Exploring explainable machine learning and Shapley additive exPlanations (SHAP) technique to uncover key factors of HNSC cancer: An analysis of the best practices
BIOMEDICAL SIGNAL PROCESSING AND CONTROL(2024)
摘要
Objective: Head and Neck Squamous Cell Carcinoma (HNSC) is characterized by poor prognosis and easy recurrence. Aberrant induction of autophagy and long non-coding RNAs (LncRNAs) have emerged as crucial factors in diagnosing and predicting HNSC. The goal of this study is to show how machine learning may help categorize HNSC subjects into risk categories. Moreover, we used SHAP architectures to offer explanations and interpretations. Methods: In this study, we screened autophagy-related LncRNAs (DEARLs) using differential analysis and co-expression network analysis, identified DEARLs with prognostic ability using LASSO regression and RF classification, built a model based on multivariate Cox regression, subsequently validated its predictive capability. Eight machine learning methods were used to construct prediction models. SHAP analysis was established based on the best model, and the output of the ARLGS model was interpreted to filter out significant DEARLs. Finally, the ARLGS model was used for pan-cancer analysis.Results: We determined Nine DEARLs with predictive ability, and then these DEARLs were utilized to construct an ARLGS model. Kaplan-Meier (KM) analysis was performed to verify that cohorts with high ARLGS exhibited lower overall survival (OS) compared to those with low ARLGS, both in the train and test set. Furthermore, ROC curve analysis demonstrated the model's strong performance for HNSC patients at 1-, 3-, and 5-year (AUC > 70 %). Comparing the prediction models established by eight machine learning methods, we found that the xgboost model has the highest accuracy. Based on this, we performed SHAP analysis and found that the five DEARLs with the highest SHAP values were MIAT, LINC01343, LINC01305, and SLCO4A1.AS1 and GATA2.AS1 reflects the importance of these five DEARLs. Finally, we obtained the differential expression, immune signature, mutation, and drug sensitivity signatures of DEARLs in 33 cancers using pan-cancer analysis.Conclusion: We developed an ARLGS model with good reliability using LASSO and RF. We also found that the Xgboost model was the best, and can be used for SHAP analysis to find important DEARLs, solving the problem of the uninterpretable nature of machine learning methods. Thus, such an approach provided important guidance for assessing the risk of HNSC patients and developing customized treatments.
更多查看译文
关键词
HNSC,Autophagy genes,LncRNAs,LASSO,RF,SHAP
AI 理解论文
溯源树
样例
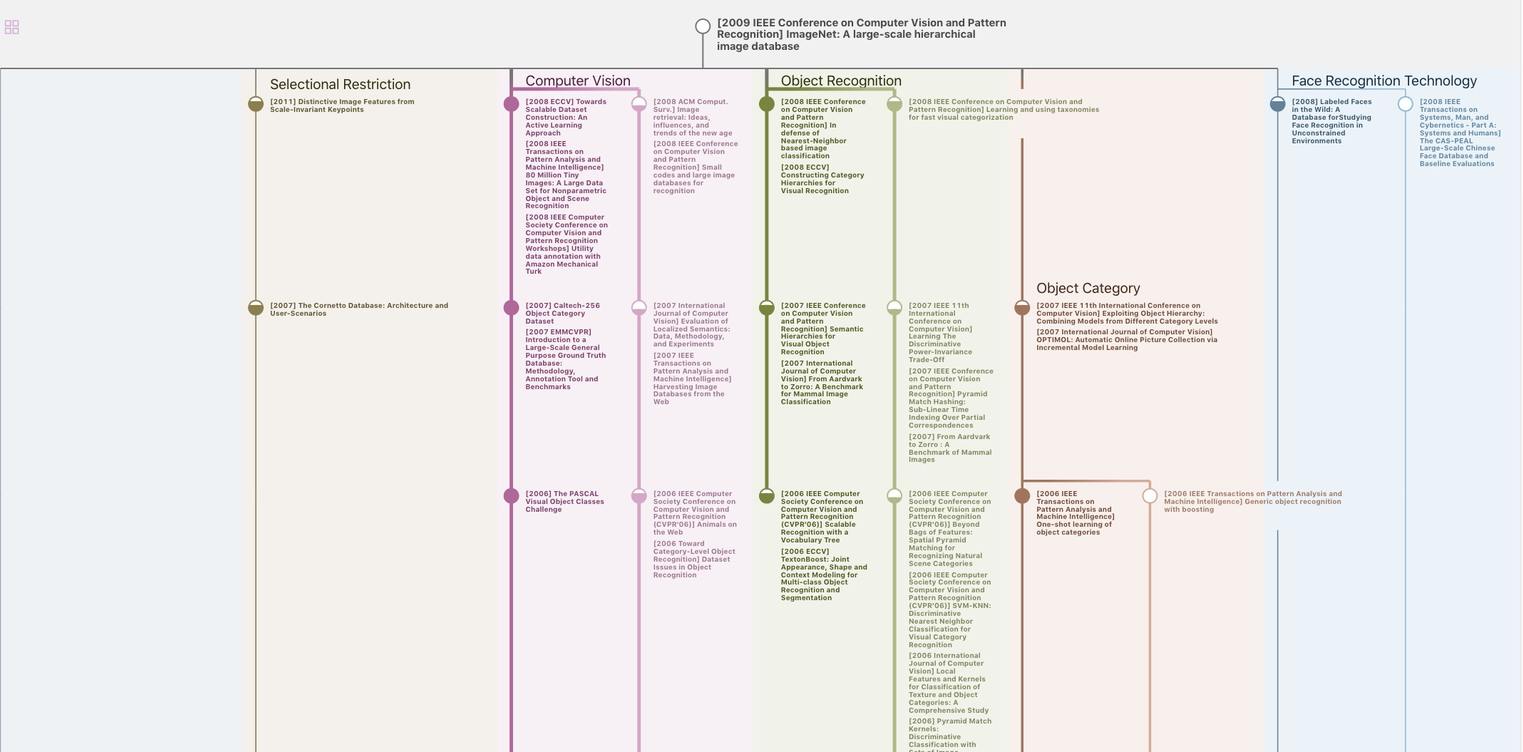
生成溯源树,研究论文发展脉络
Chat Paper
正在生成论文摘要