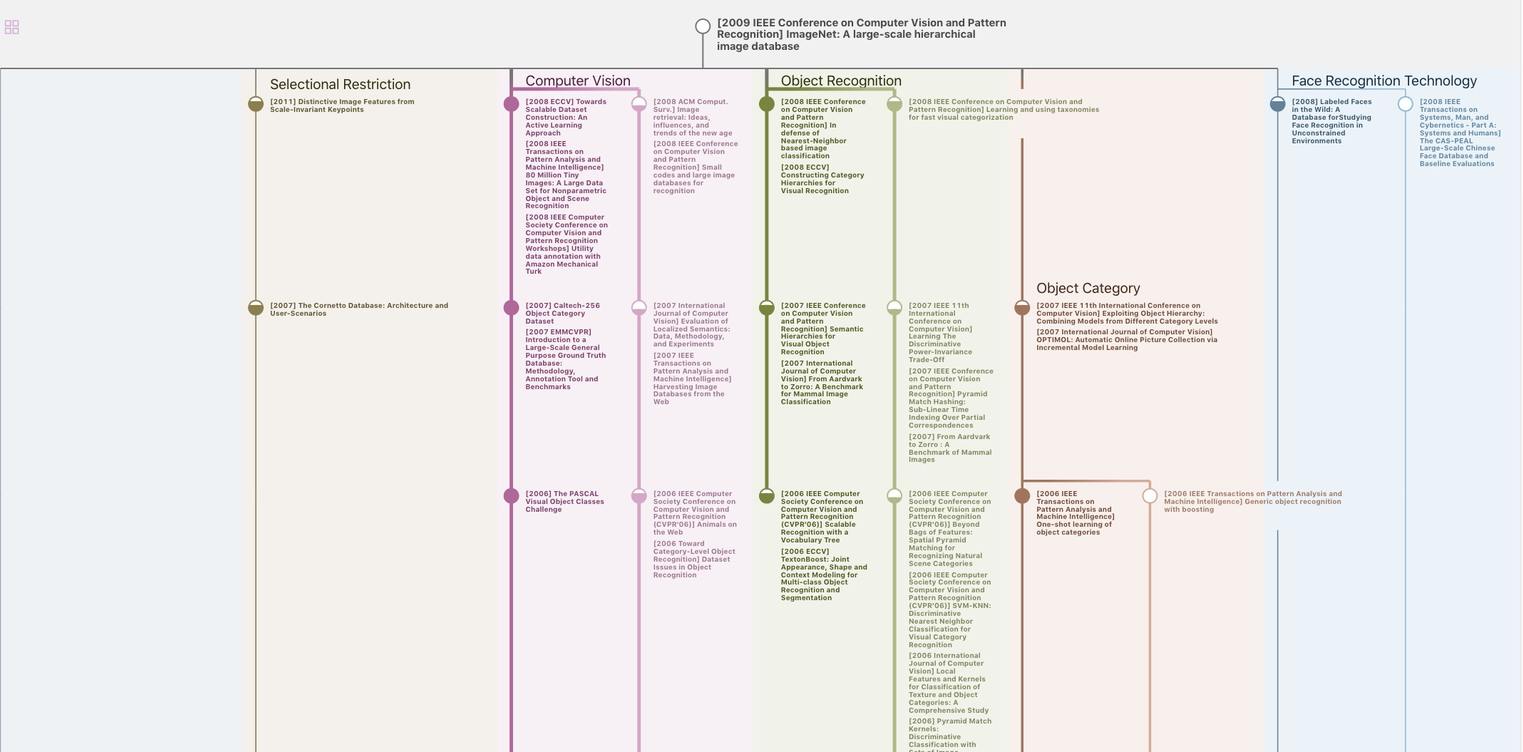
生成溯源树,研究论文发展脉络
Chat Paper
正在生成论文摘要