Knowledge Distillation Based Semi-supervised Hyperspectral Image Classification
ICBDT '23: Proceedings of the 2023 6th International Conference on Big Data Technologies(2023)
摘要
Deep learning based models have achieved great progress in hyperspectral image classification. However, the lack of labeled data increases over-fitting risk and decreases the performance of the model. To tackle this issue, we propose a semi-supervised architecture, KDSemi, for better exploring the unlabeled data in hyperspectral images. Specifically, we employ a segmentation model to assign labels to every pixel. Unlike classification models, the segmentation model exports both labeled and unlabeled pixels. The unlabeled ones are used to develop an implicit reconstruction loss to learn in a knowledge distillation manner. We evaluate our model on three popular datasets. Experiments verify that our KDSemi achieves competitive results with SOTAs.
更多查看译文
AI 理解论文
溯源树
样例
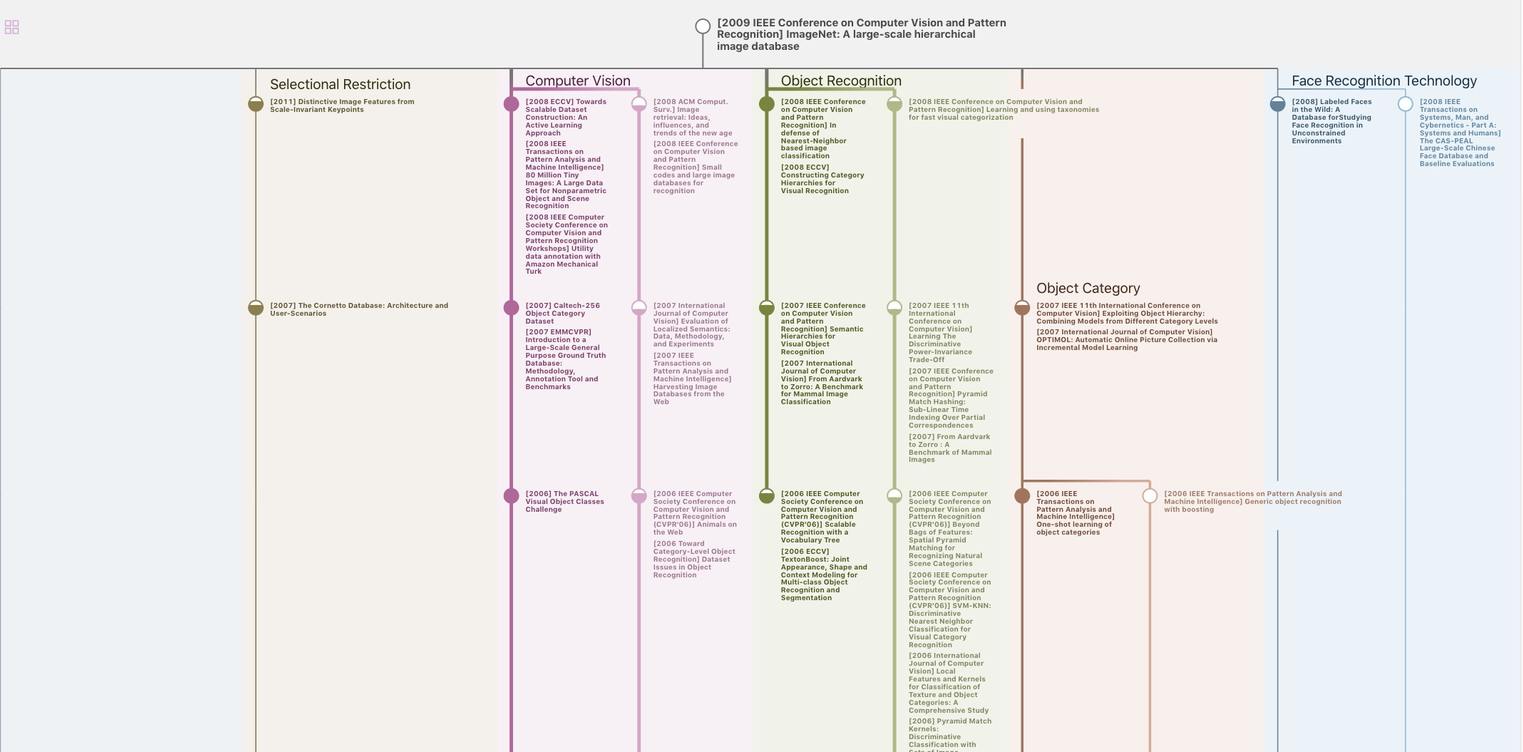
生成溯源树,研究论文发展脉络
Chat Paper
正在生成论文摘要