GAN-powered heterogeneous multi-agent reinforcement learning for UAV-assisted task
AD HOC NETWORKS(2024)
摘要
The flexible and highly mobile unmanned aerial vehicle (UAV) with computing capabilities can improve the quality of experience (QoE) of ground users (GUs) according to real-time service requirements by performing flight maneuvers. In this study, we investigate a task offloading scheme and trajectory optimization in a multi-UAV-assisted system, where UAVs offload a portion of multiple GUs' computational tasks. The optimization problem is formulated to jointly minimize the energy consumption of UAVs and the task latency of GUs by optimizing trajectory, task allocation and offloading proportion. This paper proposes a heterogeneous multi-agent reinforcement learning (MARL)-based approach to solve the issue in high dimensions and limited states, where UAV and GU are treated separately as two different types of agents. Due to the high cost and low sample efficiency of online training of RL algorithms, a generative adversarial network (GAN)-powered auxiliary training mechanism is proposed, which reduces the overhead of interacting with the real world and makes the agent's policy appropriate for real-world execution environment via offline training with generated environment states. Numerical evaluation results demonstrate that the proposed algorithm outperforms other benchmark algorithms in terms of energy consumption and task latency.
更多查看译文
关键词
Unmanned aerial vehicle,Task offloading,Multi-agent reinforcement learning,Heterogeneous agents,Generative adversarial network
AI 理解论文
溯源树
样例
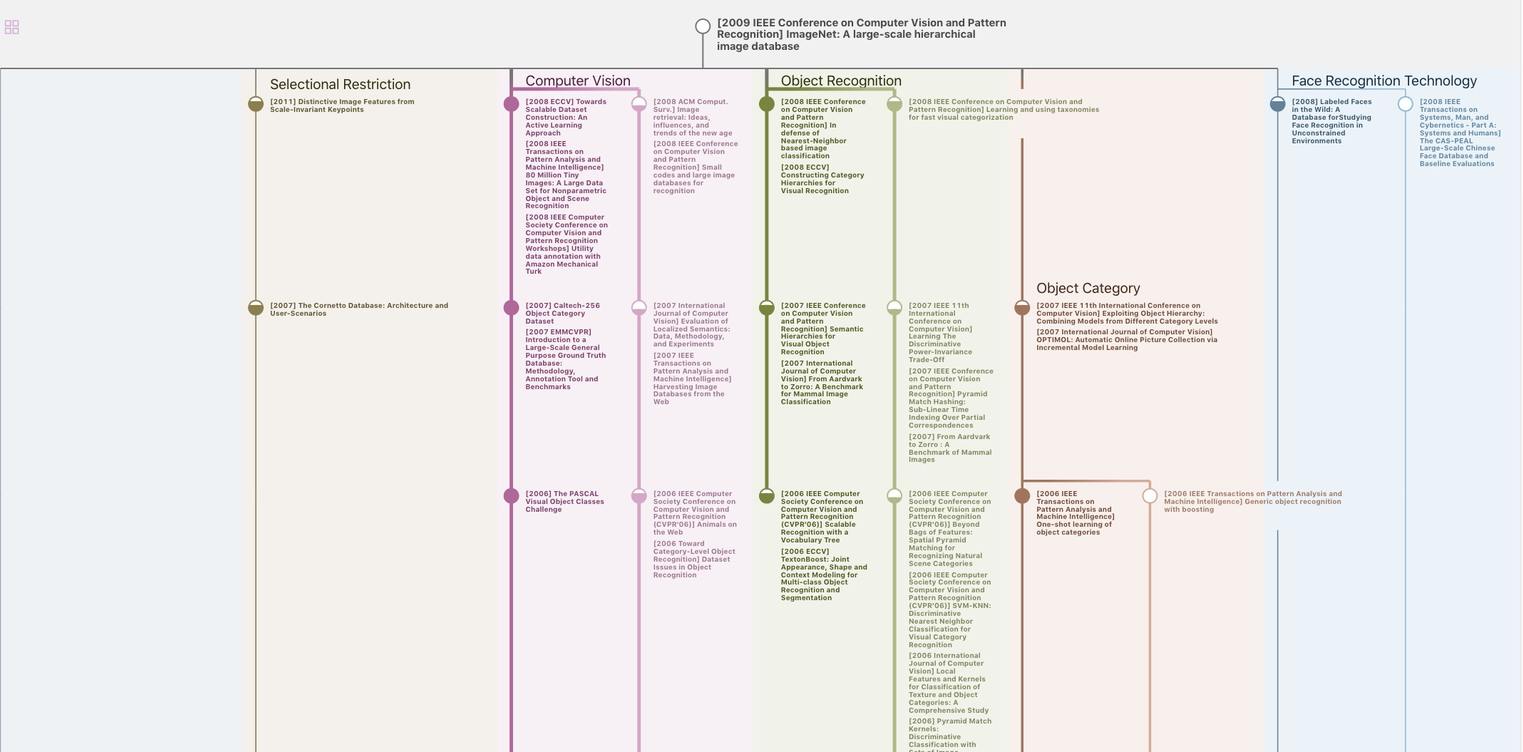
生成溯源树,研究论文发展脉络
Chat Paper
正在生成论文摘要