Pulmonary response prediction through personalized basis functions in a virtual patient model
COMPUTER METHODS AND PROGRAMS IN BIOMEDICINE(2024)
摘要
Background and Objective: Recruitment maneuvers with subsequent positive -end -expiratory -pressure (PEEP) have proven effective in recruiting lung volume and preventing alveoli collapse. However, determining a safe, effective, and patient -specific PEEP is not standardized, and this more optimal PEEP level evolves with patient condition, requiring personalised monitoring and care approaches to maintain optimal ventilation settings. Methods: This research examines 3 physiologically relevant basis function sets (exponential, parabolic, cumulative) to enable better prediction of elastance evolution for a virtual patient or digital twin model of MV lung mechanics, including novel elements to model and predict distension elastance. Prediction accuracy and robustness are validated against recruitment maneuver data from 18 volume -controlled ventilation (VCV) patients at 7 different baseline PEEP levels (0 to 12 cmH2O) and 14 pressure -controlled ventilation (PCV) patients at 4 different baseline PEEP levels (6 to 12 cmH2O), yielding 623 and 294 prediction cases, respectively. Predictions were made up to 12 cmH2O of added PEEP ahead, covering 6 x 2 cmH2O PEEP steps. Results: The 3 basis function sets yield median absolute peak inspiratory pressure (PIP) prediction error of 1.63 cmH2O for VCV patients, and median peak inspiratory volume (PIV) prediction error of 0.028 L for PCV patients. The exponential basis function set yields a better trade-off of overall performance across VCV and PCV prediction than parabolic and cumulative basis function sets from other studies. Comparing predicted and clinically measured distension prediction in VCV demonstrated consistent, robust high accuracy with R2 = 0.90-0.95. Conclusions: The results demonstrate recruitment mechanics are best captured by an exponential basis function across different mechanical ventilation modes, matching physiological expectations, and accurately capture, for the first time, distension mechanics to within 5-10 % accuracy. Enabling the risk of lung injury to be predicted before changing ventilator settings. The overall outcomes significantly extend and more fully validate this digital twin or virtual mechanical ventilation patient model.
更多查看译文
关键词
Virtual patient,Digital twin,Mechanical ventilation,Critical care,Basis function,Prediction,Elastance,Dynamic functional residual capacity,Lung distension,VILI,Pressure-volume loop
AI 理解论文
溯源树
样例
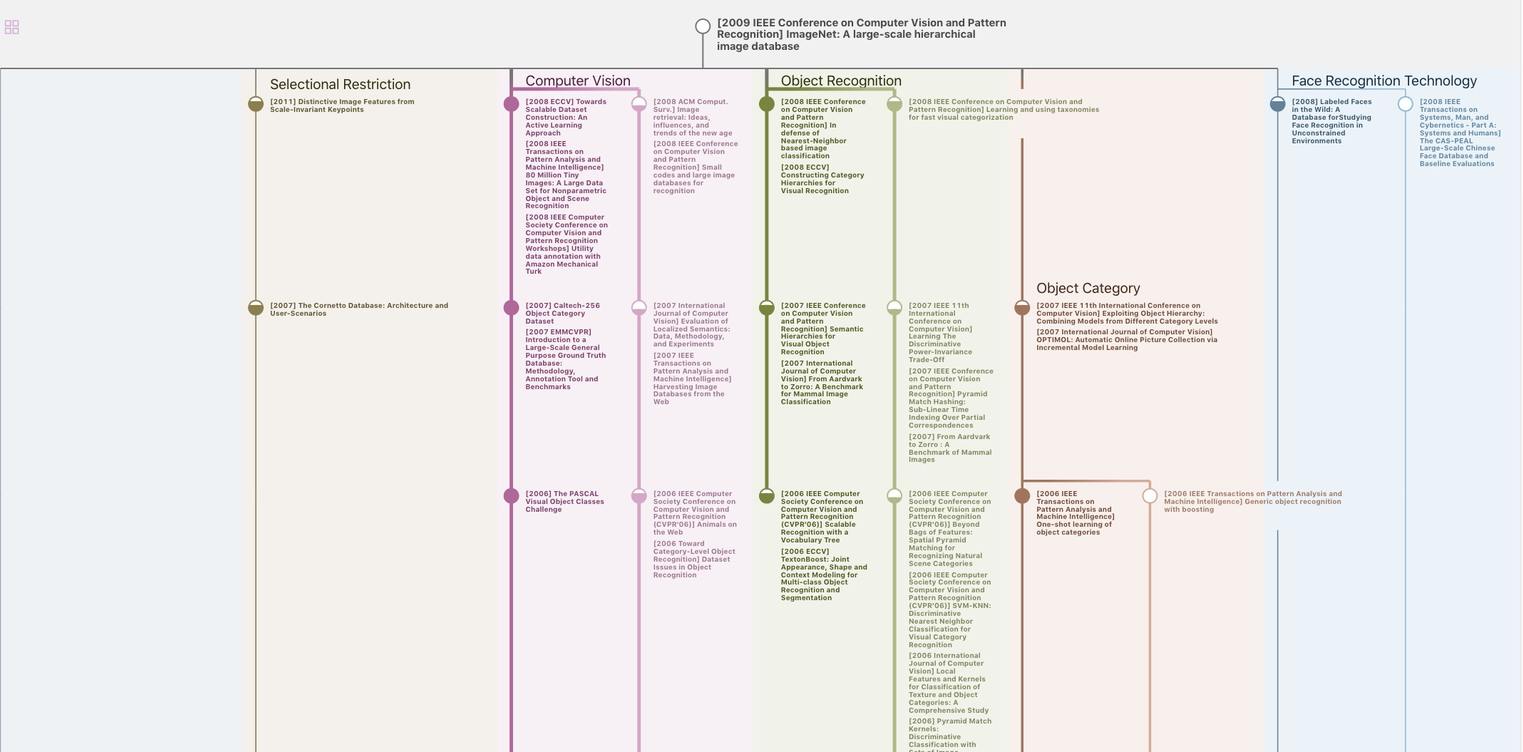
生成溯源树,研究论文发展脉络
Chat Paper
正在生成论文摘要