Towards an EMG-Based Decoder of Natural Motor Intentions
2023 IEEE Ural-Siberian Conference on Computational Technologies in Cognitive Science, Genomics and Biomedicine (CSGB)(2023)
摘要
Despite recent advances, the development of systems that combine electromyographic activity with the recognition of natural intentions to move individual fingers or complex gestures remains a challenge. The challenge is to develop systems to teach patients how to control a prosthesis in the early stages of amputation. The study includes development of a system for non-contact acquisition of synchronized hand kinematics and high-density EMG electrodes grid from a small area of the wrist; identification of significant features in the EMG activity for model training; testing the existing neural network architectures for movement prediction; development of a system for iterative cooperative training of an emulated patient and a virtual prosthesis control algorithm. Eight healthy subjects with no history of neuromuscular disorders participated in the study. An online procedure was developed to synchronize the time series of kinematic parameters estimated from the video stream and the 64-channel EMG collected by a high-density electrode grid (4×4 cm) placed over the flexor carpi radialis, palmaris longus, and flexor carpi ulnaris muscles. The experimental paradigm included continuous movements of individual fingers and fixation of 7 wrist gestures in each of the 4 arm positions. Independent component analysis (ICA) combined with the mutual information (MI) metrics showed the presence of components related to the movement of each individual finger. On average, 2.3 (SD = 0.7) EMG components were identified as having a high degree of MI with kinematics. An iterative procedure was developed based on increasing the complexity of the tasks for synchronized execution of movements of the restricted hand emulating an amputee. Decoder training at each step used the mirrored kinematics of the “healthy” (unrestricted) hand. The model predictions were evaluated by calculating the mean angle of deviation from the real movements (MAE score). The best accuracy was observed for decoding single finger movements (9±2 degrees error standard deviation) and restricted finger movements (10±2 degrees error standard deviation), while the lowest results are for movements from the fist (20±5). The developed iterative subject training procedure demonstrates good convergence and can potentially be used for training amputees when no ground truth is available.
更多查看译文
关键词
electromyography,finger movements,decoding,motor intentions
AI 理解论文
溯源树
样例
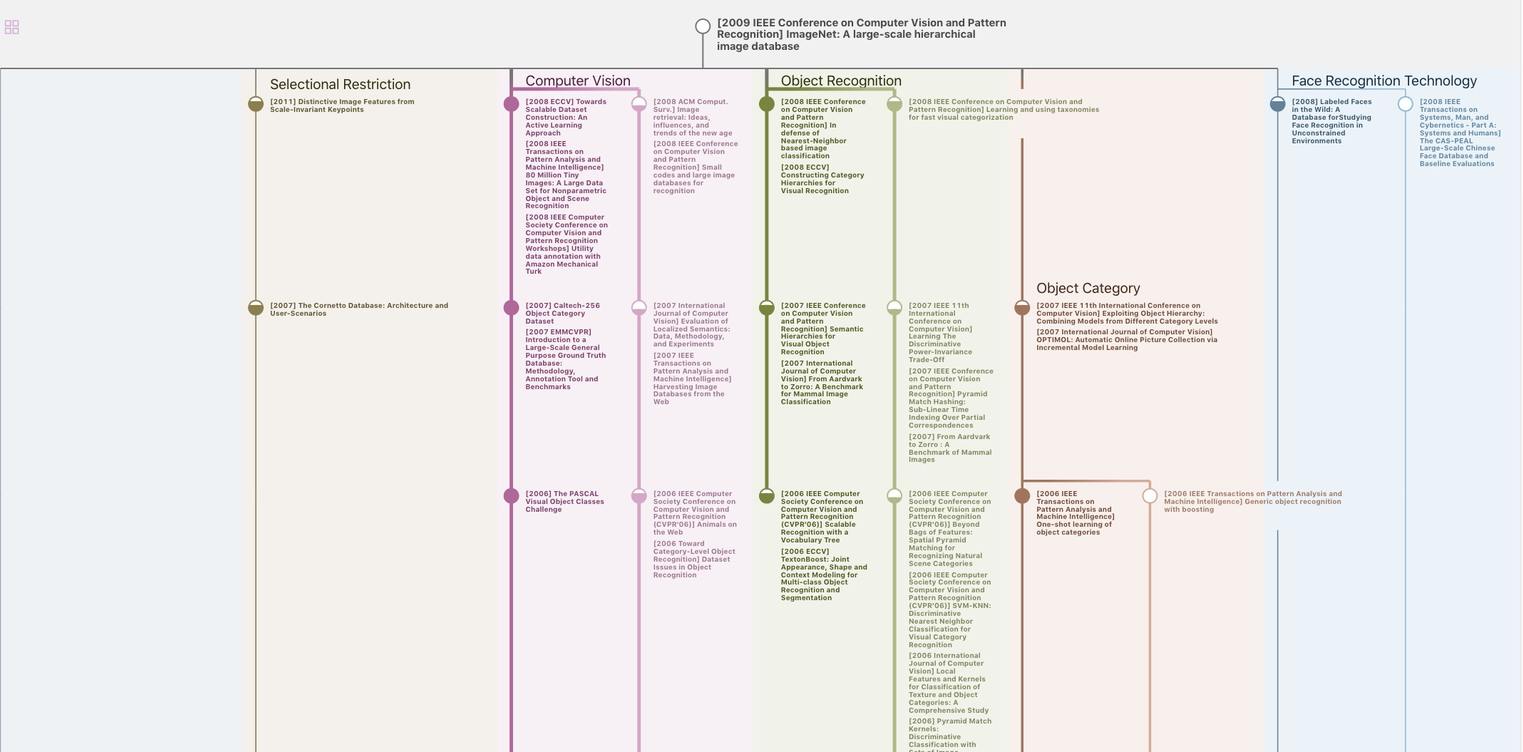
生成溯源树,研究论文发展脉络
Chat Paper
正在生成论文摘要