Sea surface reconstruction from marine radar images using deep convolutional neural networks
JOURNAL OF OCEAN ENGINEERING AND SCIENCE(2023)
摘要
The sea surface reconstructed from radar images provides valuable information for marine operations and maritime transport. The standard reconstruction method relies on the three-dimensional fast Fourier transform (3D-FFT), which introduces empirical parameters and modulation transfer function (MTF) to correct the modulation effects that may cause errors. In light of the convolutional neural networks' (CNN) success in computer vision tasks, this paper proposes a novel sea surface reconstruction method from marine radar images based on an end-to-end CNN model with the U-Net architecture. Synthetic radar images and sea surface elevation maps were used for training and testing. Compared to the standard reconstruction method, the CNN-based model achieved higher accuracy on the same data set, with an improved correlation coefficient between reconstructed and actual wave fields of up to 0.96-0.97, and a decreased non-dimensional root mean square error (NDRMSE) of around 0.06. The influence of training data on the deep learning model was also studied. Additionally, the impact of the significant wave height and peak period on the CNN model's accuracy was investigated. It has been demonstrated that the ac-curacy will fluctuate as the wave steepness increases, but the correlation coefficient remains above 0.90, and the NDRMSE remains less than 0.11.(c) 2023 Shanghai Jiaotong University. Published by Elsevier B.V.This is an open access article under the CC BY-NC-ND license ( http://creativecommons.org/licenses/by-nc-nd/4.0/ )
更多查看译文
关键词
Sea surface reconstruction,Radar image,CNN model
AI 理解论文
溯源树
样例
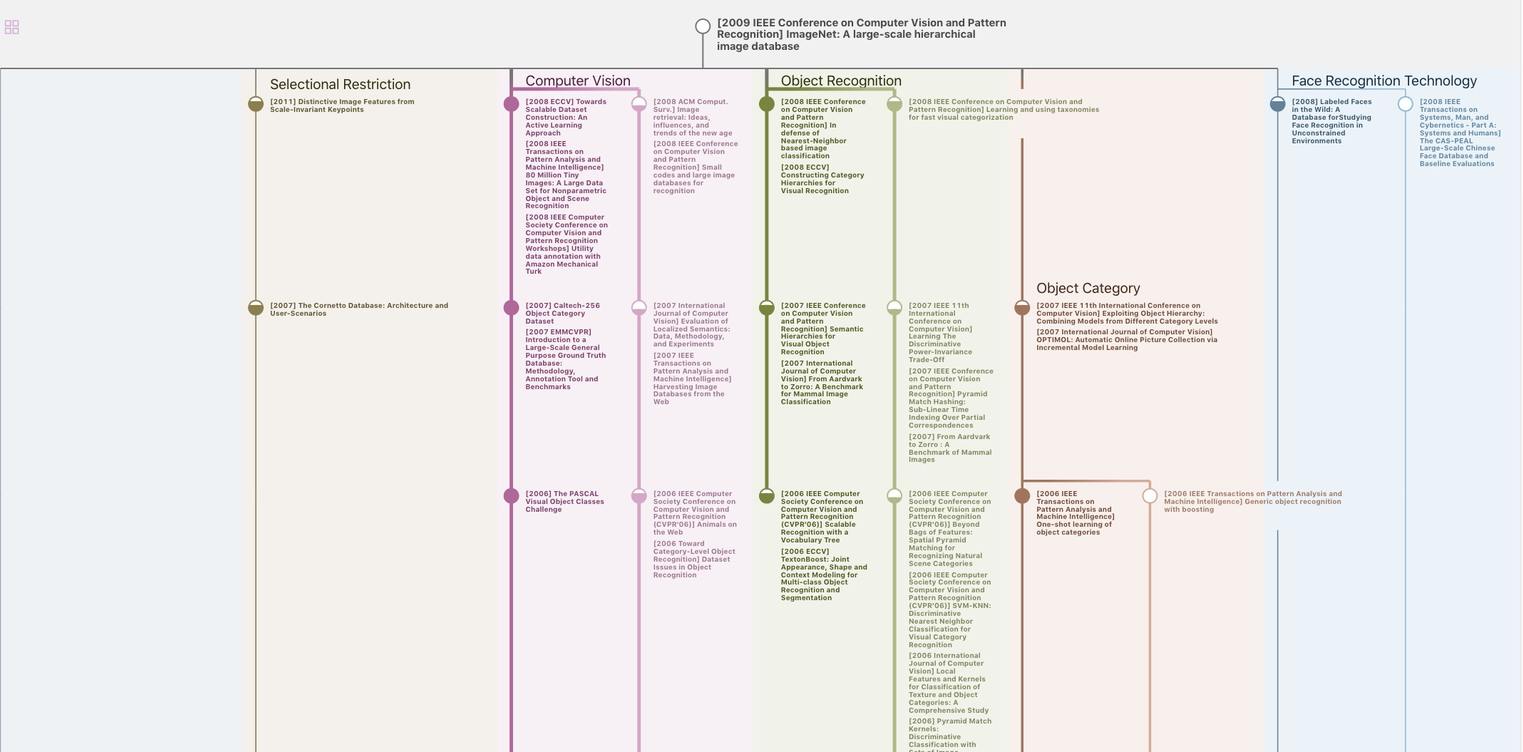
生成溯源树,研究论文发展脉络
Chat Paper
正在生成论文摘要