Spatio-temporal scale information fusion of Functional Near-Infrared Spectroscopy signal for depression detection
KNOWLEDGE-BASED SYSTEMS(2024)
摘要
Depression, a prevalent and debilitating mental health disorder, necessitates early detection to enable effective intervention. Functional Near -Infrared Spectroscopy (fNIRS) has proven to be a promising technique for studying depressive symptoms due to its affordability, portability, high spatial resolution and non-invasiveness. However, existing fNIRS-based depression detection approaches often overlook signal intrinsic properties and intrinsic correlations among different feature types, as well as local spatial geometric structures of data. To overcome these limitations, we suggest a spatio-temporal scale information fusion framework that involves supervised local low -rank correlation (IFLRC) to detect depression. IFLRC incorporates a low -rank constraint, a sparse l(2,1)-norm term, multiple Laplacian regularizations, and a sparse penalty projection matrix to capture global spatial functional information, handle errors to enhance robustness to noise, preserve local spatial geometric structures, exploit correlations among multiple information sets, and enhance discriminative ability. An iterative algorithm, based on the alternating direction method of multipliers (ADMM), has been developed to solve the suggested objective function. The experimental findings validate the efficacy of IFLRC, yielding favorable detection rates of 95.8%, 87.3%, and 97.2% in response to positive, neutral, and negative emotional stimuli, respectively. These findings exceed existing methods, demonstrating the potential of fNIRS and the effectiveness of IFLRC for detecting depression. IFLRC presents notable advancements in feature extraction and fusion techniques for depression detection. Its capacity to capture spatio-temporal scale information, leverage intrinsic correlations, and preserve local geometric structures translates into improved performance. This framework holds promise for early detection and intervention in depressive disorders, offering valuable insights for clinical applications.
更多查看译文
关键词
Information fusion,Alternating optimization,Depression detection,Functional Near-Infrared Spectroscopy (fNIRS)
AI 理解论文
溯源树
样例
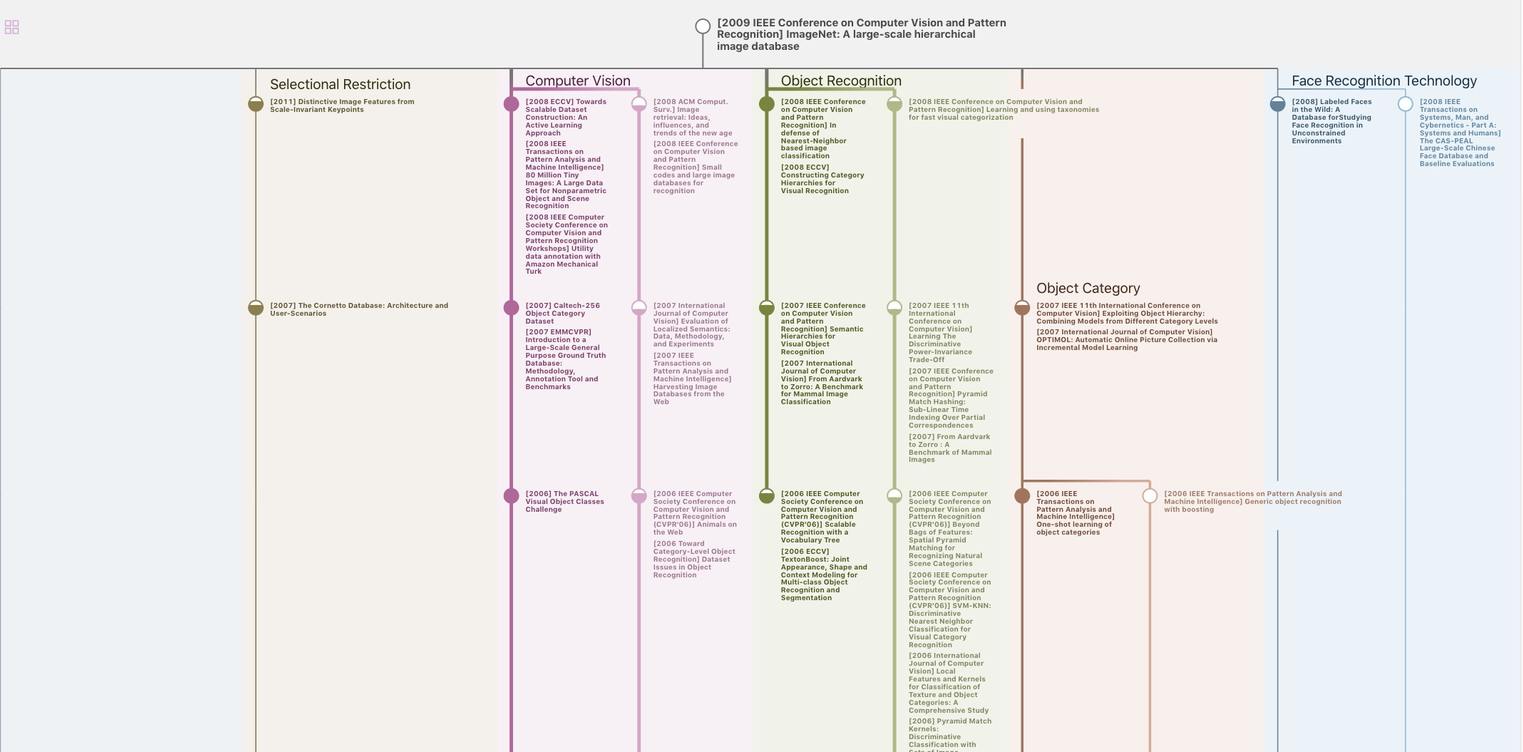
生成溯源树,研究论文发展脉络
Chat Paper
正在生成论文摘要