A knowledge-guided bi-population evolutionary algorithm for energy-efficient scheduling of distributed flexible job shop problem
ENGINEERING APPLICATIONS OF ARTIFICIAL INTELLIGENCE(2024)
摘要
With the guidance of the advanced manufacturing philosophy, green scheduling and energy efficiency have received considerable attention from enterprises and countries. Meanwhile, distributed manufacturing is becoming widespread due to the exploration of the business. Thus, this paper investigates the energy-efficient scheduling of the distributed flexible job shop problem (EEDFJSP) with the goal of minimizing the makespan and total energy consumption (TEC). Considering the difficulty of simultaneously optimizing both objectives, a knowledge-guided bi-population evolutionary algorithm (KBEA) is proposed to address this issue. Firstly, a problem-specific initialization strategy based on a four-vector representation is presented, which corresponds to four sub-problems including factory assignment, operation sequence, machine assignment, and speed assignment. Secondly, five different types of evolutionary operators with adaption strategy is designed to guide the bi-population to complete efficient evolution. Thirdly, a knowledge-guided local search strategy is used to enhance the exploitation capability of the algorithm. Furthermore, an elaborately-designed energy-saving strategy based on knowledge is developed to further reduce energy consumption. Additionally, to verify the effectiveness of the proposed KBEA, extensive experiments are conducted to compare with other 7 comparison algorithms on 39 instances. Experimental results manifest that KBEA is superior to its competitors.
更多查看译文
关键词
Distributed flexible job shop scheduling,Multi-objective optimization problem,Energy-efficient,Bi-population evolutionary algorithm
AI 理解论文
溯源树
样例
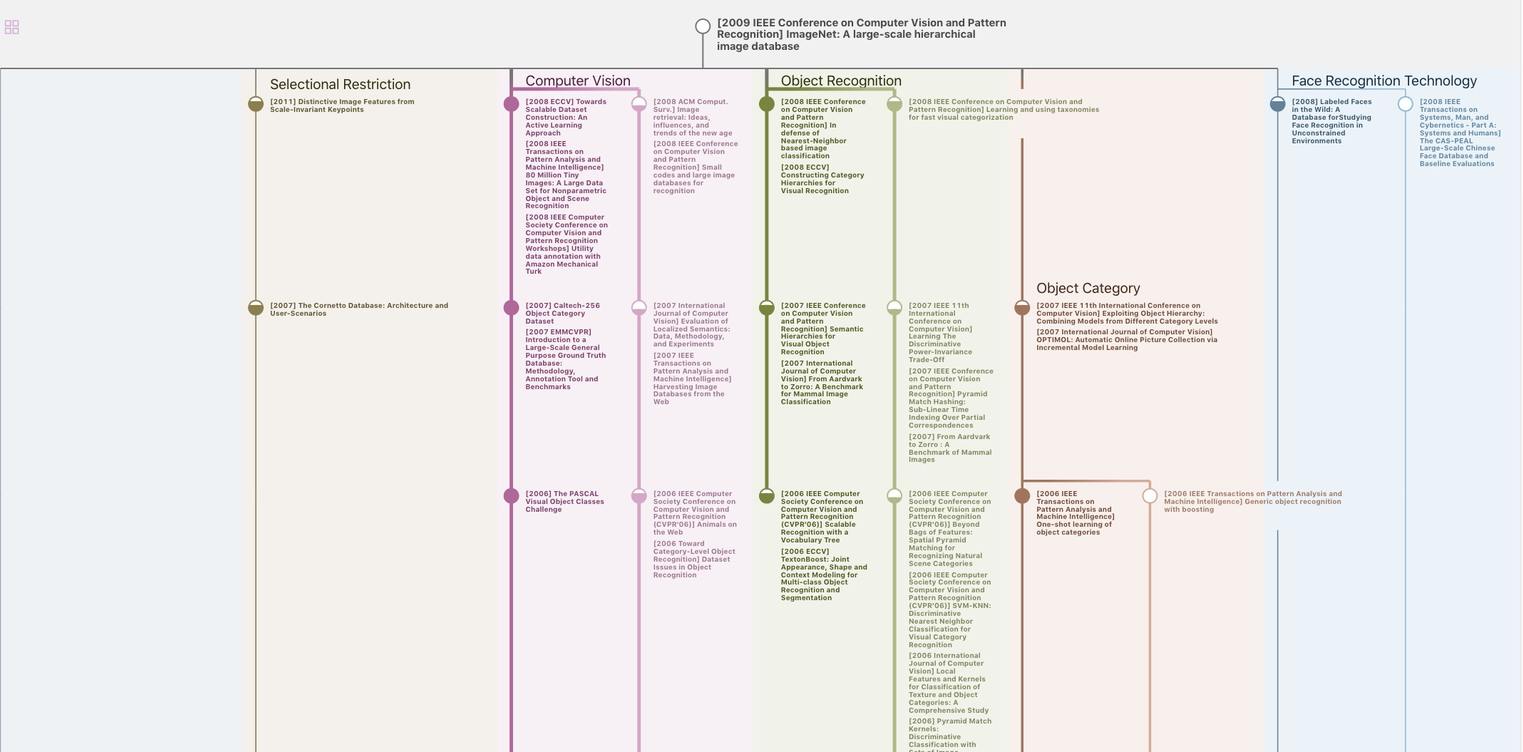
生成溯源树,研究论文发展脉络
Chat Paper
正在生成论文摘要