MEDS-Net: Multi-encoder based self-distilled network with bidirectional maximum intensity projections fusion for lung nodule detection
Engineering Applications of Artificial Intelligence(2024)
摘要
Early detection of lung cancer increases cure rates, making precise detection of lung nodules in computed tomography (CT) images crucial. However, creating an accurate computer-aided detection (CADe) system is challenging due to lung structure complexity and nodule heterogeneity. We present a lung nodule detection scheme, inspired by radiologist workflow, fusing CT scan sub-volumes with bidirectional maximum intensity projection (MIP) images within a single architecture. We have developed a novel multi-encoder-based network (MEDS-Net) using self-distillation to learn from three types of inputs: 3D sub-volume, forward, and backward MIP images. MEDS-Net utilizes three encoders to extract insights, passed to the decoder via attention units to suppress redundant features. The decoder employs a self-distillation mechanism, connecting to a distillation block with four auxiliary lung nodule detectors, accelerating convergence and enhancing learning ability. The auxiliary detectors are used during inference to reduce false positives. Our scheme, tested on 888 LIDC-IDRI dataset scans, achieved a 93.6% competition performance metric score, illustrating that fusing bidirectional MIP images with raw CT slices and auxiliary detectors empowers MEDS-Net to accurately detect lung nodules while minimizing false positives.
更多查看译文
关键词
Bidirectional maximum intensity projection,Computer-aided detection (CADe),Multi-encoder network,Pulmonary nodule detection,Self-distillation
AI 理解论文
溯源树
样例
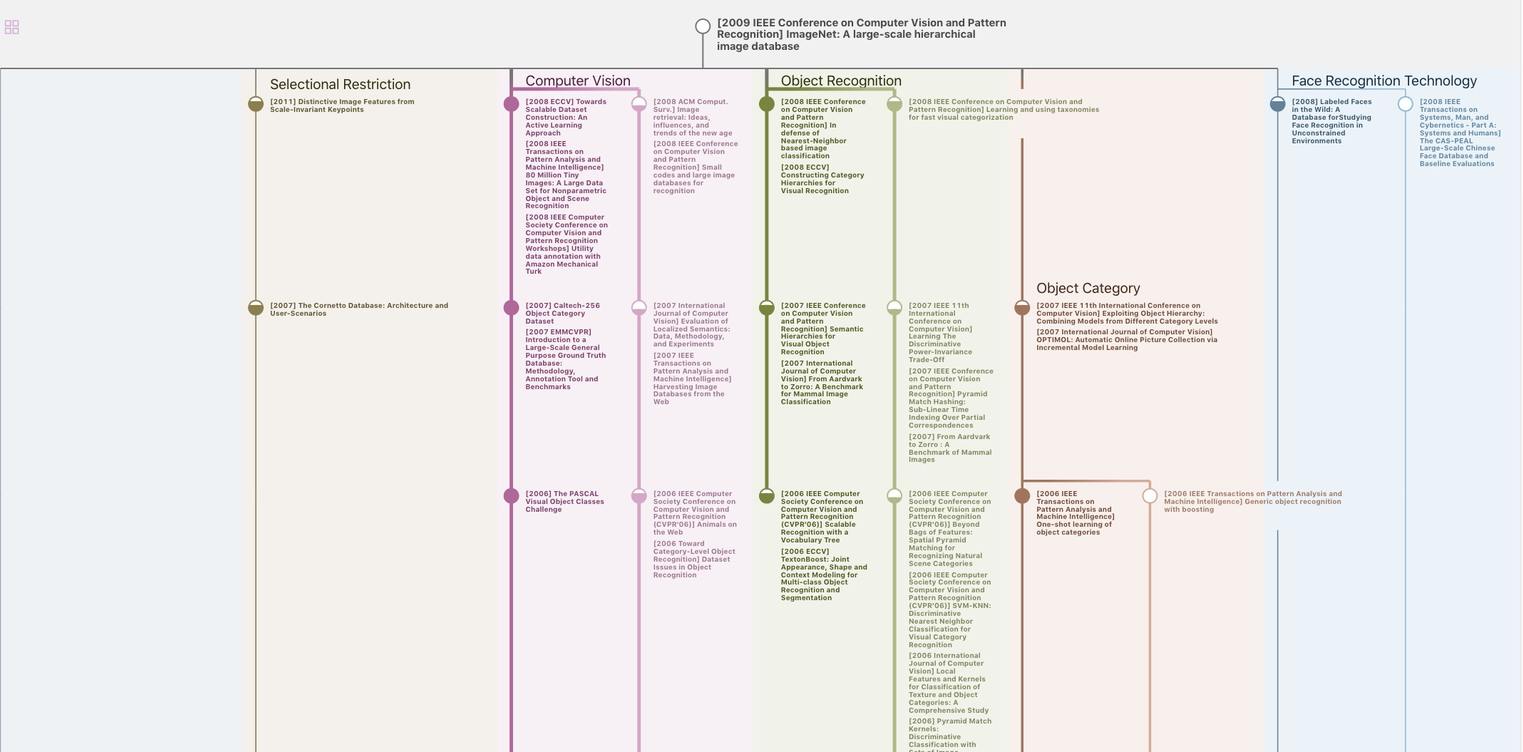
生成溯源树,研究论文发展脉络
Chat Paper
正在生成论文摘要