Fault diagnosis using data-driven residuals for anomaly classification with incomplete training data
IFAC PAPERSONLINE(2023)
摘要
Data-driven modeling and machine learning have received a lot of attention in fault diagnosis and system monitoring research. Since faults are rare events, conventional multi-class classification is complicated by incomplete training data and unknown faults. One solution is anomaly classification which can be used to detect abnormal behavior when only training data from the nominal operation is available. However, data-driven fault isolation is still a non-trivial task when training data is not representative of nominal and faulty behavior. In this work, the importance of redundancy for a set of known variables that are fed to a data-driven anomaly classification is discussed. It is shown that residual-based anomaly detection can be used to reject the nominal class which is not possible with one-class classifiers, such as one-class support vector machines. Based on these results, it is also discussed how data-driven residuals can be integrated with model-based fault isolation logic.
更多查看译文
关键词
Fault detection and isolation,Anomaly detection,Machine learning.
AI 理解论文
溯源树
样例
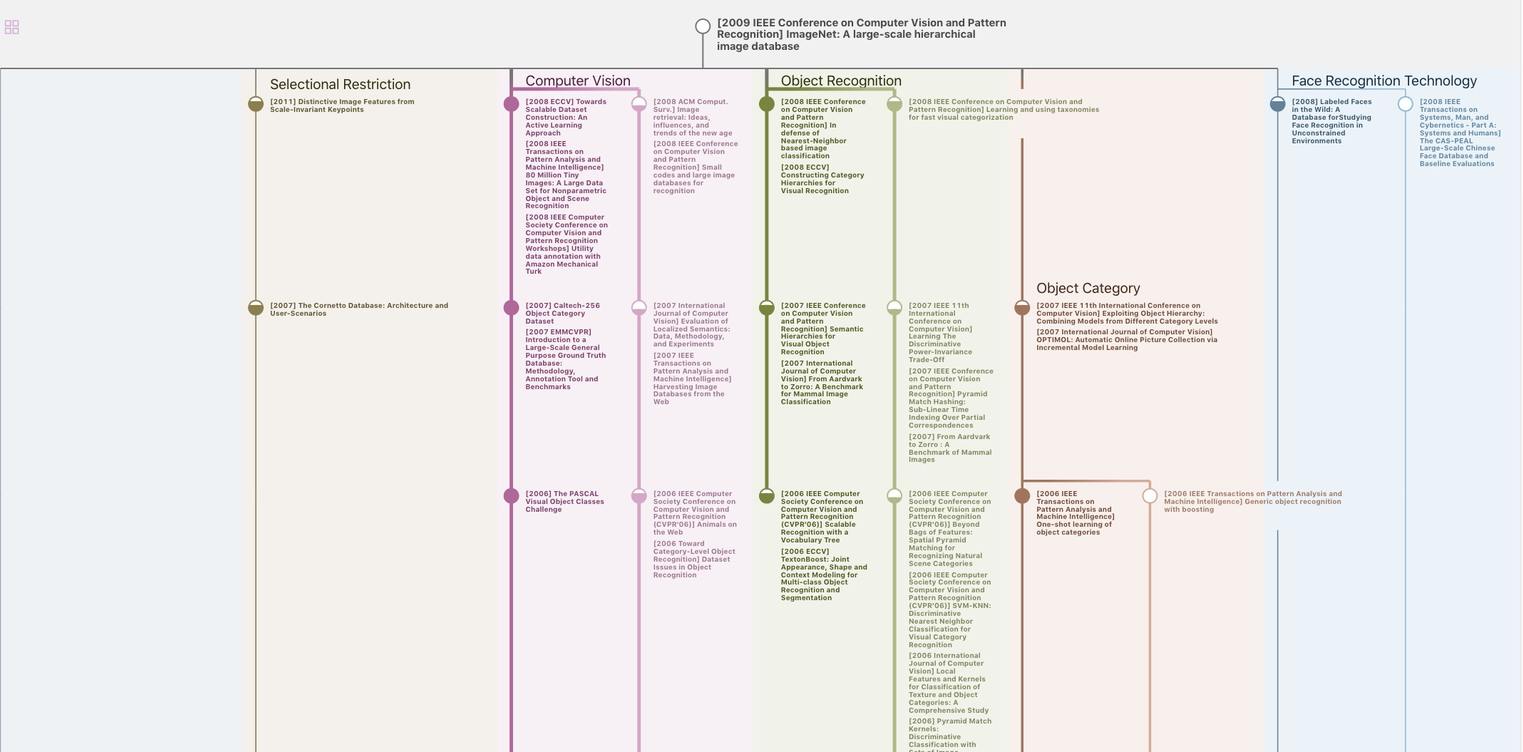
生成溯源树,研究论文发展脉络
Chat Paper
正在生成论文摘要