Photo-based Carbohydrates Counting using Pre-trained Transformer Models
IFAC-PapersOnLine(2023)
摘要
Type 1 diabetes is a severe form of diabetes that involves inadequate insulin production by the pancreas. Therefore, patients must take adequate amounts of external insulin to balance blood glucose, where the amount of carbohydrate ingested is the major factor in calculating insulin doses correctly. However, calculating the carbohydrate content of a meal can be complicated by several factors, including patient inexperience. In this work, we propose to devise a system for automatically estimating carbohydrates from images of plated meals. The aim is to reduce patients’ computational burden and to reduce the miss-estimates associated with traditional carbohydrate counting methods. The proposal introduces the generation of estimation models for carbohydrate counting using transformer-based neural networks by casting pre-trained models in a more general image context. The models are retrained using the Nutrition5k database, which contains a large diversity of meal samples with multiple examples, a wide range of carbohydrate amounts, and various ingredients. This study presents a detailed analysis of the performance of the implemented models, as well as how the meal composition and size can undermine the estimation models. The metrics show promising results, achieving a 23% error reduction over previous studies.
更多查看译文
关键词
Machine learning,carbohydrate estimation,transformer neural network,diabetes type 1,blood glucose
AI 理解论文
溯源树
样例
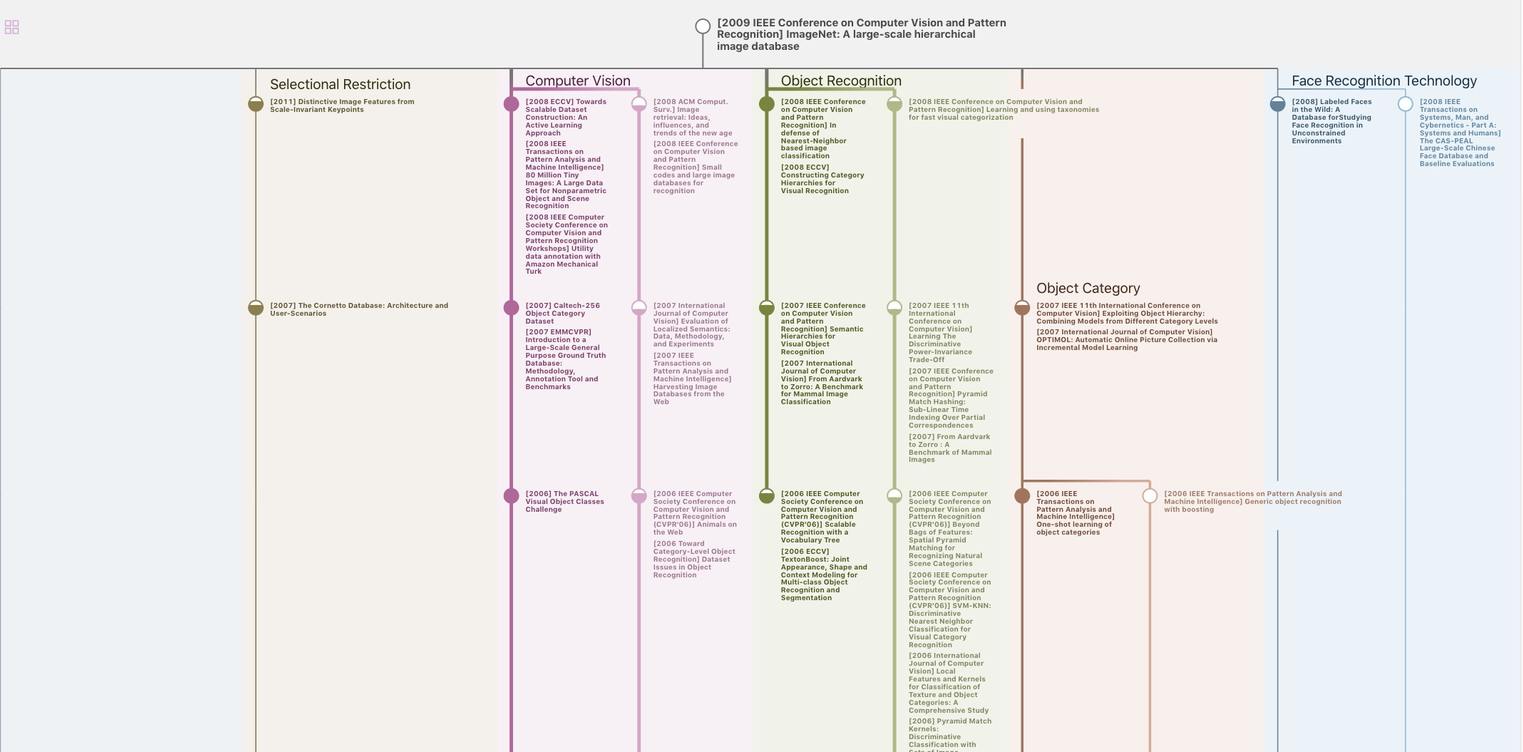
生成溯源树,研究论文发展脉络
Chat Paper
正在生成论文摘要