Reinforcement learning based multi-parameter joint optimization in dense multi-hop wireless networks
AD HOC NETWORKS(2024)
摘要
Carrier Sense Multiple Access with Collision Avoid (CSMA/CA) restricts the channel utilization efficiency although it always is regarded as a promising distributed channel access scheme, especially in dynamic dense wireless network scenarios. This paper investigates a Reinforcement Learning (RL) based multi-parameter joint optimization mechanism to build suitable access configuration for dense wireless multi-hop networks. Specifically, we propose a light-weight CSMA/CA mechanism based on Q-Learning (QL), namely QL-CSMA/CA, which jointly optimizes multiple channel access parameters, including Contention Window (CW), Maximum Backoff Exponent (MaxBE), and Maximum CSMA Backoffs (MaxCSMABackoffs), by setting mixed rewards and thus leading to higher network performance. Due to the limitation of the QL-CSMA/CA in large-scale state-space scenarios, we further present an enhanced CSMA/CA mechanism based on Deep Q-Network (DQN), namely DQN-CSMA/CA. Despite introducing a little complexity, the DQN-CSMA/CA can significantly improve transmission performance by utilizing more comprehensive network state information in complex dynamic network scenarios. Simulation results demonstrate the effectiveness of the proposed algorithms in terms of throughput, packet loss rate, delay, and collision rate, especially in high-density scenarios. Specifically, compared to SCAMA and traditional CSMA/CA algorithms, the QL-CSMA/CA algorithm achieves 9% and 5% performance improvements in terms of throughput, while DQN-CSMA/CA algorithm achieves 14% and 10% improvements in terms of throughput, respectively.
更多查看译文
关键词
Dense wireless multi-hop networks,CSMA/CA,Mixed rewards,Reinforcement learning
AI 理解论文
溯源树
样例
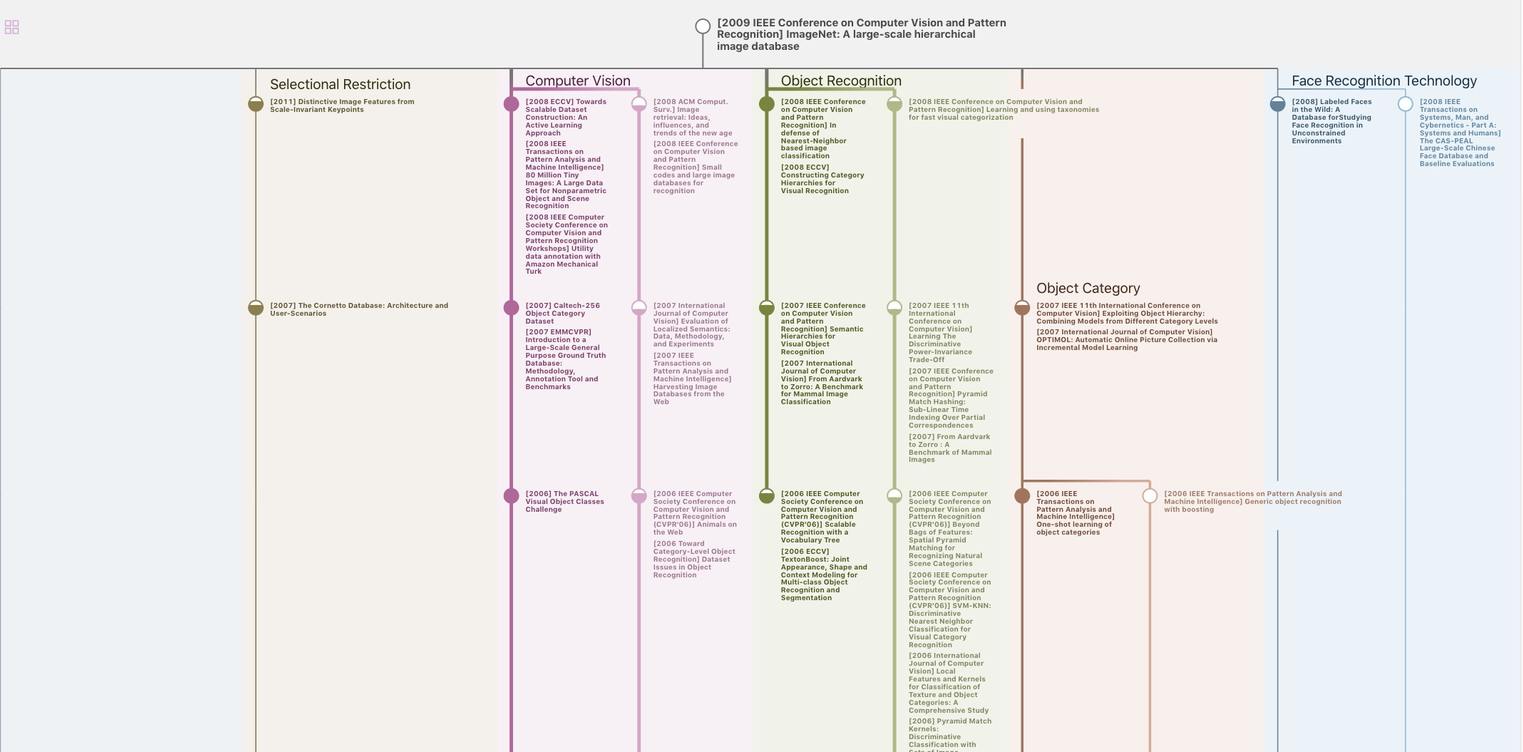
生成溯源树,研究论文发展脉络
Chat Paper
正在生成论文摘要