SRTNet: Scanning, Reading, and Thinking Network for myocardial infarction detection and localization
EXPERT SYSTEMS WITH APPLICATIONS(2024)
摘要
Objective: The incidence of myocardial infarction (MI) is increasing year by year and is characterized by a high mortality rate with rapid outbreaks. Electrocardiogram (ECG)-based detection and localization of myocardial infarction is susceptible to individual patient differences. We propose a new deep learning model to improve the detection and localization performance on different patient data. Methods: To address these issues, we propose SRTNet, a novel model with scanning, reading, and thinking modules, for MI detection and localization. The model learns ECG features from three different perspectives, namely, the scanning module for learning 12-lead ECG features, the reading module for learning the correlation features of different leads, and the thinking module for learning temporal dimension features. The model learned features contain both temporal and spatial ones that can alleviate overfitting individual differences. Results: In the experiments, we used 5-fold cross-validation in the PTB dataset to demonstrate the performance of SRTNet. The results show that SRTNet achieves the state-of-the-art performance. For detection performance, the accuracy is 99.25%, the sensitivity is 99.15%, the specificity is 98.58%, and the F1 score is 99.09%. For localization performance, the accuracy is 68.45%, the sensitivity is 61.82%, the specificity is 67.95% , and the F1 score is 60.50%. Conclusion: The experimental results show that SRTNet's multi-level feature learning method can detect and locate MI more accurately. Significance: Furthermore, the design of SRTNet provided a new perspective on clinical MI detection and localization systems.
更多查看译文
关键词
Myocardial infarction,Transformer,12-lead electrocardiogram,Inter-patient,Convolutional neural network
AI 理解论文
溯源树
样例
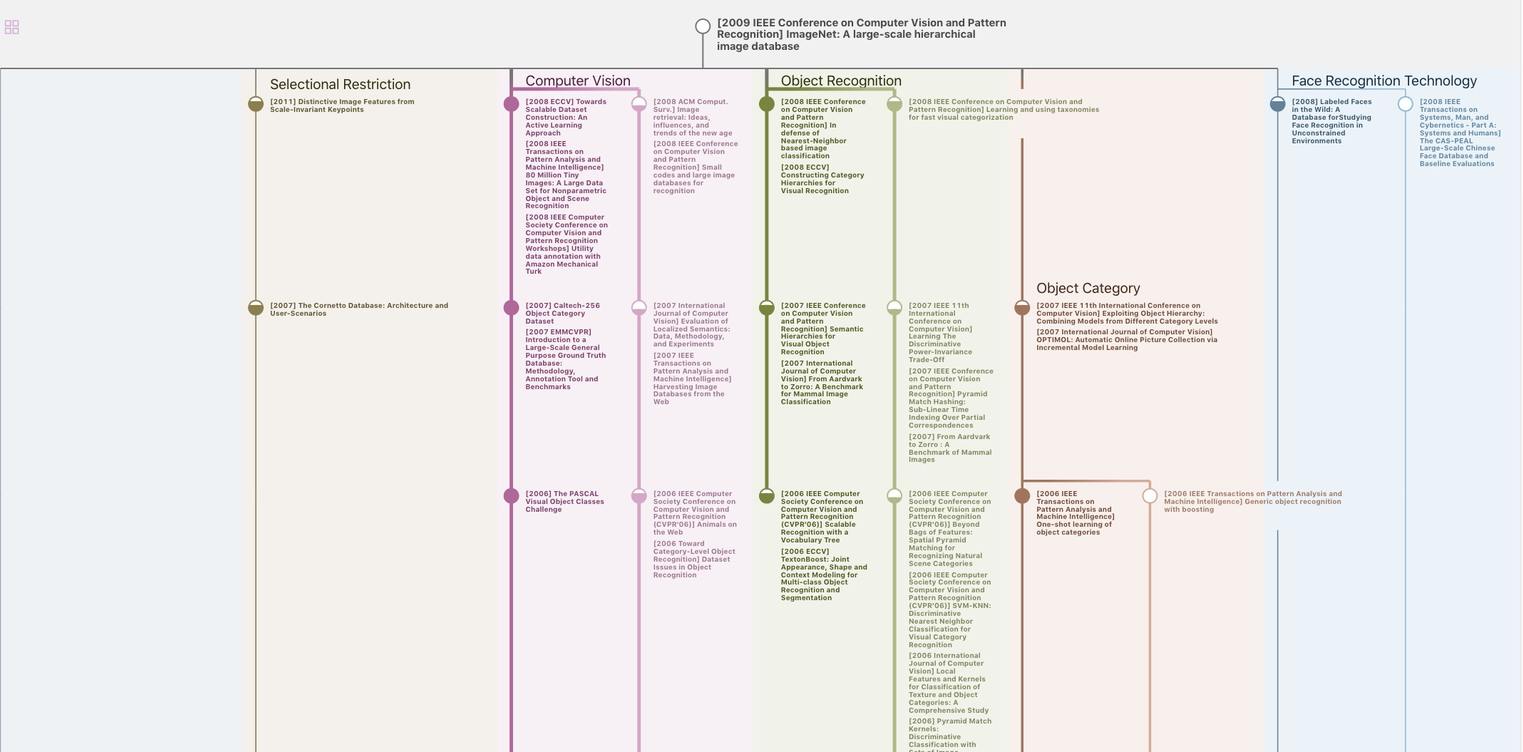
生成溯源树,研究论文发展脉络
Chat Paper
正在生成论文摘要