Face using of Classifiers
Advances in intelligent systems and computing(2019)
摘要
In this paper, we have proposed an efficient and computationally inexpensive approach toward two mainstreams of image recognition, i.e, face recognition and person identification. Our proposed model is invariant to pose, expression, scale, illumination, and translation with the application of different techniques and implementation of their algorithms. For the purpose of making the model illumination invariant, we have preprocessed the image with linear transformation. Features are extracted from preprocessed facial images using a Modified-Local Difference Binary descriptor which incorporates gradient knowledge from a nonlinear scale space. The extracted facial features are then quantized to a vector. Subsequently, an extreme gradient boosting algorithm is used, resulting in fast and high-performance classification. The proposed method has been experimented on three benchmark datasets like GRIMACE, FACES95, FACES96 producing significant results in terms of speed, accuracy, and efficiency. We extended our proposed method and tested it on Raspberry Pi 3 to conclude that it is fast on limited processor and memory settings. This pipeline has resulted in a faster and efficient face recognition approach with decrease in error rate around +8 to +10%.
更多查看译文
关键词
classifiers
AI 理解论文
溯源树
样例
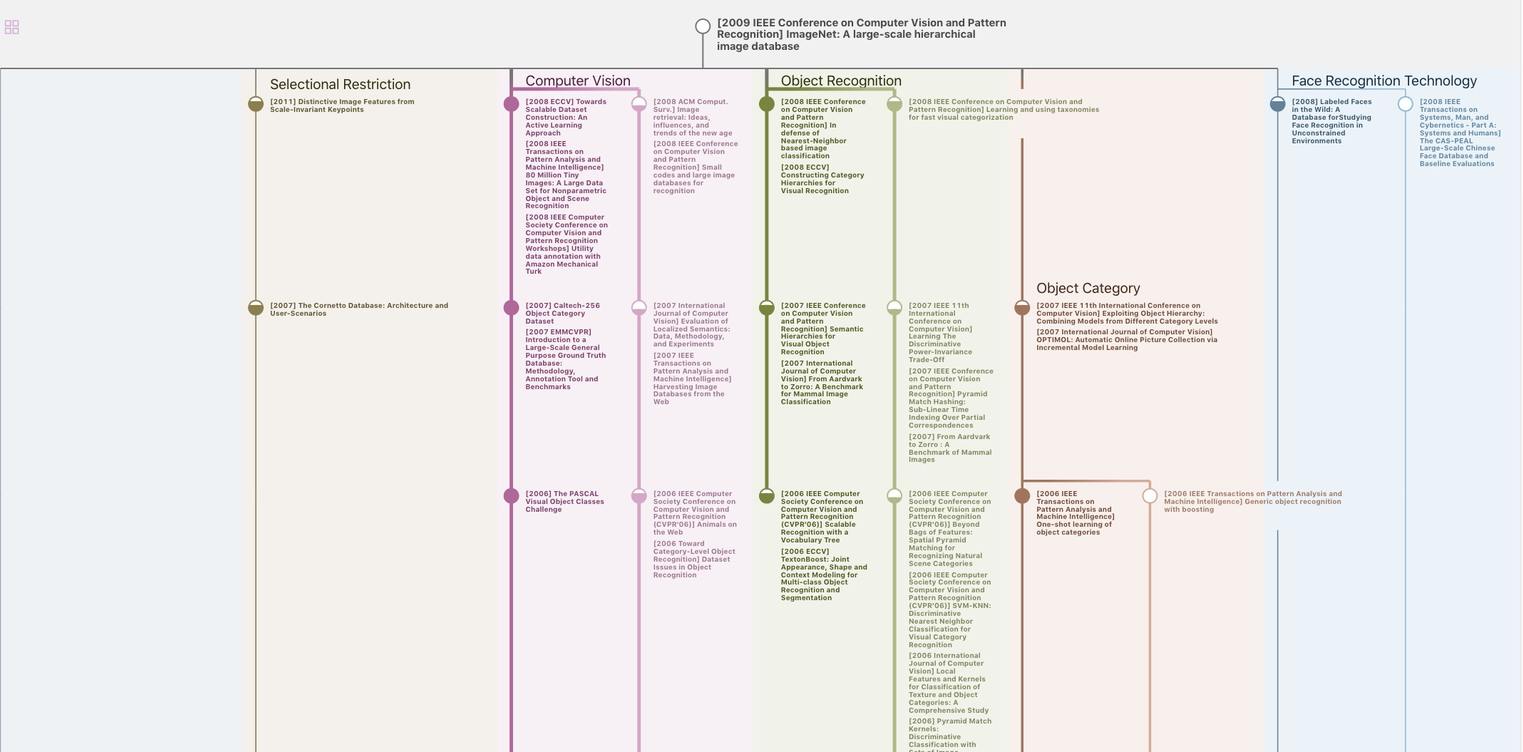
生成溯源树,研究论文发展脉络
Chat Paper
正在生成论文摘要