Quick taxi route assignment via real-time intersection state prediction with a spatial-temporal graph neural network
TRANSPORTATION RESEARCH PART C-EMERGING TECHNOLOGIES(2024)
摘要
The optimization of airport taxi routes is crucial for increasing airport capacity and resource utilization. However, existing methods for preplanning schedules are often time-consuming. To address this, we propose a new approach that reduces the problem scale by predicting the intersection state in the airport taxiway network using a spatial-temporal graph neural network (STGNN) that incorporates a graph convolutional network and recursive neural network with an attention mechanism. A mixed-integer linear problem (MILP) model for taxi route assignment is established based on a subgraph obtained from the prediction results. Our approach treats time as a continuous variable without discretization, allowing aircraft to stop and wait at any node to avoid conflict. Finally, we demonstrate the effectiveness of our approach by testing it on actual operation data from Beijing Capital International Airport (PEK). Compared with several baseline algorithms, the method developed in this study has a significant impact on improving airport transportation efficiency. The results indicate that our method not only produces high-quality solutions but also operates in real time.
更多查看译文
关键词
Taxi route scheduling,Ground support services control,GCN,MILP,Real-time
AI 理解论文
溯源树
样例
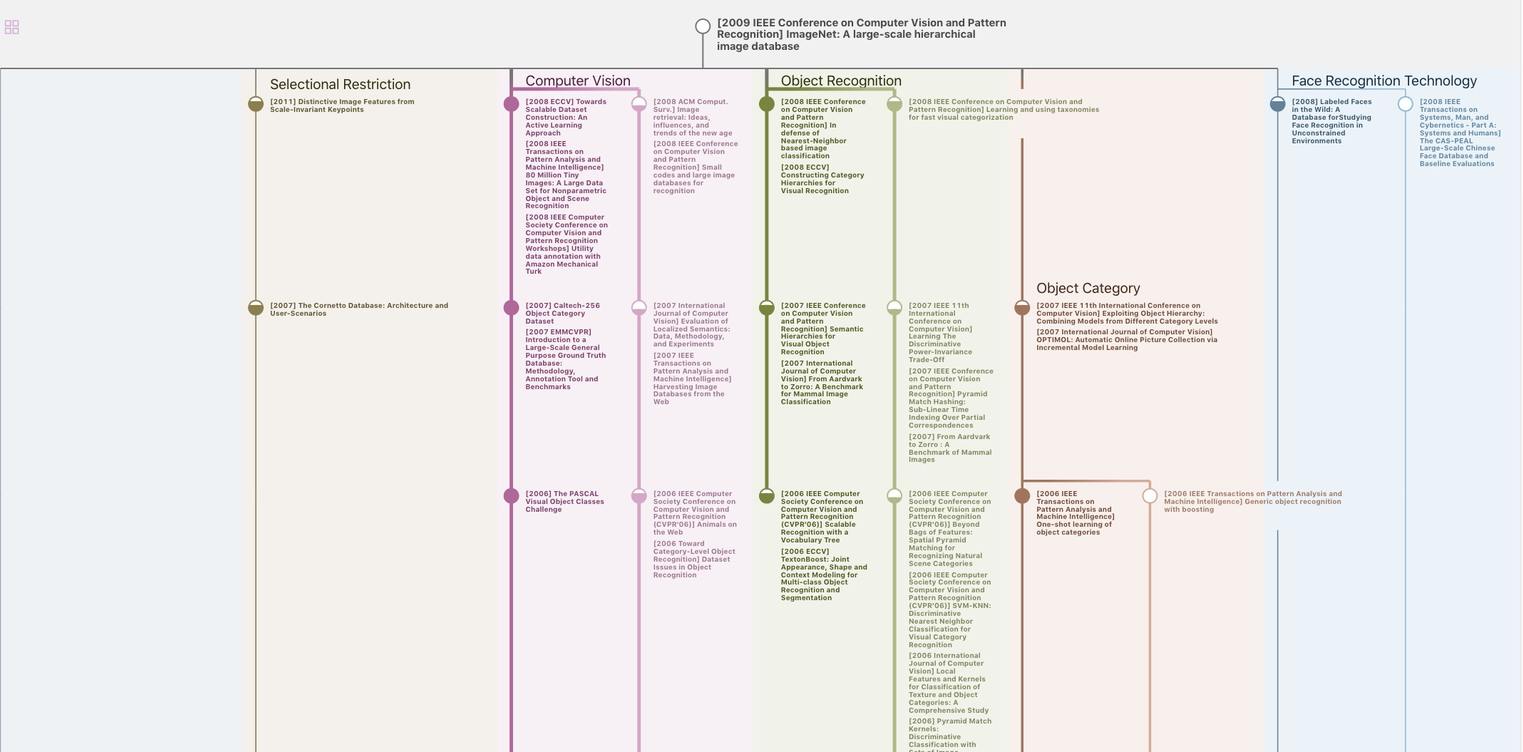
生成溯源树,研究论文发展脉络
Chat Paper
正在生成论文摘要