An Improved YOLOv7-based Detection of Tire Defects for Driving Assistance
IFAC PAPERSONLINE(2023)
摘要
Tire safety is critical to driverless driving safety, and tire defect detection facilitates the enhancement of Advanced Driver Assistance Systems. Considering the current characteristics of timeconsuming and labor-intensive manual detection, different criteria, tire pattern interference and diversity of defects, a tire defect detection approach project on Convolutional Block Attention Module (CBAM) and Adaptively Spatial Feature Fusion (ASFF) is proposed. First and foremost, combined with the dual attention mechanism of space and channel, the CBAM is introduced to obtain more comprehensive feature information. Secondly, combined with the thought of Depthwise Separable Convolution lightweight, the number of parameters is decreased to satisfy the application demands of high speed and high precision for tire detection. Finally, ASFF is introduced after the PANet of the model to combine the reserved feature maps of different depth levels to increase the scale invariance of the defect detection model, thereby resolve the matter of difficult detection due to the different size of tire defects. The experimental results state clearly that the scheme can precisely classify and locate tire defects, and the average precision of the test can reach 94.56%, which can effectively detect tire defects. Copyright (c) 2023 The Authors. This is an open access article under the CC BY-NC-ND license (https://creativecommons.org/licenses/by-nc-nd/4.0/)
更多查看译文
关键词
Tire,defect detection,Driving Assistance,CBAM,ASFF
AI 理解论文
溯源树
样例
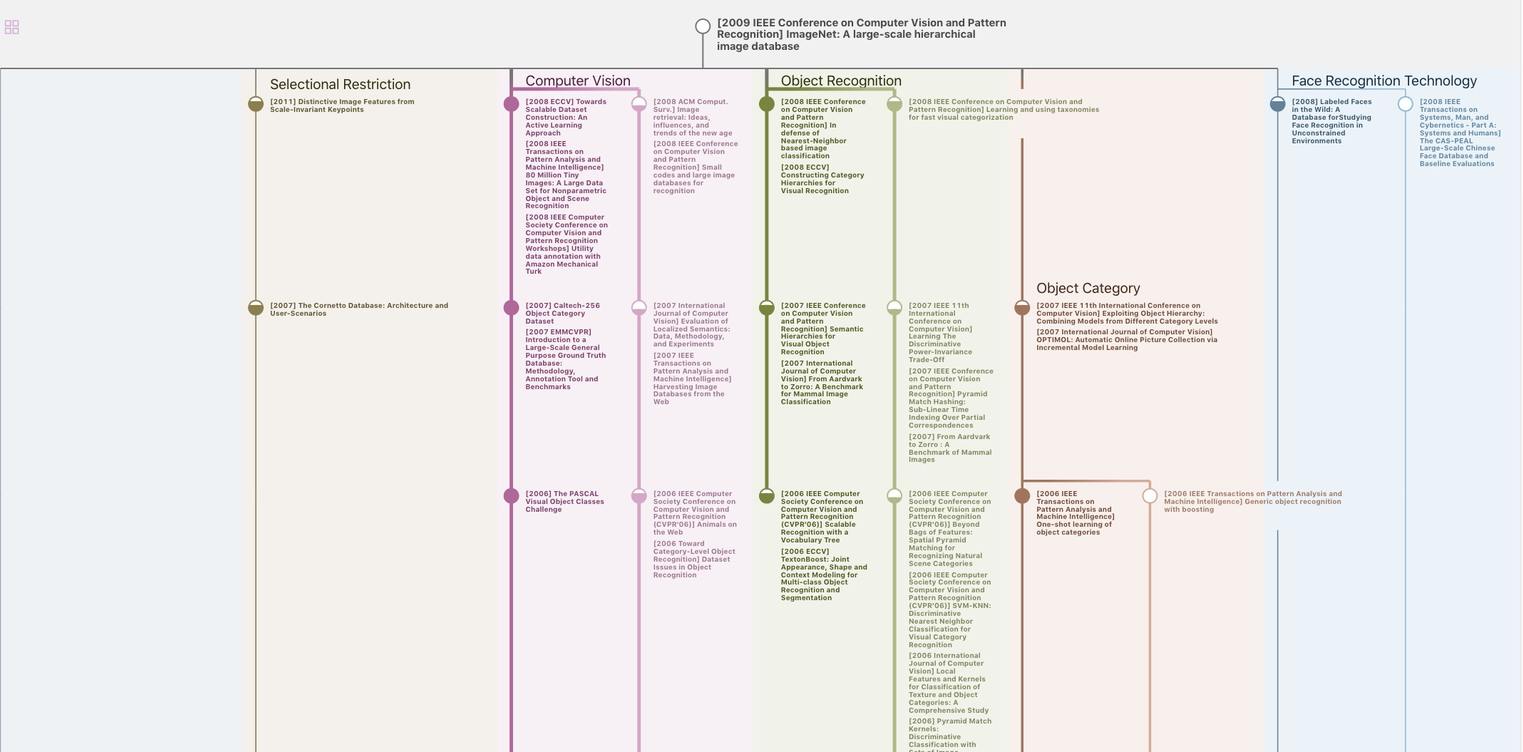
生成溯源树,研究论文发展脉络
Chat Paper
正在生成论文摘要