Heart Disease Prediction Based on MWMOTE and Res-BiGRU Models
2023 IEEE 6th International Conference on Pattern Recognition and Artificial Intelligence (PRAI)(2023)
摘要
Predicting the incidence rate of heart disease is of inestimable significance in the field of clinical medicine and health management. In recent years, machine learning and artificial intelligence technologies have developed rapidly, offering new possibilities for the prediction and diagnosis of heart disease. However, there are challenges in collecting disease data, as the number of diseased samples is often much larger than the normal sample size. Moreover, traditional prediction methods struggle to fully extract the spatio-temporal characteristics of the data, resulting in low prediction accuracy and issues such as gradient dispersion in complex prediction systems. To address these challenges, this paper proposes a new heart disease prediction model, which is based on the Minority Weighted Majority Oversampling Technique (MWMOTE) and improved Residual Bidirectional Gated Recurrent Unit (Res-BIGRU). The model utilizes the MWMOTE oversampling method to balance the positive and negative samples of the dataset, and employs CNN and BiGRU to extract features, while using residual connections to avoid gradient dispersion and alleviate network degradation. The model's optimal parameters are determined through continuous experiments. Testing and evaluating the model reveals an accuracy of 0.98. In comparison to multiple traditional prediction models reproduced for the experiment, this model demonstrates higher prediction accuracy, enabling better assistance for doctors in diagnosing heart disease.
更多查看译文
关键词
MWMOTE,BiGRU,Resnet,Heart disease prediction
AI 理解论文
溯源树
样例
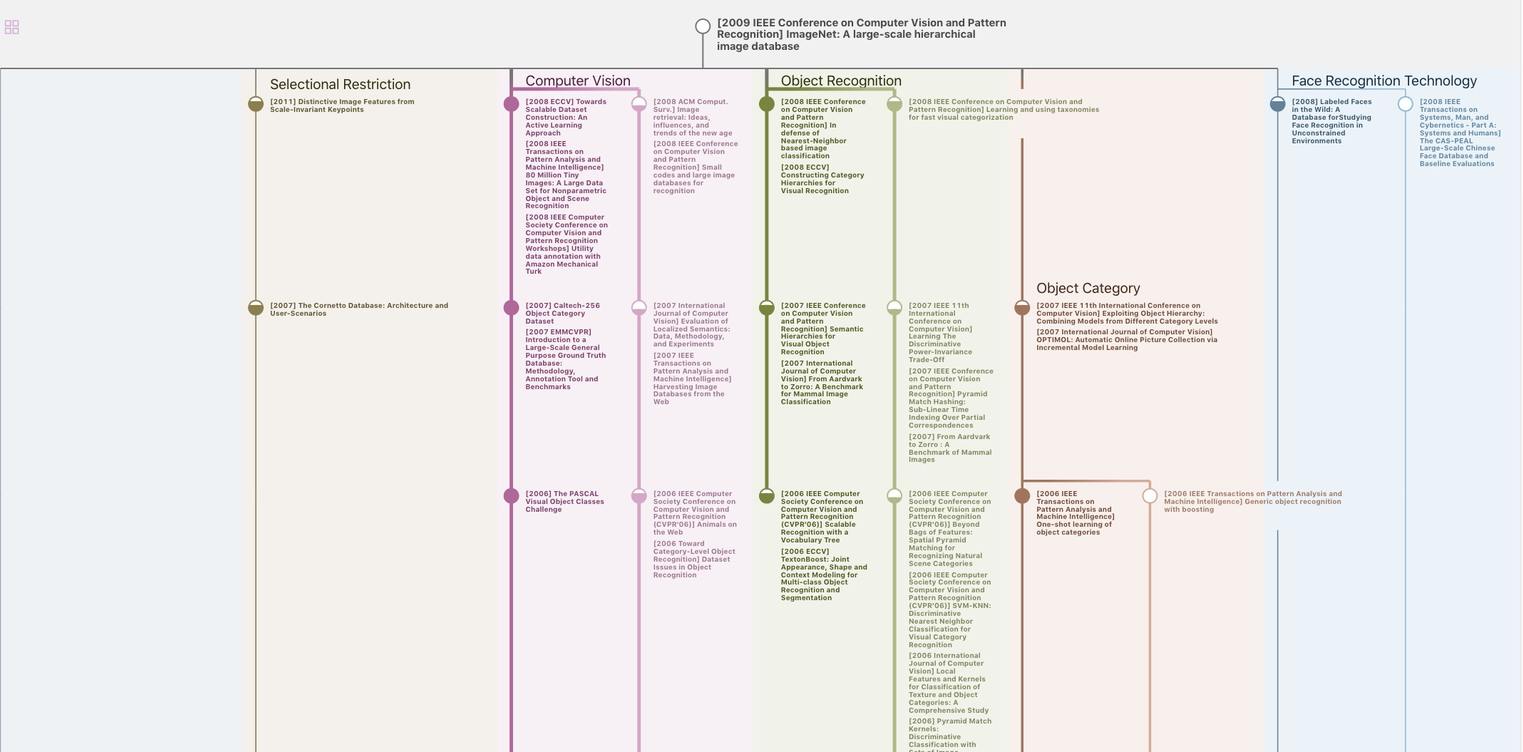
生成溯源树,研究论文发展脉络
Chat Paper
正在生成论文摘要