Low-Redundant Unsupervised Feature Selection based on Data Structure Learning and Feature Orthogonalization
EXPERT SYSTEMS WITH APPLICATIONS(2024)
摘要
An orthogonal representation of features can offer valuable insights into feature selection as it aims to find a representative subset of features in which all features can be accurately reconstructed by a set of features that are linearly independent, uncorrelated, and perpendicular to each other. In this paper, a novel feature selection method, called Low-Redundant Unsupervised Feature Selection based on Data Structure Learning and Feature Orthogonalization (LRDOR), is presented. In the first stage, the suggested LRDOR method makes use of the QR factorization over the whole set of features to find the orthogonal representation of the feature space. Then, LRDOR utilizes the directional distance based on the matrix factorization in order to determine the distance among the set of considered features and the orthogonal set obtained from the original features. Moreover, LRDOR simultaneously takes into account the local correlation of features and the data manifold as dual information into the feature selection process, which can lead to a low level of redundancy and maintain the geometric data structure when reducing the data dimension. In addition to providing a proficient iterative algorithm, the convergence analysis is also included to solve the objective function of LRDOR. The results of the experiments demonstrate that for clustering purposes, LRDOR works better than other related state-of-the-art unsupervised feature selection methods on ten real-world datasets.
更多查看译文
关键词
Feature selection,Orthogonalization,Data manifold,Local correlation,Matrix factorization
AI 理解论文
溯源树
样例
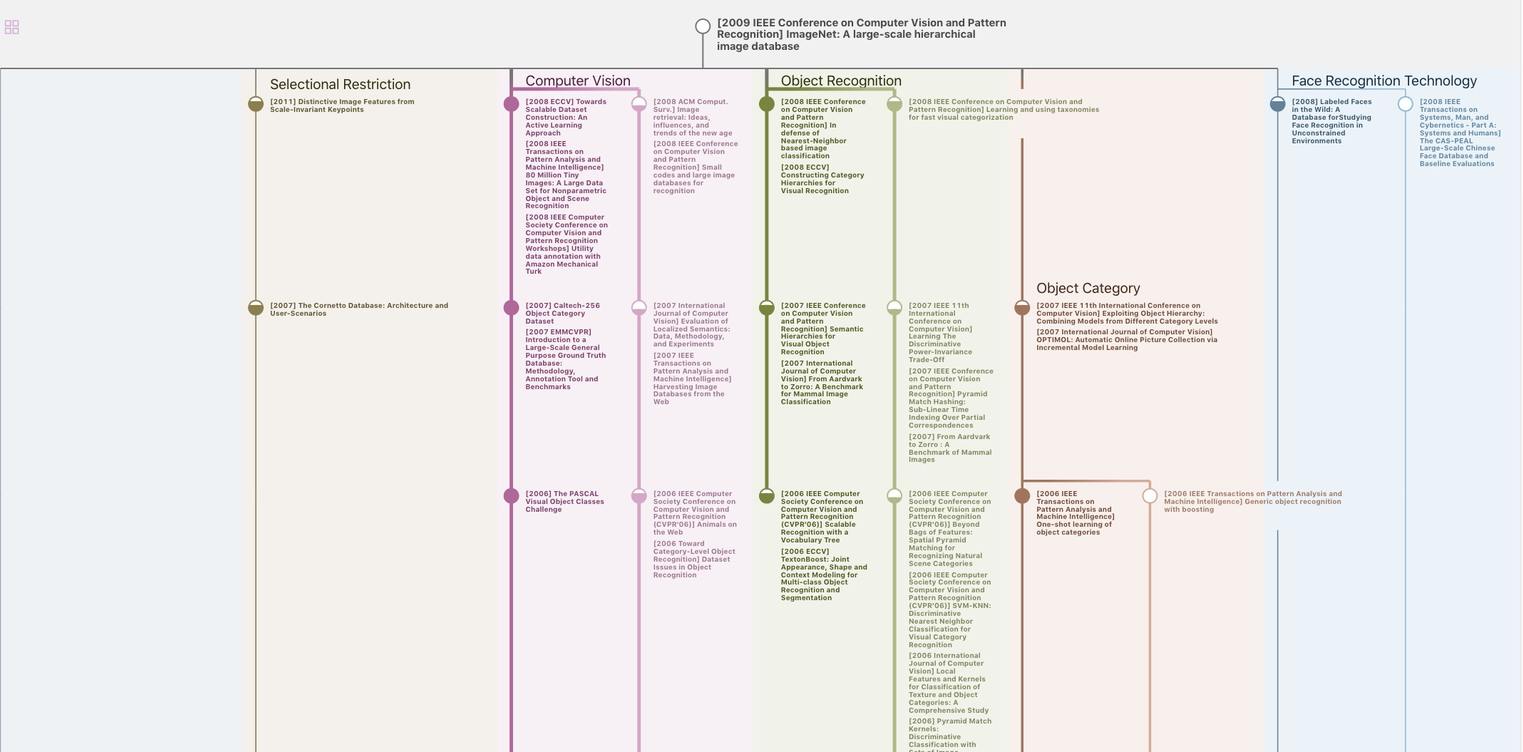
生成溯源树,研究论文发展脉络
Chat Paper
正在生成论文摘要