Identifying Novel Defects during AI-driven Visual Quality Inspection
IFAC PAPERSONLINE(2023)
摘要
Visual Quality Inspection is an integral part of the manufacturing process, becoming increasingly automated with the advent of Industry 4.0. While modern industries reap substantial benefits from using AI-driven Computer Vision Algorithms and Deep Neural Networks, these technologies bring about new issues, such as the requirement for extensive training datasets and the lack of robustness to out-of-distribution samples. This can be particularly risky in real-world autonomous applications such as cyber-physical reinforcement learning systems where a lack of resilience to unexpected inputs could compromise the integrity of the production process or put human operators at risk. Our research explicitly examines scenarios of handling unanticipated defect types during the continuous operation of Visual Quality Inspection systems. To this end, many modern Machine Learning methods can be employed, such as open- set recognition, semi-supervised novelty detection, and intelligent data augmentation. We examine a range of proven and state-of-the-art methods focusing on Deep Learning over a real-world dataset. Our comparative analysis highlights significant trade-offs between approaches and provides concrete suggestions for immediate implementation and further research toward robust Visual Quality Inspection.
更多查看译文
关键词
Manufacturing Plant Control,Industry 4.0,Smart Manufacturing,Visual Quality Inspection,Deep Learning,Open-set Recognition,Novelty Detection
AI 理解论文
溯源树
样例
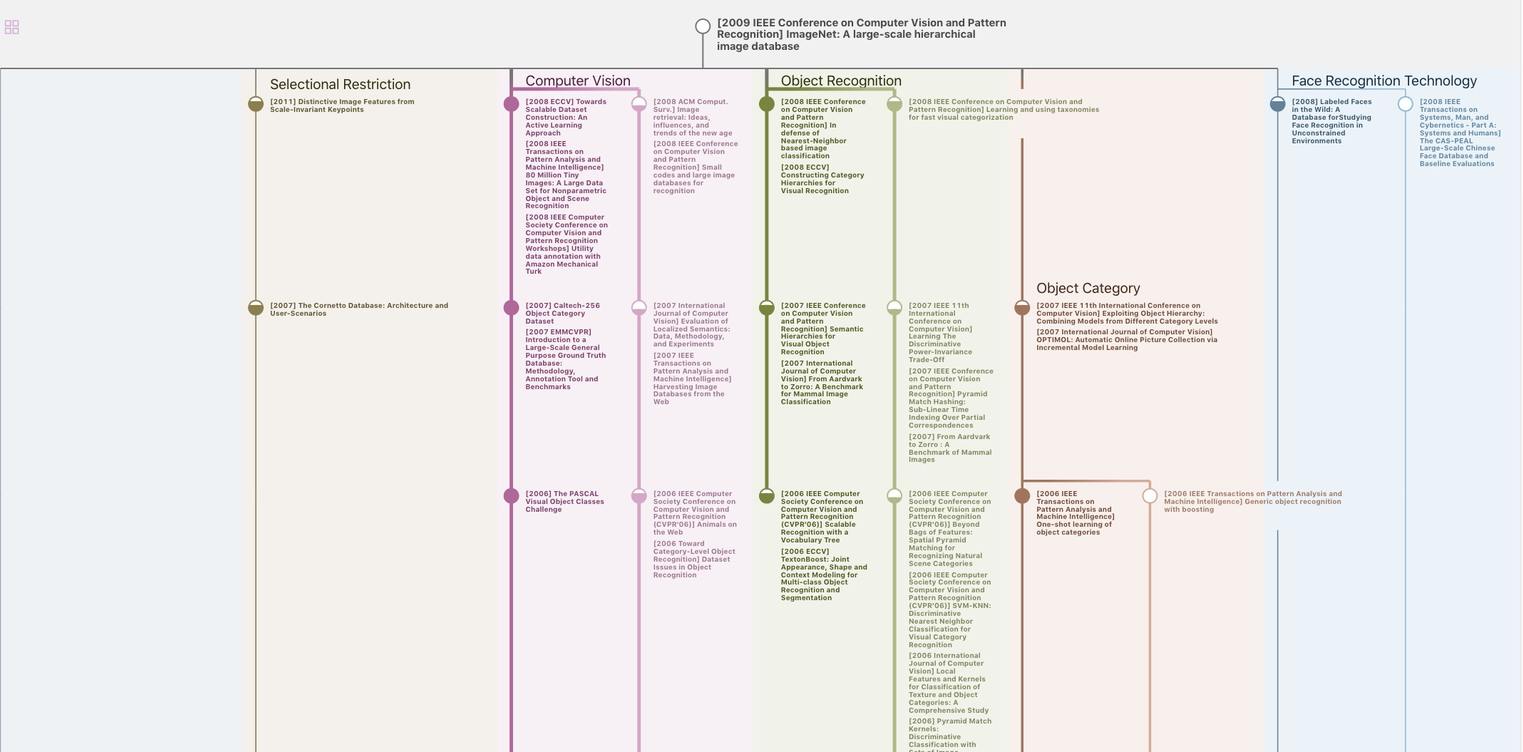
生成溯源树,研究论文发展脉络
Chat Paper
正在生成论文摘要