Knowledge evolution learning: A cost-free weakly supervised semantic segmentation framework for high-resolution land cover classification
ISPRS JOURNAL OF PHOTOGRAMMETRY AND REMOTE SENSING(2024)
摘要
Despite the success of deep learning in land cover classification, high-resolution (HR) land cover mapping remains challenging due to the time-consuming and labor-intensive process of collecting training samples. Many global land cover products (LCP) can reflect the low-level commonality (LLC) knowledge of land covers, such as basic shape and underlying semantic information. Therefore, we expect to use LCP as weakly supervised in-formation to guide the semantic segmentation of HR images. We regard high-level specialty (HLS) knowledge as HR information unavailable in the LCP. We believe LLC knowledge can gradually evolve into HLS knowledge through self-active learning. Hence, we design a knowledge evolution learning strategy from LLC to HLS knowledge and correspondingly devise a knowledge evolution weakly supervised learning framework (KE-WESUP) based on LCP. KE-WESUP mainly includes three tasks: (1) Abstraction of LLC knowledge. KE-WESUP first adopts a training method based on superpixel to alleviate the inconsistency between LCP and HR images and directly learns the LLC knowledge from LCP according to the feature-fitting capacity of convolutional neural networks. (2) Automatic exploration of HLS knowledge. We propose a dynamic label optimization strategy to obtain a small number of point labels with high confidence and encourage the model to automatically mine HLS knowledge through the knowledge exploration mechanism, which prompts the model to adapt to complexHR scenes. (3) Dynamic interaction of LLC and HLS knowledge. We adopt the consistency regularization method to achieve further optimization and verification of LLC and HLS knowledge. To verify the effectiveness of KE-WESUP, we conduct experiments on USDA National Agriculture Imagery Program (1 m) and GaoFen-2 (1 m) data using WolrdCover (10 m) as labels. The results show that KE-WESUP achieves outstanding results in both experiments, which has significant advantages over existing weakly supervised methods. Therefore, the proposed method has great potential in utilizing the prior information of LCP and is expected to become a new paradigm for large-scale HR land cover classification.
更多查看译文
关键词
Weakly supervised,Land cover product,Superpixel,Knowledge evolution,Consistency regularization
AI 理解论文
溯源树
样例
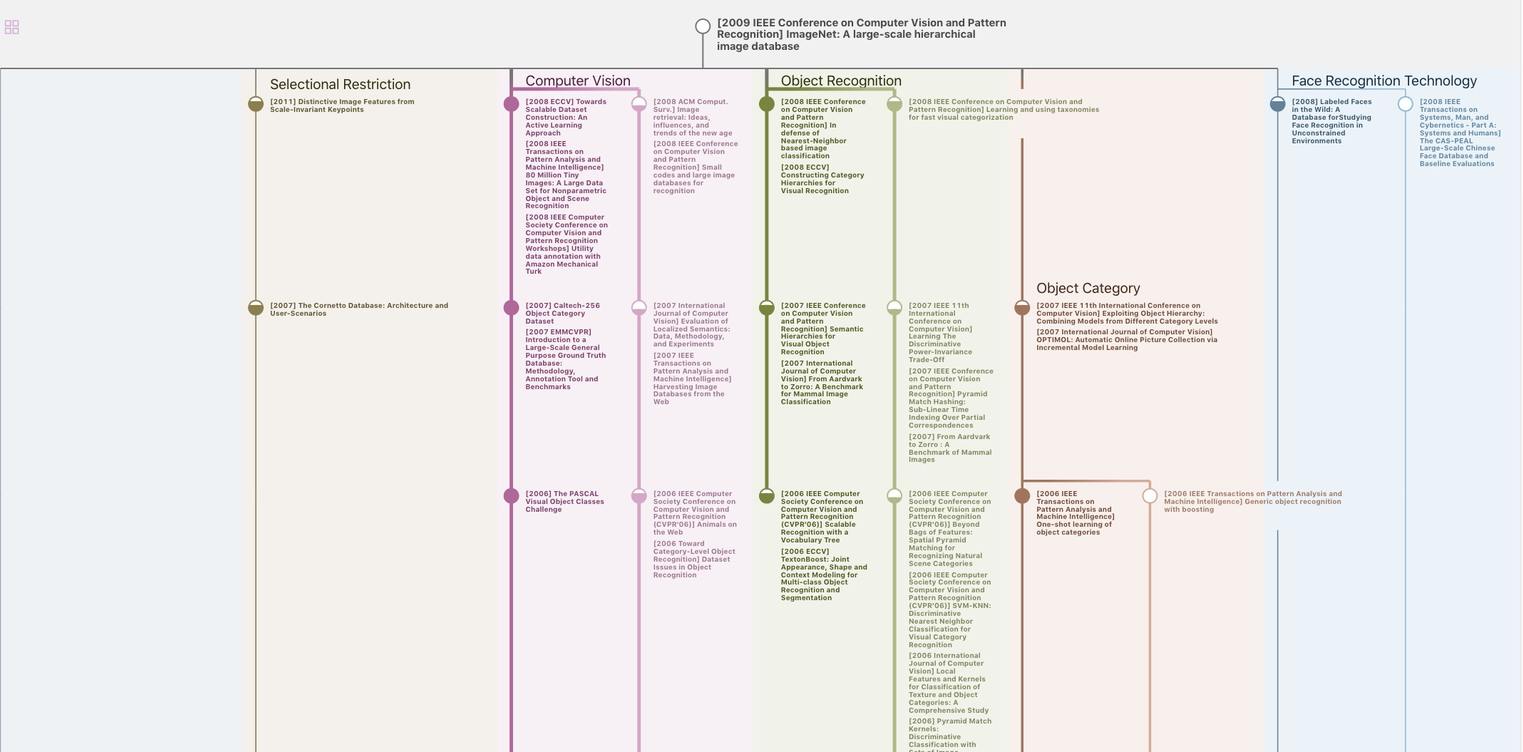
生成溯源树,研究论文发展脉络
Chat Paper
正在生成论文摘要