Scaling Whole-Chip QAOA for Higher-Order Ising Spin Glass Models on Heavy-Hex Graphs
CoRR(2023)
摘要
We show through numerical simulation that the Quantum Alternating Operator
Ansatz (QAOA) for higher-order, random-coefficient, heavy-hex compatible spin
glass Ising models has strong parameter concentration across problem sizes from
16 up to 127 qubits for p=1 up to p=5, which allows for
straight-forward transfer learning of QAOA angles on instance sizes where
exhaustive grid-search is prohibitive even for p>1. We use Matrix Product
State (MPS) simulation at different bond dimensions to obtain confidence in
these results, and we obtain the optimal solutions to these combinatorial
optimization problems using CPLEX. In order to assess the ability of current
noisy quantum hardware to exploit such parameter concentration, we execute
short-depth QAOA circuits (with a CNOT depth of 6 per p, resulting in
circuits which contain 1420 two qubit gates for 127 qubit p=5 QAOA) on
100 higher-order (cubic term) Ising models on IBM quantum superconducting
processors with 16, 27, 127 qubits using QAOA angles learned from a single
16-qubit instance. We show that (i) the best quantum processors generally
find lower energy solutions up to p=3 for 27 qubit systems and up to p=2
for 127 qubit systems and are overcome by noise at higher values of p, (ii)
the best quantum processors find mean energies that are about a factor of two
off from the noise-free numerical simulation results. Additional insights from
our experiments are that large performance differences exist among different
quantum processors even of the same generation and that dynamical decoupling
significantly improve performance for some, but decrease performance for other
quantum processors. Lastly we show p=1 QAOA angle mean energy landscapes
computed using up to a 414 qubit quantum computer, showing that the mean QAOA
energy landscapes remain very similar as the problem size changes.
更多查看译文
AI 理解论文
溯源树
样例
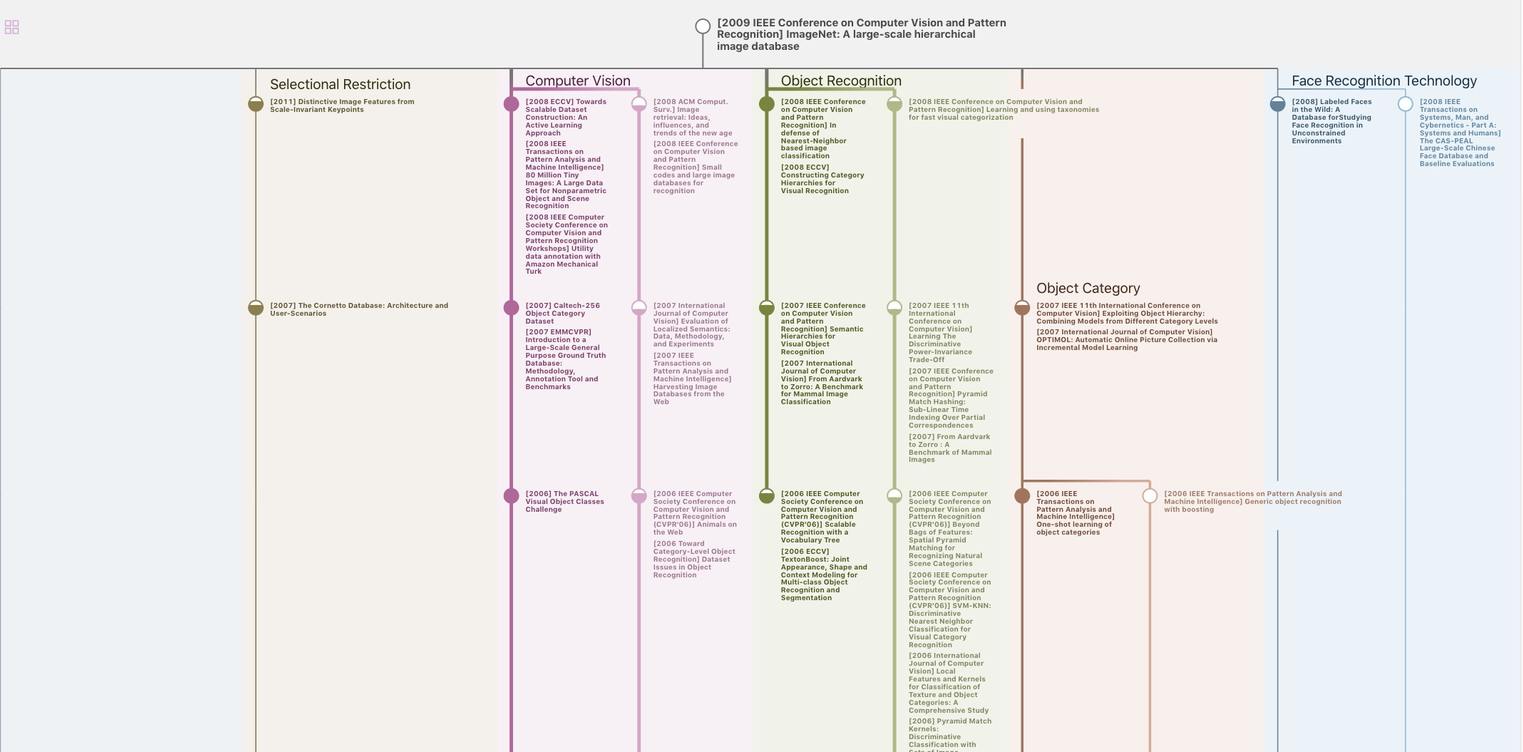
生成溯源树,研究论文发展脉络
Chat Paper
正在生成论文摘要