Multi-feature deep supervised voiceprint adversarial network for depression recognition from speech
BIOMEDICAL SIGNAL PROCESSING AND CONTROL(2024)
摘要
Depression can induce a range of physiological effects, leading to notable distinctions in the acoustic charac-teristics exhibited by individuals with depression as opposed to those without. Designing efficient algorithms to accurately identify depression through speech poses a formidable challenge. In this paper, we propose the Multi-Feature Deep Supervised Voiceprint Adversarial Network (MFDS-VAN) for audio-based depression recognition. The MFDS-VAN assimilates extracted acoustic features and the audio waveform, subsequently generating predictions regarding the depression score. In order to attain more robust and discriminative spatial- temporal features associated with depression, the Encoding Network module merges long-term and short-term acoustic features with the unprocessed audio waveform, while the Regression Network module enables prediction of the depression score. The Deep Supervised Regression algorithm is designed by combining GE2E clustering and Huber regression for better network optimization. Furthermore, to enhance the representation the MFDS-VAN while diminishing the influence of individual voiceprint information, we propose the Voiceprint Adversarial Network. Experimental results conducted on AVEC 2013, AVEC 2014, and AVEC 2017 datasets demonstrate that the MFDS-VAN significantly enhances robustness and performance in speech-based depression recognition. Our model achieves competitive results when compared to recent audio-based methodologies.
更多查看译文
关键词
Adversarial learning,Audio processing,Attention mechanism,Deep neural network,Depression recognition,Feature enhancement
AI 理解论文
溯源树
样例
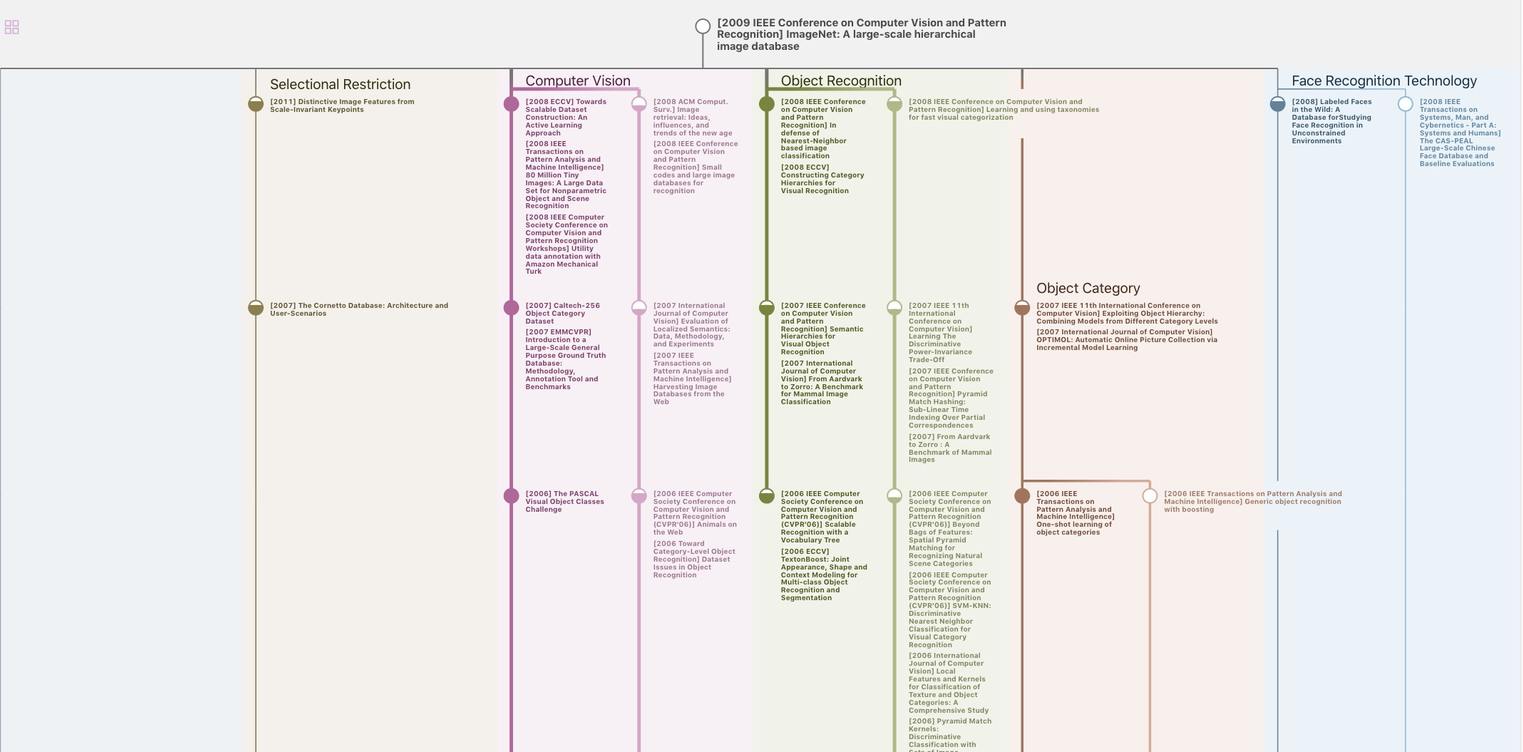
生成溯源树,研究论文发展脉络
Chat Paper
正在生成论文摘要