SSGCNet: A Sparse Spectra Graph Convolutional Network for Epileptic EEG Signal Classification
arXiv (Cornell University)(2022)
摘要
In this article, we propose a sparse spectra graph convolutional network (SSGCNet) for solving Epileptic EEG signal classification problems. The aim is to achieve a lightweight deep learning model without losing model classification accuracy. We propose a weighted neighborhood field graph (WNFG) to represent EEG signals, which reduces the redundant edges between graph nodes. WNFG has lower time complexity and memory usage than the conventional solutions. Using the graph representation, the sequential graph convolutional network is based on a combination of sparse weight pruning technique and the alternating direction method of multipliers (ADMM). Our approach can reduce computation complexity without effect on classification accuracy. We also present convergence results for the proposed approach. The performance of the approach is illustrated in public and clinical-real datasets. Compared with the existing literature, our WNFG of EEG signals achieves up to 10 times of redundant edge reduction, and our approach achieves up to 97 times of model pruning without loss of classification accuracy.
更多查看译文
关键词
epileptic eeg
AI 理解论文
溯源树
样例
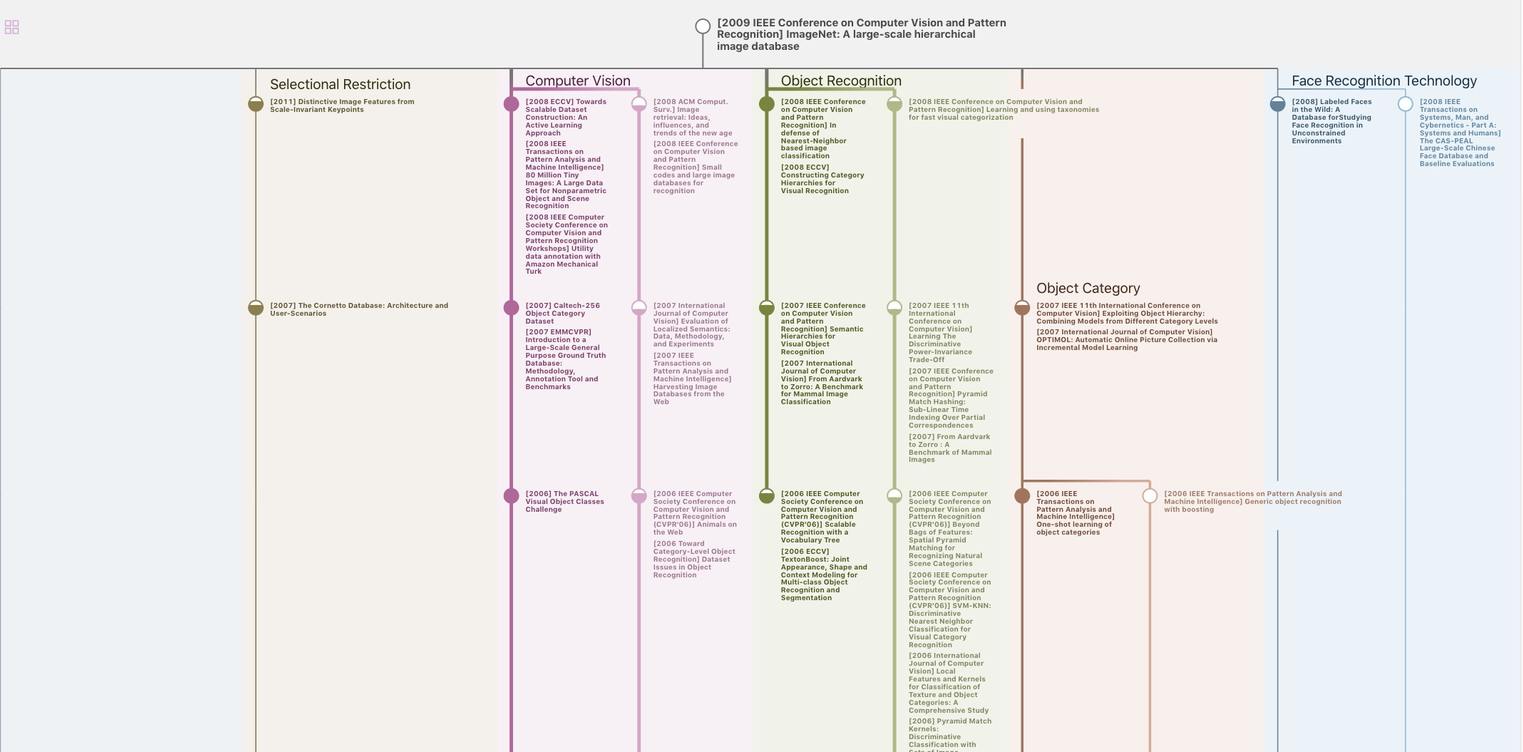
生成溯源树,研究论文发展脉络
Chat Paper
正在生成论文摘要