Dual ensemble approach to predict rice heading date by integrating multiple rice phenology models and machine learning-based genetic parameter regression models
AGRICULTURAL AND FOREST METEOROLOGY(2024)
摘要
Recent research advances in crop breeding and bioinformatics have revealed several genes that determine the flowering (heading) time in some crops. In this study, we calibrated an integrated rice phenology model that uses the genetic information of the cultivar to estimate the parameters of the models. Differences in the prediction of heading stage were examined using different phenology models and parameter estimation methods. We also proposed a novel approach to construct a dual-integrated ensemble model using both multiple crop phenology and regression models to estimate their parameters based on the haplotype combinations of 11 genes related to the heading date of rice. We used the data of 144 Japanese rice (Oryza sativa L.) cultivars to compare the prediction accuracy of the proposed approach with that of individual integrated phenology models. Subsequently, 4,083 ensemble patterns were analyzed using four rice phenology models (Beta, simplified Beta, ORYZA2000, and SIMIRIW) and three machine learning-based parameter regression methods (support vector, random forest, and ridge regression). The accuracy of the individual integrated model depended on the phenology model and its parameter regression method used. In some cases, the RMSE differed by more than one day. When the individual integrated model with the highest prediction accuracy in the training data was applied to the known and unknown environmental test data sets, the prediction accuracies were about 7.0 d and 8.2 d, respectively in the cross-validation. The prediction accuracy of the dual ensemble model with five ensemble members was approximately 5.9 d and 6.5 d in known and unknown environments. Our results suggest that the dual ensemble approach can enhance crop phenology prediction accuracy and present the importance of ensemble parameterization as well as combining multiple crop models when integrating genomic prediction with crop phenology models.
更多查看译文
关键词
Japan,Ensemble model,Genomic prediction,Heading/flowering-related gene,Integrated crop model,Parameterization,Genetic information,Parameter uncertainty
AI 理解论文
溯源树
样例
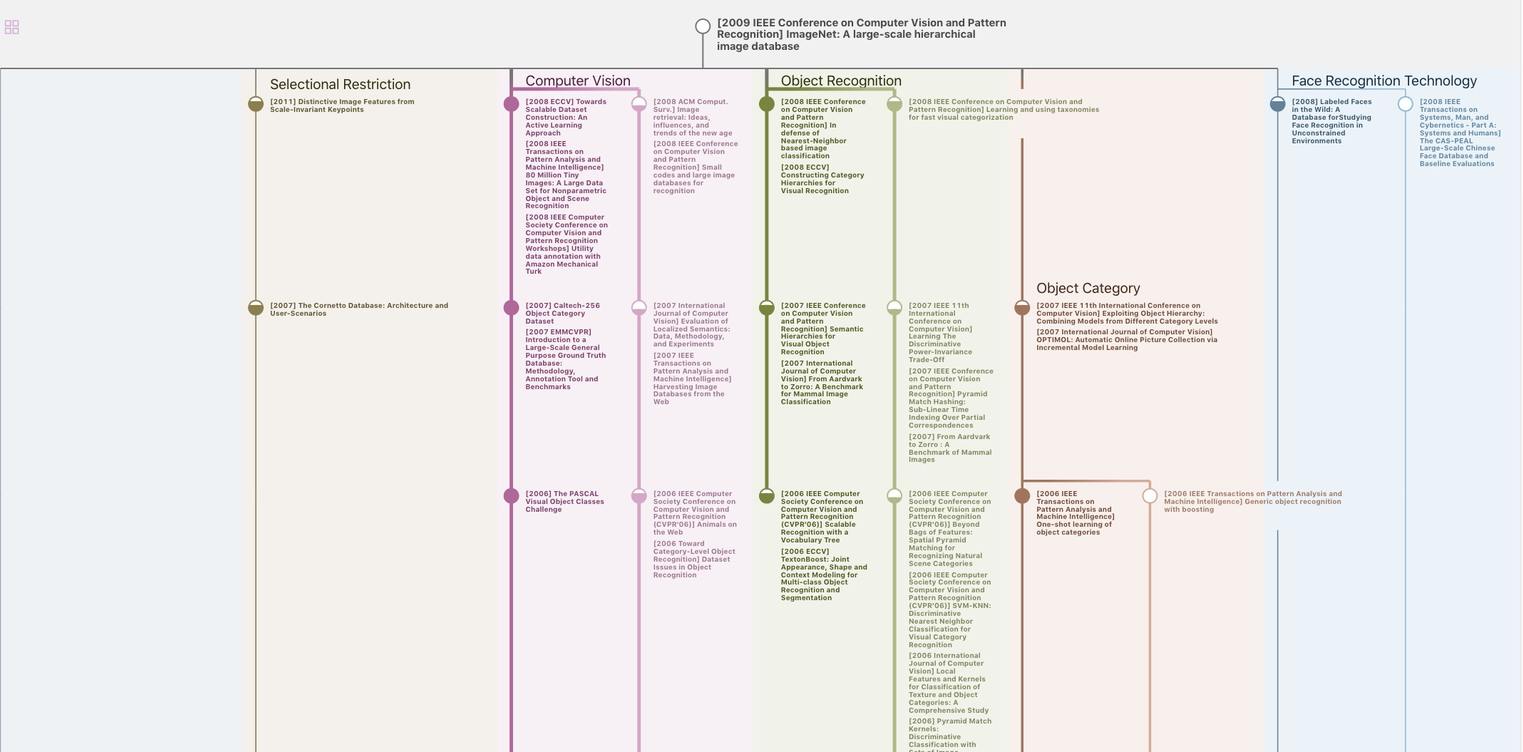
生成溯源树,研究论文发展脉络
Chat Paper
正在生成论文摘要